Visual Semantic Planning for Service Robot Via Natural Language Instructions
2021 China Automation Congress (CAC)(2021)
摘要
The interactive instruction following task requires intelligent robots to complete natural language instructions by interacting with the surrounding environment through visual perception and mechanical manipulation. The most representative benchmark is the ALFRED challenge task, which aims to perform restricted sequential actions to accomplish daily housework in a virtual environment. However, recently proposed multi-modal task planning approaches that combine vision and language do not perform well on ALFRED task. In this work, we utilize natural language instructions to build a single-mode model, and translate the task into a sequential decision problem, which focuses on generating continuous high-level action sequences directly from the instructions. We demonstrate that our K-PLM (knowledge enabled pre-trained language model) may successfully generate concrete visual semantic plans in 31.4% tasks on unseen scenarios without visual cues, where 62.2% can be generated if the model merges some visual cues, i.e. the location of the first object in the scene. The results show that our model provides outstanding visual semantic plans for the embodied agent to perform tasks and outperforms prior works.
更多查看译文
关键词
natural language instructions,knowledge graph,pre-trained model,visual semantic planning,interactive instruction following task
AI 理解论文
溯源树
样例
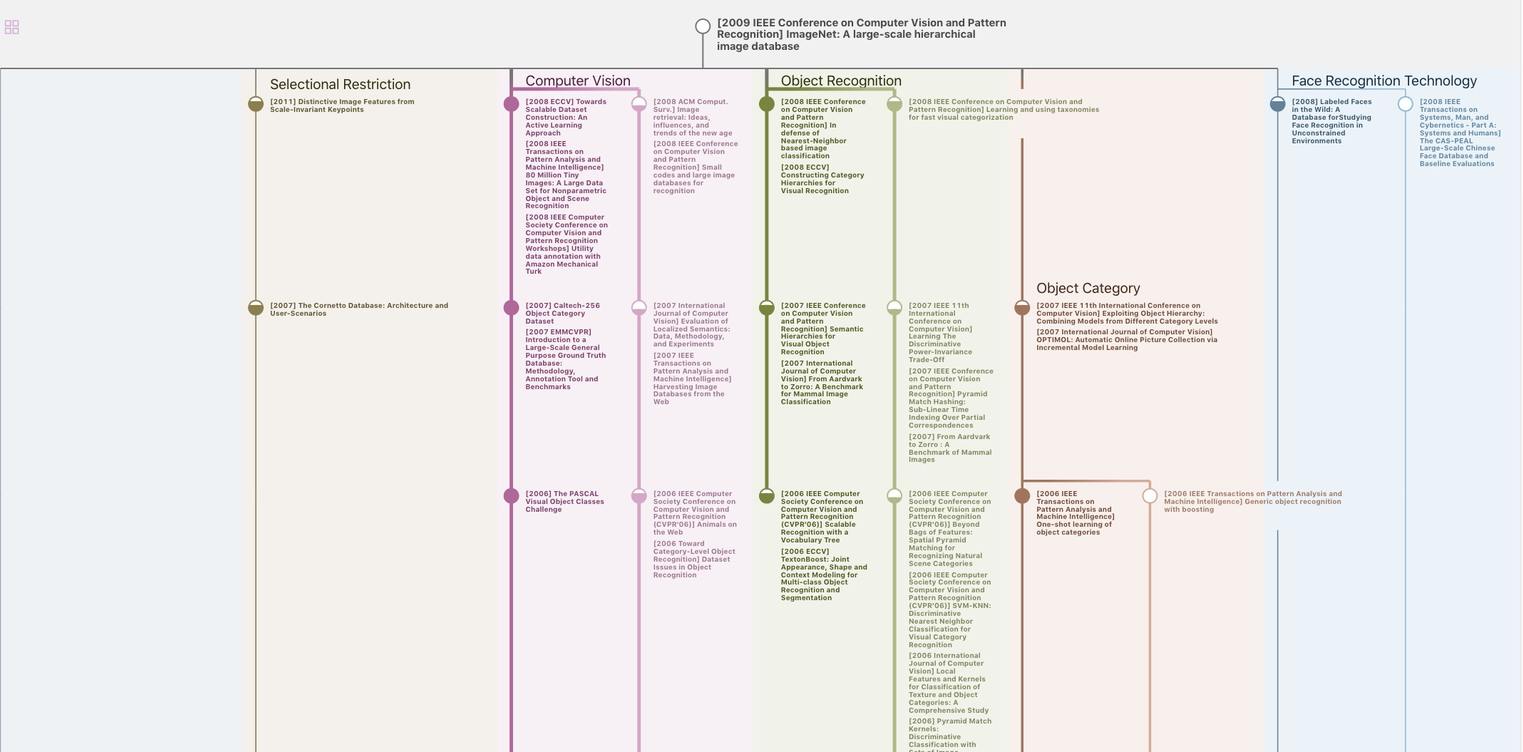
生成溯源树,研究论文发展脉络
Chat Paper
正在生成论文摘要