Targeted-BEHRT: Deep Learning for Observational Causal Inference on Longitudinal Electronic Health Records.
IEEE transactions on neural networks and learning systems(2024)
摘要
Observational causal inference is useful for decision-making in medicine when randomized clinical trials (RCTs) are infeasible or nongeneralizable. However, traditional approaches do not always deliver unconfounded causal conclusions in practice. The rise of "doubly robust" nonparametric tools coupled with the growth of deep learning for capturing rich representations of multimodal data offers a unique opportunity to develop and test such models for causal inference on comprehensive electronic health records (EHRs). In this article, we investigate causal modeling of an RCT-established causal association: the effect of classes of antihypertensive on incident cancer risk. We develop a transformer-based model, targeted bidirectional EHR transformer (T-BEHRT) coupled with doubly robust estimation to estimate average risk ratio (RR). We compare our model to benchmark statistical and deep learning models for causal inference in multiple experiments on semi-synthetic derivations of our dataset with various types and intensities of confounding. In order to further test the reliability of our approach, we test our model on situations of limited data. We find that our model provides more accurate estimates of relative risk [least sum absolute error (SAE) from ground truth] compared with benchmark estimations. Finally, our model provides an estimate of class-wise antihypertensive effect on cancer risk that is consistent with results derived from RCTs.
更多查看译文
关键词
Causal inference,deep learning,electronic health records (EHRs),machine learning
AI 理解论文
溯源树
样例
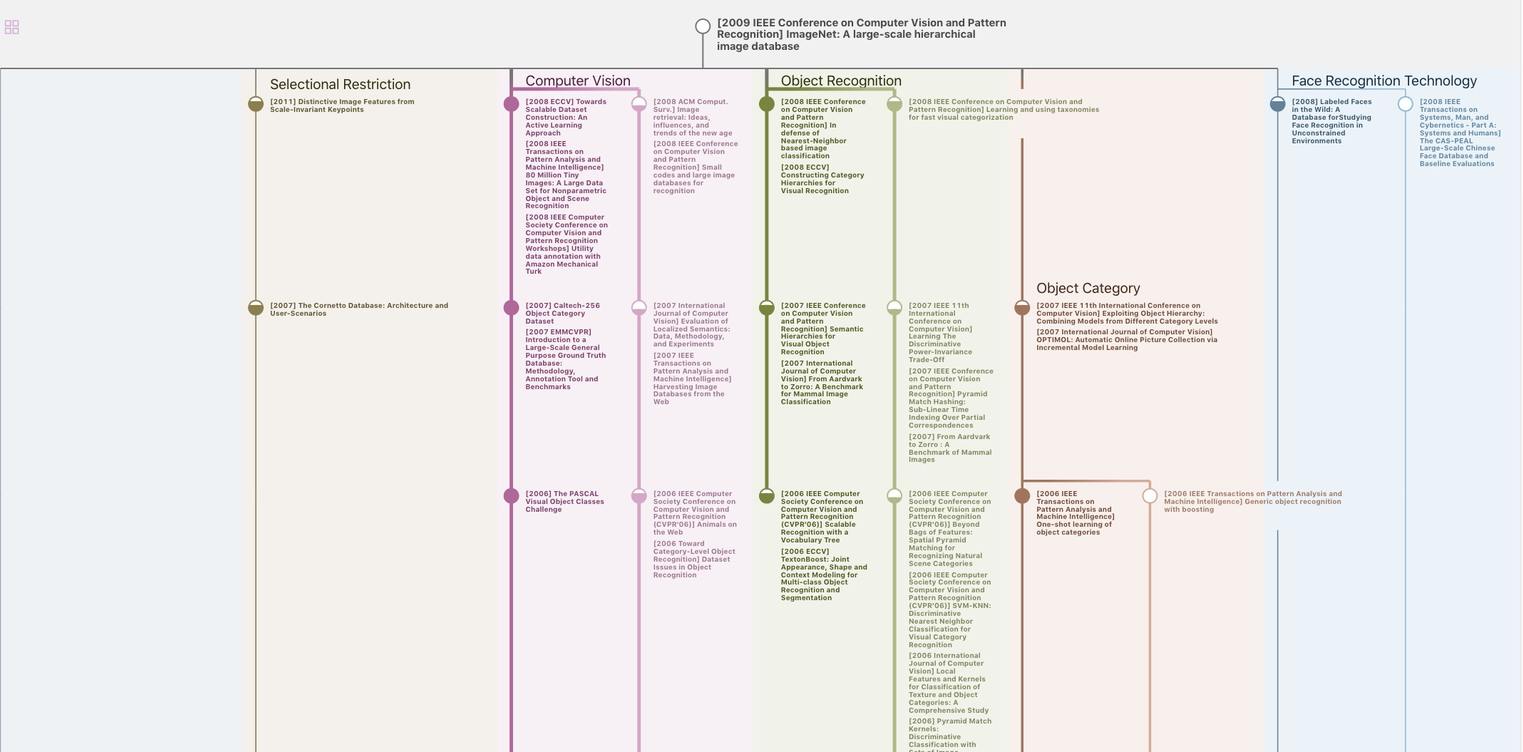
生成溯源树,研究论文发展脉络
Chat Paper
正在生成论文摘要