Collecting and Reporting Race and Ethnicity Data in HCI
EXTENDED ABSTRACTS OF THE 2022 CHI CONFERENCE ON HUMAN FACTORS IN COMPUTING SYSTEMS, CHI 2022(2022)
Univ Washington | Univ Texas Austin | Northeastern Univ
- Pretraining has recently greatly promoted the development of natural language processing (NLP)
- We show that M6 outperforms the baselines in multimodal downstream tasks, and the large M6 with 10 parameters can reach a better performance
- We propose a method called M6 that is able to process information of multiple modalities and perform both single-modal and cross-modal understanding and generation
- The model is scaled to large model with 10 billion parameters with sophisticated deployment, and the 10 -parameter M6-large is the largest pretrained model in Chinese
- Experimental results show that our proposed M6 outperforms the baseline in a number of downstream tasks concerning both single modality and multiple modalities We will continue the pretraining of extremely large models by increasing data to explore the limit of its performance
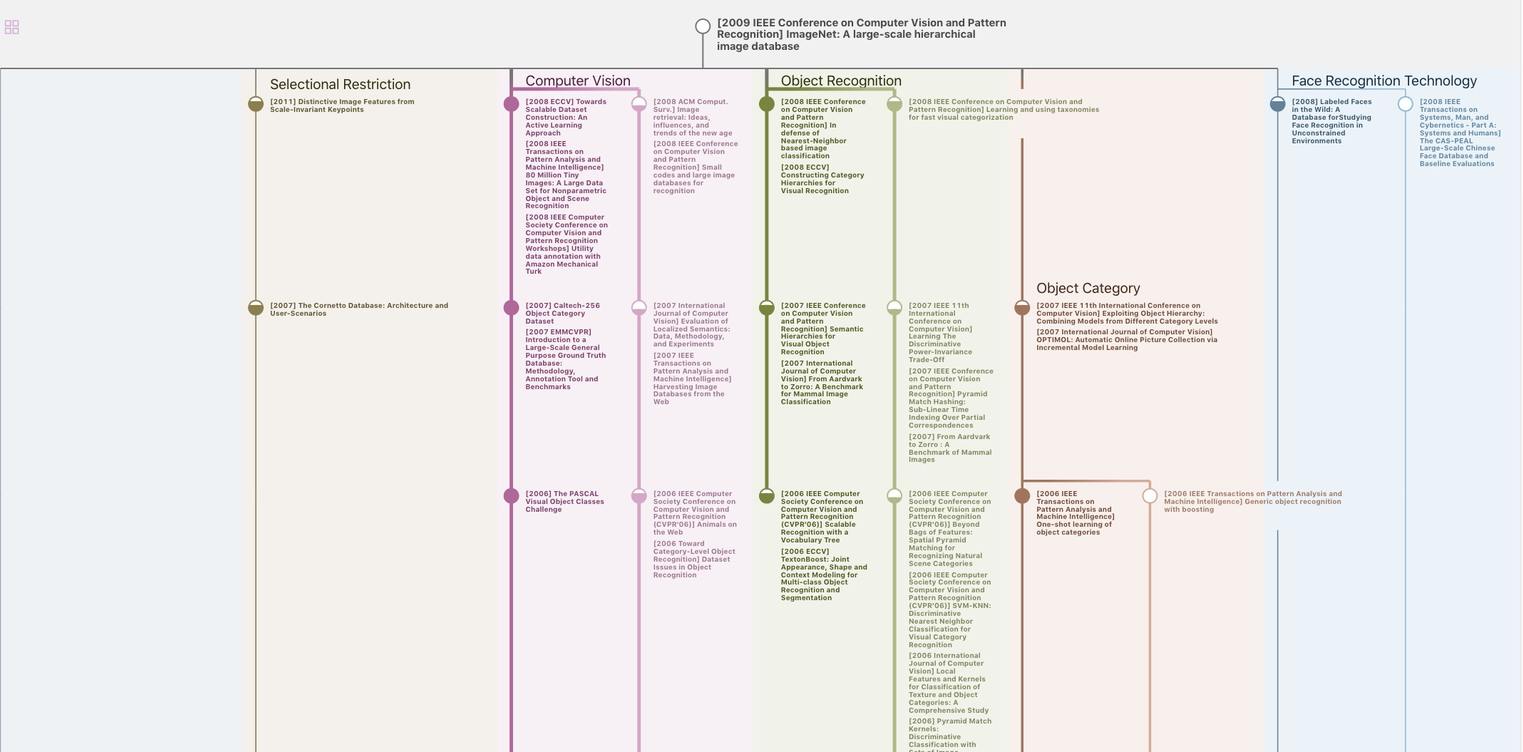
被引用6
Limiting Oxygen Concentration and Supply Rate of Smoldering Propagation
被引用7
A Multidimensional Numeric Study on Smoldering-Driven Pyrolysis of Waste Polypropylene
被引用2
被引用8
Improved Pedestrian Fall Detection Model Based on YOLOv5
被引用6
被引用2
被引用9
Progress Variables to Resolve the Steady State Period in a Batch-Type Fixed Bed Combustor
被引用2
被引用3
被引用14
被引用16
"Picture the Audience...": Exploring Private AR Face Filters for Online Public Speaking.
被引用11
A Practical Type-3 Fuzzy Control for Mobile Robots: Predictive and Boltzmann-based Learning
被引用8
被引用1
Smouldering-to-flaming Transition on Wood Induced by Glowing Char Cracks and Cross Wind
被引用4
Flourishing in the Everyday: Moving Beyond Damage-Centered Design in HCI for BIPOC Communities.
被引用10
Every Little Makes a Mickle: the Role of Extracurricular Activity Event in Leadership Emergence
被引用0
被引用0
Limiting Conditions of Smoldering-to-flaming Transition of Cellulose Powder
被引用4
被引用2
UX Design Curriculum: Intersectionality, Race and Ethnicity in Persona Construction
被引用0
被引用0