Machine Learning-Based Operational State Recognition and Compressive Property Prediction in Fused Filament Fabrication
3D PRINTING AND ADDITIVE MANUFACTURING(2023)
摘要
3D printing has exhibited significant potential in outer space and medical implants. To use this technology in the specific high-value scenarios, 3D-printed parts need to satisfy quality-related requirements. In this article, the influence of the filament feeder operating states of 3D printer on the compressive properties of 3D-printed parts is studied in the fused filament fabrication process. A machine learning approach, back-propagation neural network with a genetic algorithm (GA-BPNN) optimized by k-fold cross-validation, is proposed to monitor the operating states and predict the compressive properties. Vibration and current sensors are used in situ to monitor the operating states of the filament feeder, and a set of features are extracted and selected from raw sensor data in time and frequency domains. Results show that the operating states of the filament feeder significantly affected the compressive properties of the fabricated samples, the operating states were accurately recognized with 96.3% rate, and compressive properties were successfully predicted by the GA-BPNN. This proposed method has the potential for use in industrial applications after 3D printing without requiring any further quality control.
更多查看译文
关键词
3D Printing,Fused Deposition Modeling,Metal 3D Printing
AI 理解论文
溯源树
样例
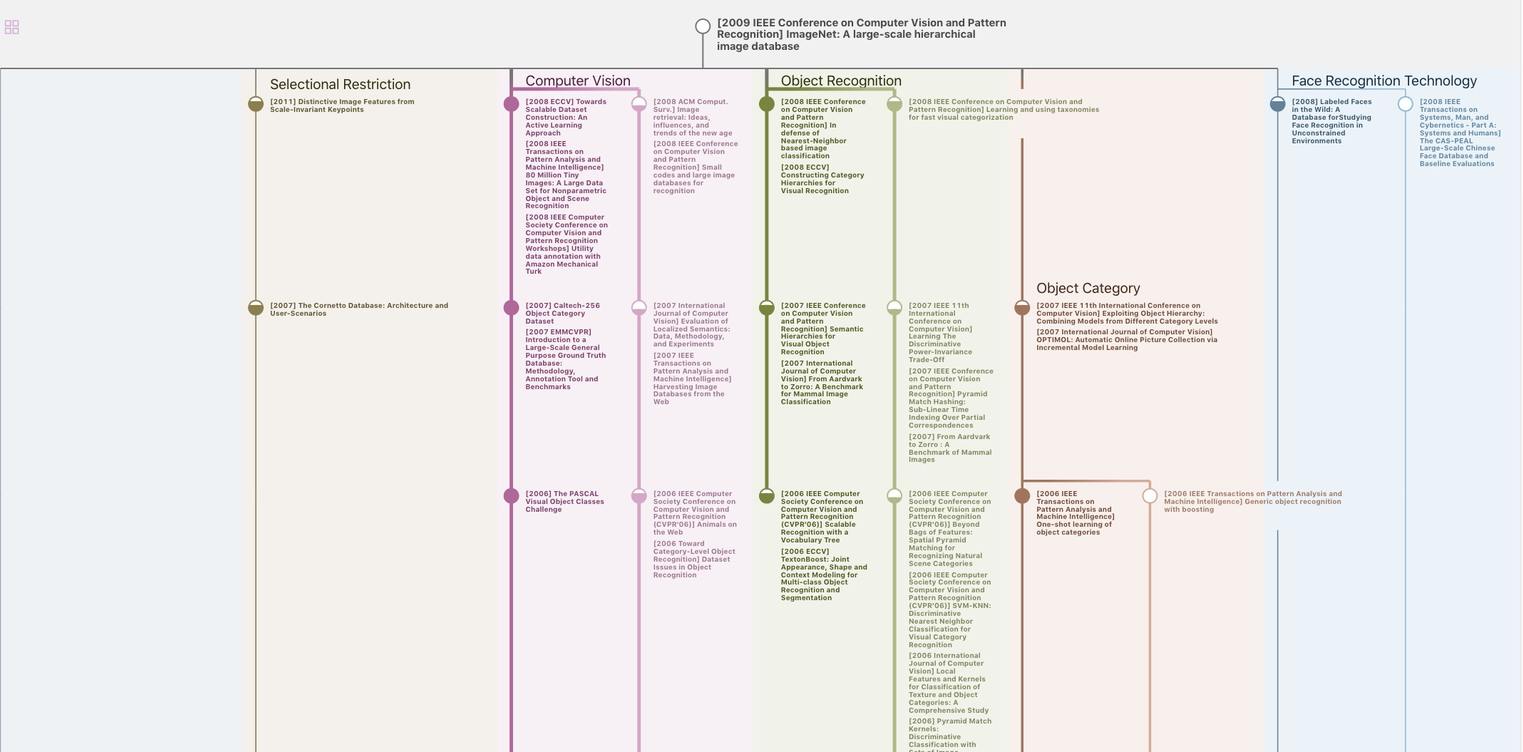
生成溯源树,研究论文发展脉络
Chat Paper
正在生成论文摘要