Meta-Interpretive Learning meets Neural Networks
semanticscholar(2021)
摘要
In this work we present NeuralLog+MIL, a structure learning system based on Meta-Interpretive Learning. NeuralLog+MIL applies the idea of Metagol to learn theories in the NeuralLog language. Metagol is a system that uses a set of higher-order clauses to define a hypotheses space of first-order logic theories and applies those clauses in order to derive first-order theories from examples. Then, NeuralLog transforms a first-order logic program into a neural network. It receives as input a set of first-order clauses that are used to define the neural network models, and a set of facts that becomes weights in the neural network. We show that our approach has competitive results for the link prediction task when compared with Neural-LP in three different datasets. Achieving comparable values for the hit at top 10 entities and the mean rank metric in the UMLS and WordNet datasets; and outperforming Neural-LP in the UWCSE dataset in those metrics.
更多查看译文
AI 理解论文
溯源树
样例
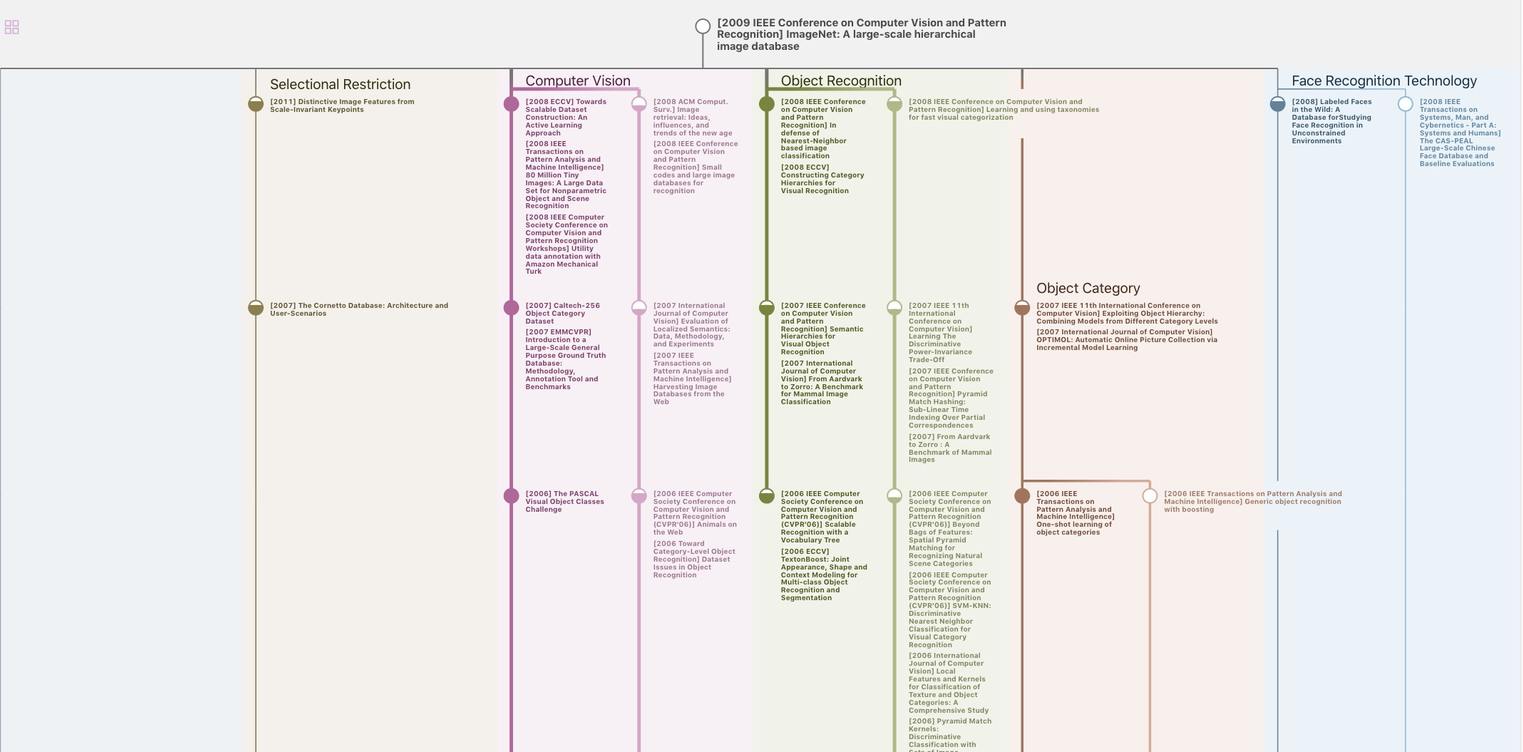
生成溯源树,研究论文发展脉络
Chat Paper
正在生成论文摘要