Liquid Cloud Optical Property Retrieval and Associated Uncertainties Using Multi-Angular and Bispectral Measurements of the Airborne Radiometer OSIRIS
Atmospheric measurement techniques(2023)
摘要
Abstract. In remote sensing applications, clouds are generally characterized by two properties: cloud optical thickness (COT) and effective radius of water/ice particles (Reff). Most of the current operational passive remote sensing algorithms use a mono-angular bi-spectral method to retrieve COT and Reff. They are based on pre-computed lookup tables while assuming a homogeneous plane-parallel cloud layer and without considering measurement errors and the choice of ancillary data. We use the formalism of the optimal estimation method applied to near-infrared multi-angular measurements, to retrieve COT and Reff, and the corresponding uncertainties related to the measurement errors, the ancillary data, and the cloud model assumption. The used measurements were acquired by the airborne radiometer OSIRIS (Observing System Including PolaRization in the Solar Infrared Spectrum), developed by the Laboratoire d'Optique Atmosphérique. It provides multi-angular measurements at tens of meters resolution, very suitable for refining our knowledge of cloud properties and their high spatial variability. OSIRIS is based on the POLDER concept as a prototype of the future 3MI space instrument planned to be launched on the EUMETSAT-ESA MetOp-SG platform in 2023. The used approach allows the exploitation of all the angular information available for each pixel to overcome the radiance angular effects. More consistent cloud properties with lower uncertainty compared to operational mono-directional retrieval methods (MODIS-like methods) are then obtained. The framework of the optimal estimation method provides also the possibility to estimate uncertainties of different sources. Three types of errors were evaluated: (1) Errors related to measurement uncertainties, which reach 10 % for high values of COT and Reff, (2) errors related to an incorrect estimation of the ancillary data that remain below 0.5 %, (3) errors related to the simplified cloud physical model assuming homogeneous plane-parallel cloud and the independent pixel approximation. We show that not considering the in-cloud heterogeneous vertical profiles and the 3D radiative transfer effects lead to uncertainties on COT and Reff exceeding 10 %.
更多查看译文
AI 理解论文
溯源树
样例
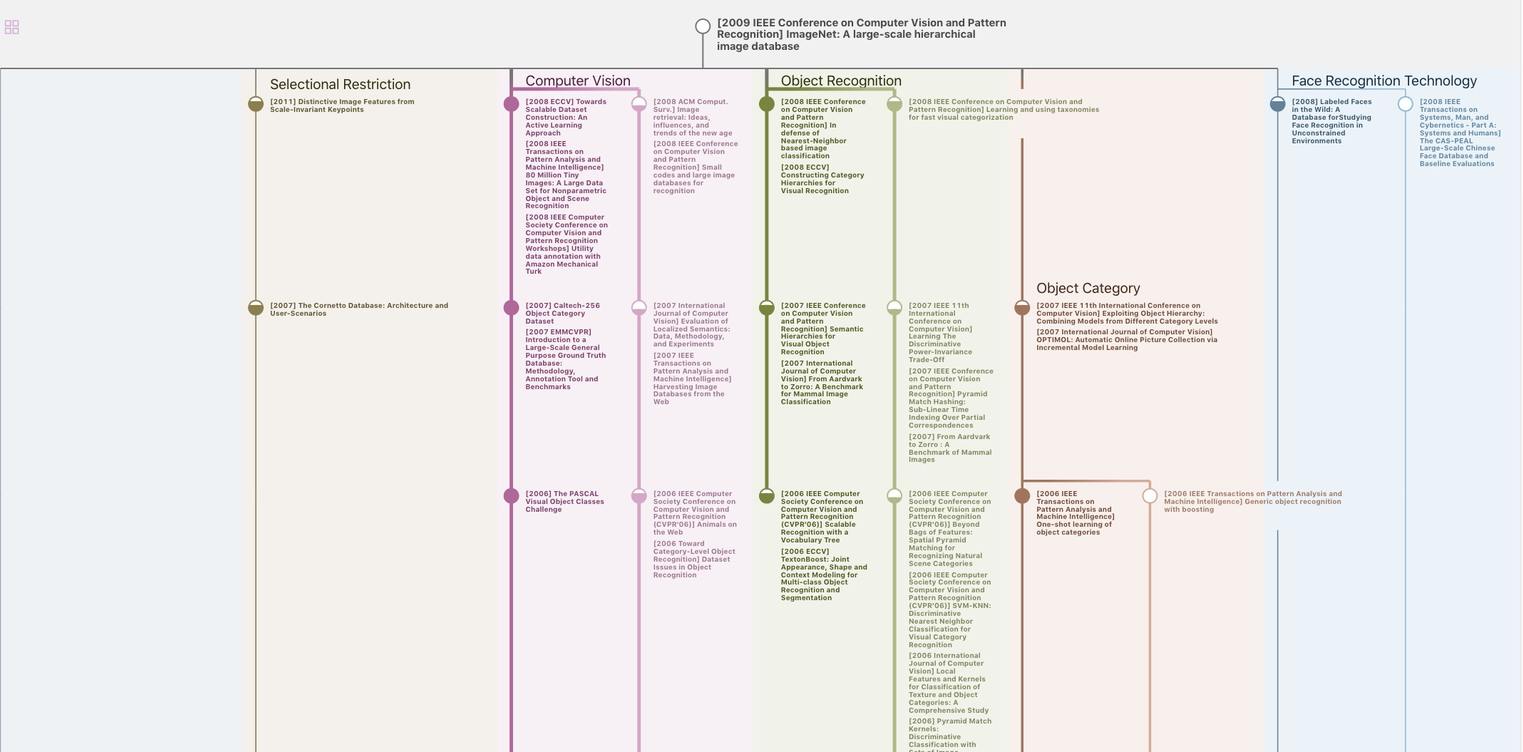
生成溯源树,研究论文发展脉络
Chat Paper
正在生成论文摘要