Conceptual Edits as Counterfactual Explanations.
AAAI Spring Symposia(2022)
摘要
We propose a framework for generating counterfactual explanations of black-box classifiers, which answer the question “What has to change for this to be classified as X instead of Y?” in terms of given domain knowledge. Specifically, we identify minimal and meaningful “concept edits” which, when applied, change the prediction of a black-box classifier to a desired class. Furthermore, by accumulating multiple counterfactual explanations from interesting regions of a dataset, we propose a method to estimate a "global" counterfactual explanation for that region and a desired target class. We implement algorithms and show results from preliminary experiments employing CLEVR-Hans3 and COCO as datasets. The resulting explanations were useful, and even managed to unintendedly reveal a bias in the classifier’s training set, which was unknown to us.
更多查看译文
AI 理解论文
溯源树
样例
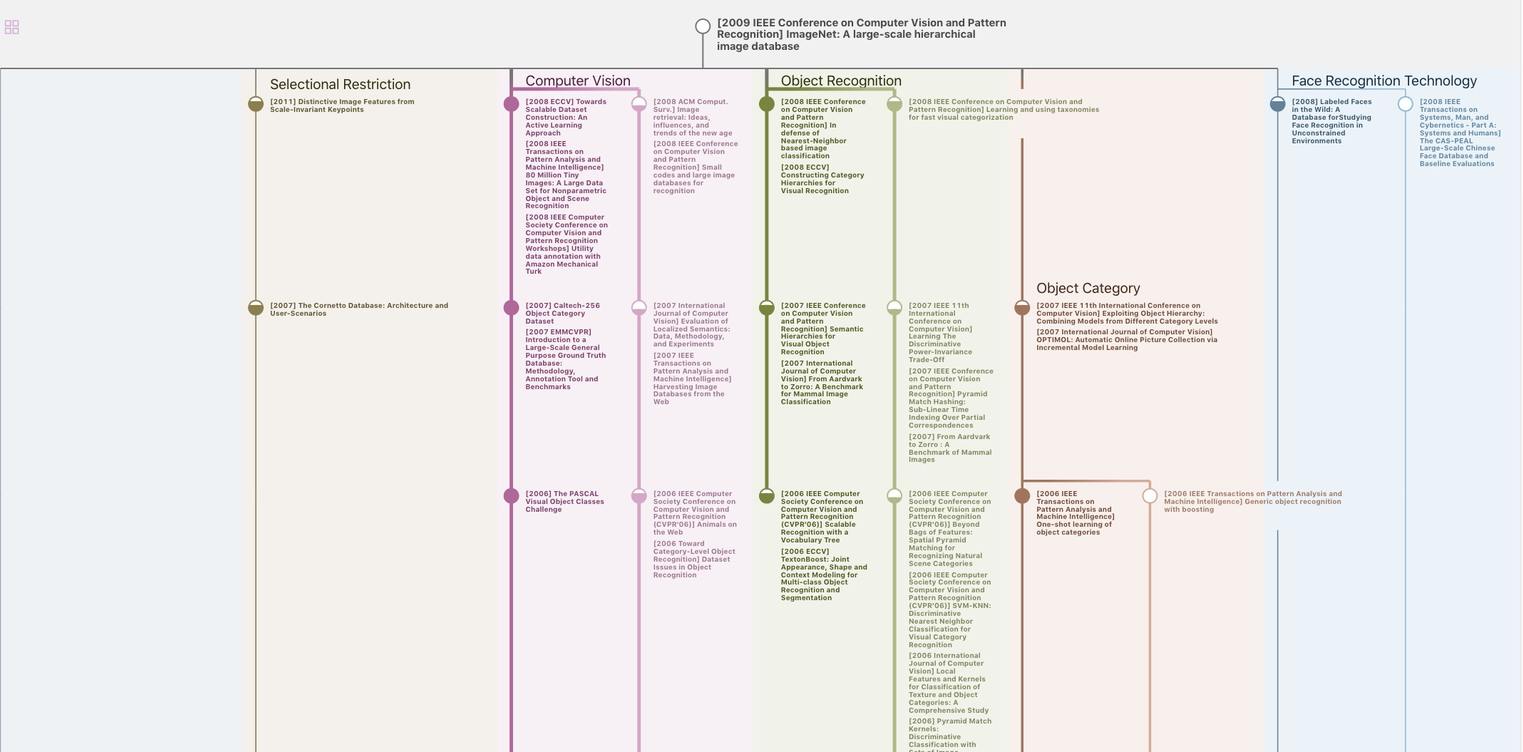
生成溯源树,研究论文发展脉络
Chat Paper
正在生成论文摘要