Moving Objects Tracking Based on Geometric Model-Free Approach with Particle Filter Using Automotive LiDAR
IEEE transactions on intelligent transportation systems(2022)
摘要
In this paper, we propose a Geometric Model-Free Approach with a Particle Filter (GMFA-PF) through the use of automotive LiDAR for real-time tracking of moving objects within an urban driving environment. GMFA-PF proved to be lightweight, capable of finishing the process within the sensing period of the LiDAR on a single CPU. The proposed GMFA-PF tracks and estimates moving objects without any assumptions on the geometry of the target. This approach enables efficient tracking of multiple object classes, with robustness to a sparse point cloud. Point cloud on moving objects is classified via the predicted Static Obstacle Map (STOM). A likelihood field is generated through the classified point cloud and is used in particle filtering to estimate the moving object’s pose, shape, and speed. Quantitative and qualitative comparisons - with Geometric Model-Based Tracking (MBT), Deep Neural Network (DNN), and GMFA - are performed for GMFA-PF using urban driving and scenario driving data gathered on an autonomous vehicle fitted with close-to-market sensors. The proposed approach shows robust tracking and accurate estimation performance in both sparse and dense point clouds; GMFA-PF achieves improved tracking performance in dense traffic and reduces yaw estimation delay compared to the others. Autonomous vehicles with GMFA-PF demonstrated auto-nomous driving on urban roads.
更多查看译文
关键词
Radar tracking,Point cloud compression,Laser radar,Shape,Target tracking,Autonomous vehicles,Vehicle dynamics,Autonomous vehicles,LiDAR,moving object state estimation,particle filter,sparse point cloud
AI 理解论文
溯源树
样例
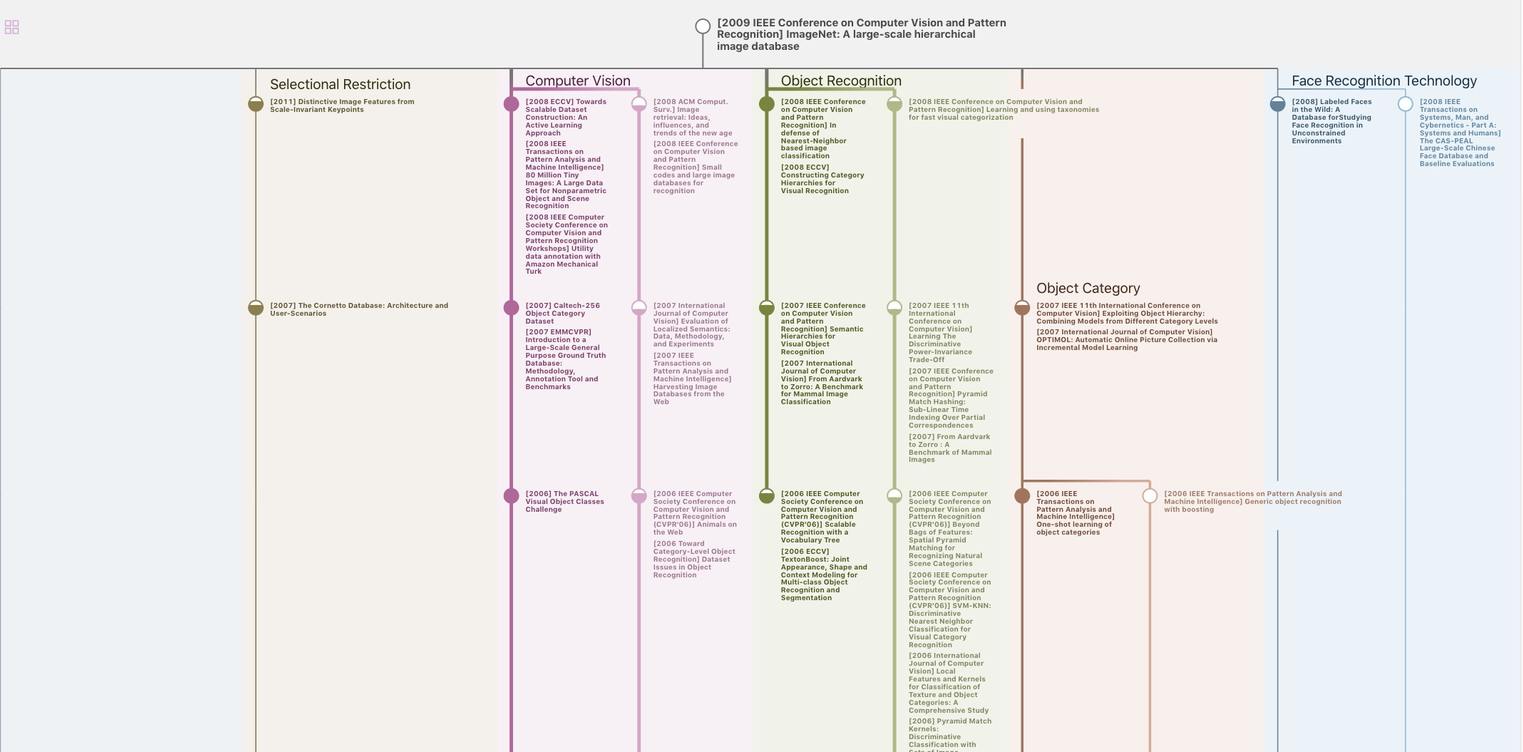
生成溯源树,研究论文发展脉络
Chat Paper
正在生成论文摘要