DESTRESS: Computation-Optimal and Communication-Efficient Decentralized Nonconvex Finite-Sum Optimization
SIAM JOURNAL ON MATHEMATICS OF DATA SCIENCE(2022)
摘要
Emerging applications in multiagent environments such as internet-of-things, networked sensing, autonomous systems, and federated learning, call for decentralized algorithms for finite-sum op-timizations that are resource efficient in terms of both computation and communication. In this paper, we consider the prototypical setting where the agents work collaboratively to minimize the sum of local loss functions by only communicating with their neighbors over a predetermined network topology. We develop a new algorithm, called DEcentralized STochastic REcurSive gradient meth-odS (DESTRESS) for nonconvex finite-sum optimization, which matches the optimal incremental first-order oracle complexity of centralized algorithms for finding first-order stationary points, while maintaining communication efficiency. Detailed theoretical and numerical comparisons corroborate that the resource efficiencies of DESTRESS improve upon prior decentralized algorithms over a wide range of parameter regimes. DESTRESS leverages several key algorithm design ideas includ-ing stochastic recursive gradient updates with minibatches for local computation, gradient tracking with extra mixing (i.e., multiple gossiping rounds) for periteration communication, together with careful choices of hyperparameters and new analysis frameworks to provably achieve a desirable computation-communication trade-off.
更多查看译文
关键词
decentralized optimization,nonconvex finite-sum optimization,stochastic recursive gradient methods
AI 理解论文
溯源树
样例
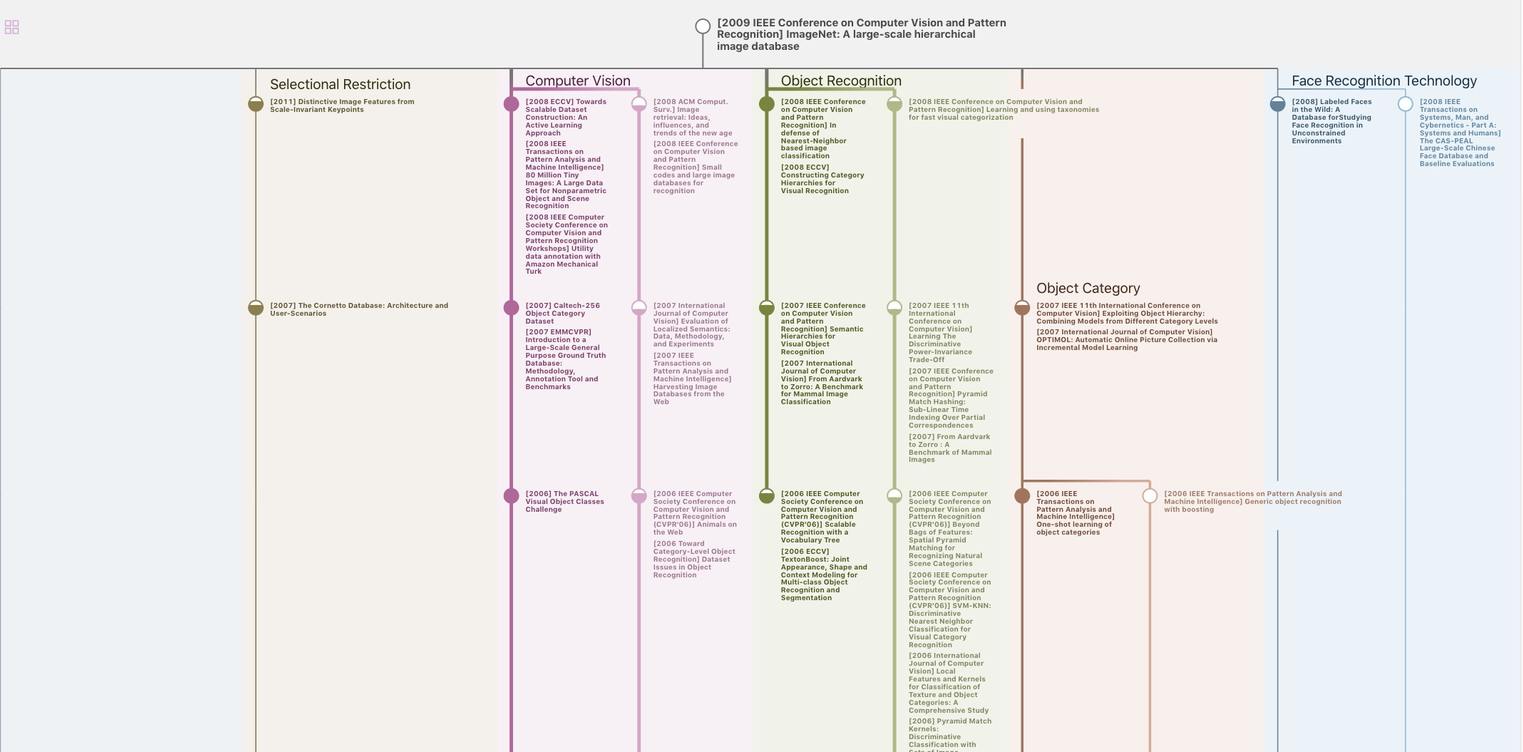
生成溯源树,研究论文发展脉络
Chat Paper
正在生成论文摘要