Elimination of Overfitting of Non-intrusive Load Monitoring Model
2021 IEEE IAS INDUSTRIAL AND COMMERCIAL POWER SYSTEM ASIA (IEEE I&CPS ASIA 2021)(2021)
摘要
The sequence-to-point model has achieved remarkable results in load disaggregation. It relies on a trained deep neural network to identify the power consumption of a single appliance from aggregate load data. However, the model has an over-fitting phenomenon, which makes the loss of the model to the training set small, and it is difficult to obtain a high accuracy rate in the test set. Therefore, it is necessary to use appropriate methods to modify the model to eliminate over-fitting and achieve a higher appliance recognition rate. As a result, the power prediction deviation for a single appliance is relatively large. For example, in the washing machine, the deviation between the predicted value and the ground value can reach more than 90%. So far, there is no documented method to eliminate the over-fitting phenomenon of this model. Therefore, this paper proposes the use of L2 regularization and Dropout to adjust and modify its network. The results show that the increased network architecture and over-fitting elimination methods can improve the decomposition results. The prediction accuracy rate of a single appliance is improved to more than 10%.
更多查看译文
关键词
Non-intrusive load monitoring,sequence-to-point,overfitting,L2 regulation,Dropout
AI 理解论文
溯源树
样例
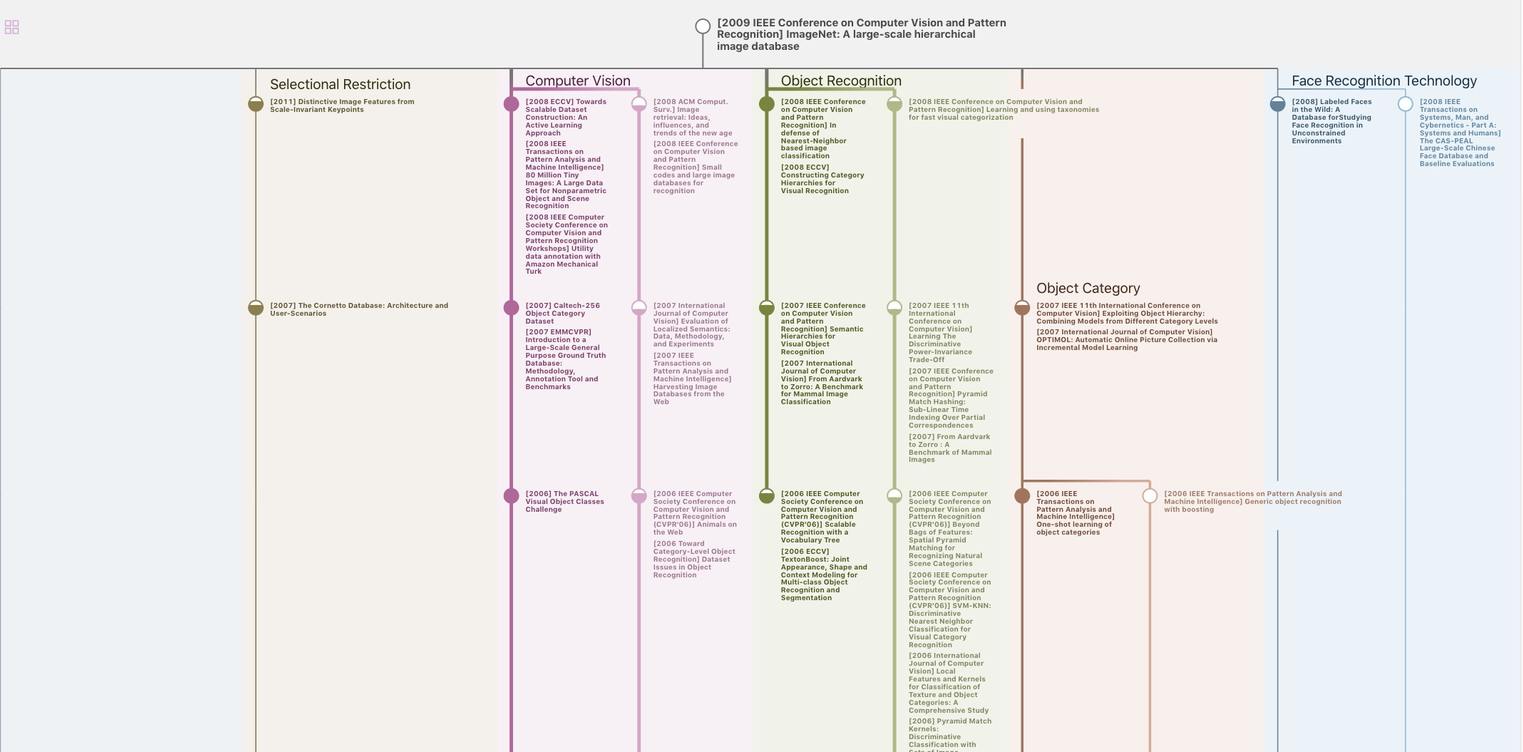
生成溯源树,研究论文发展脉络
Chat Paper
正在生成论文摘要