DynamicDeepFlow: An Approach for Identifying Changes in Network Traffic Flow Using Unsupervised Clustering
MACHINE LEARNING FOR NETWORKING, MLN 2021(2022)
摘要
Understanding flow changes in network traffic has great importance in designing and building robust networking infrastructure. Recent efforts from industry and academia have led to the development of monitoring tools that are capable of collecting real-time flow data, predicting future traffic patterns, and mirroring packet headers. These monitoring tools, however, require offline analysis of the data to understand the big versus small flows and recognize congestion hot spots in the network, which is still an unfilled gap in research. In this study, we proposed an innovative unsupervised clustering approach, DynamicDeep-Flow, for network traffic pattern clustering. The DynamicDeepFlow can recognize unseen network traffic patterns based on the analysis of the rapid flow changes from the historical data. The proposed method consists of a deep learning model, variational autoencoder, and a shallow learning model, k-means++. The variational autoencoder is used to compress and extract the most useful features from the flow inputs. The compressed and extracted features then serve as input-output pairs to k-means++. The k-means++ explores the structure hidden in these features and then uses them to cluster the network traffic patterns. To the best of our knowledge, this is one of the first attempts to apply a real-time network clustering approach to monitor network operations. The real-world network flow data from Energy Sciences Network (a network serving the U.S. Department of Energy to support U.S. scientific research) was utilized to verify the performance of the proposed approach in network traffic pattern clustering. The verification results show that the proposed method is able to distinguish anomalous network traffic patterns from normal patterns, and thereby trigger an anomaly flag.
更多查看译文
关键词
Network traffic pattern clustering,Unsupervised learning,Flow recognition,Wide area network
AI 理解论文
溯源树
样例
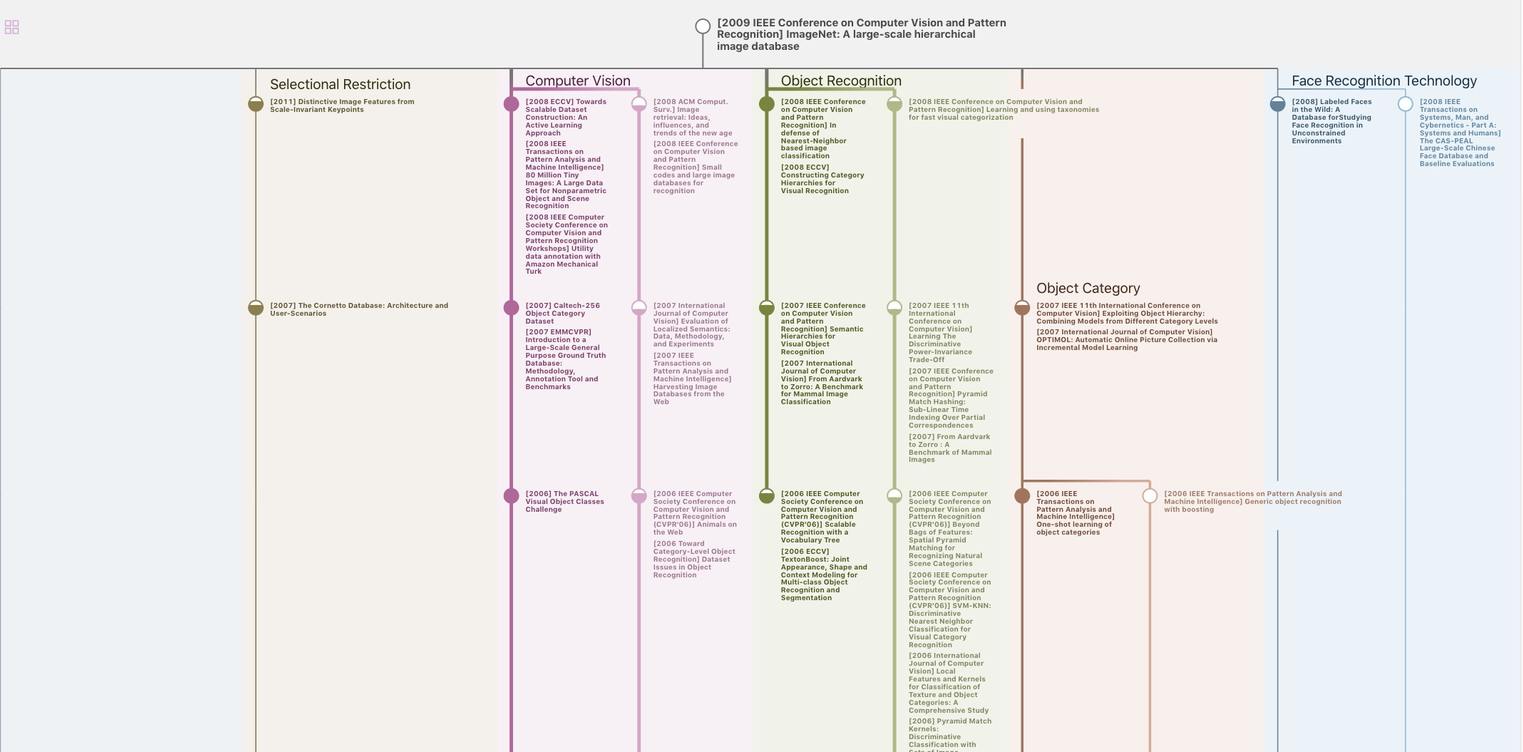
生成溯源树,研究论文发展脉络
Chat Paper
正在生成论文摘要