Adjustable Piecewise Regression Strategy Based Wind Turbine Power Forecasting for Probabilistic Condition Monitoring
Sustainable energy technologies and assessments(2022)
摘要
Due to the frequently changing environmental factors, the wind turbine power generation process shows strong nonlinearity and indeterminacy. How to accurately predict the output power and then to quantitatively monitor the indeterminate condition is thus a great challenge. In this paper, an adjustable piecewise multivariable regression model is proposed to predict the power, and then a probabilistic monitoring strategy is developed to monitor the wind turbine. Firstly, an index is designed to evaluate the distribution characteristics of wind speed and then separate the process into different bins to reduce the nonlinear effect. Then a nonlinear identification clustering algorithm is developed to cluster the bins, which can further identify the nonlinearity among different bins and make the nonlinear relationship stays similar in each cluster. Then, by performing Gaussian process regression on each cluster, the proposed regression model is constructed, which can accurately predict the output power of this process. At last, a probabilistic monitoring strategy is proposed. With this monitoring strategy, each prediction of the proposed regression model can provide the distribution information for the corresponding observation, which can softly evaluate the uncertainty of condition. Four real data sets are used in the experiment. The results illustrate that the proposed regression model can accurately predict the output power, and the monitoring strategy can detect the abnormal conditions for wind turbines with the 95.35% detection accuracy.
更多查看译文
关键词
Nonlinearity,Adjustable piecewise multivariable regression,Nonlinear identification clustering,Bins,Gaussian process regression
AI 理解论文
溯源树
样例
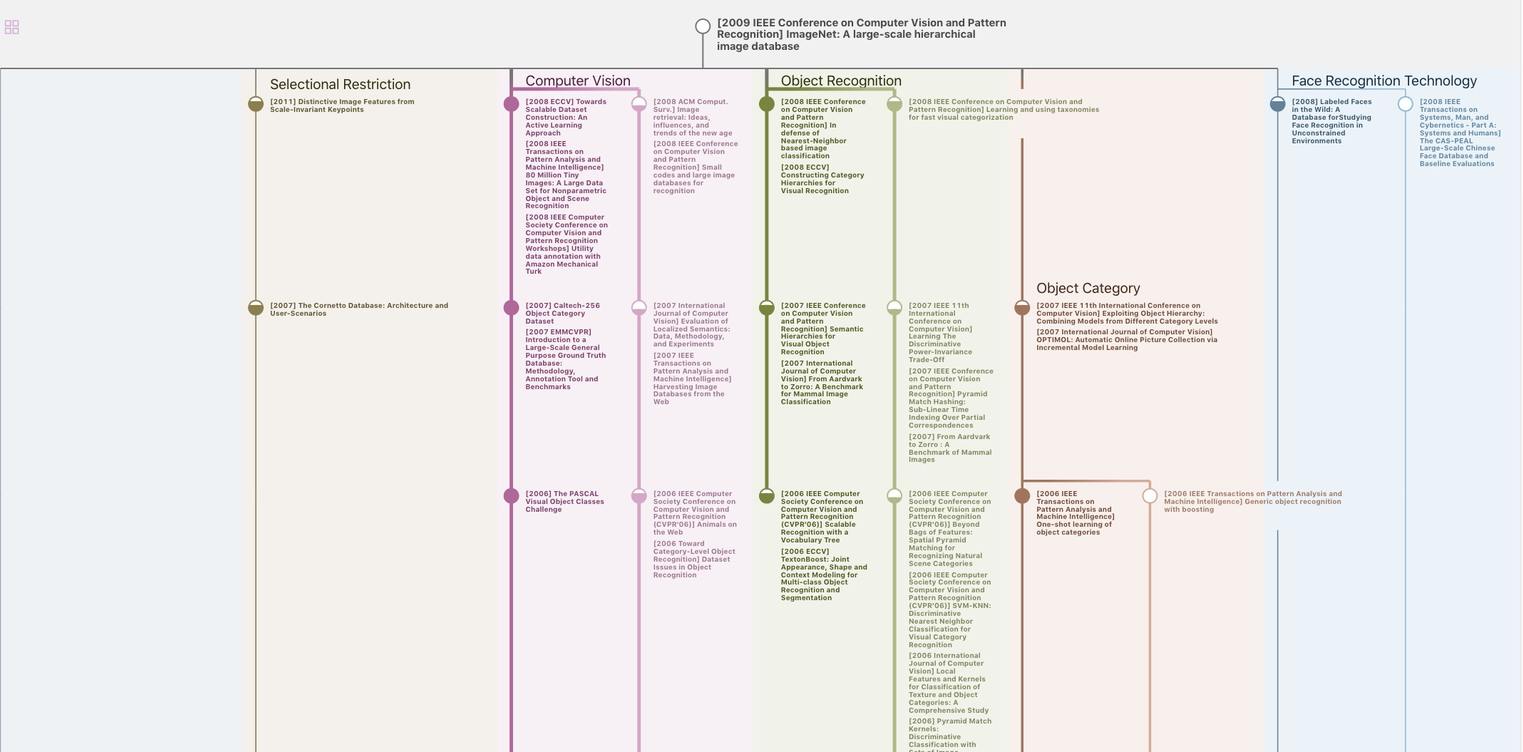
生成溯源树,研究论文发展脉络
Chat Paper
正在生成论文摘要