Forecasting Water Levels in Krasnodar Krai Rivers with the Use of Machine Learning
Water resources(2022)
摘要
The potential of construction of machine learning models was considered as applied to water level forecasting in mountain river reaches in Krasnodar Krai based on observation data on water levels at automated hydrological complexes of the Automated System of Flood Conditions Monitoring in Krasnodar Krai. The study objects were two mountain rivers in Krasnodar Krai―the Pshish and Mzymta. These rivers flow in different natural conditions and differ in their water regimes and the character of lateral inflow in the reaches under consideration. The study focused on three widely used machine learning architectures: regression model of decision trees M5P, gradient boosting of decision trees XGBoost, and artificial neural network based on multilayer perceptron. The forecast quality was evaluated for lead time from 1 to 20 h; variations for rivers with different water regimes and the potential of the examined models as applied to operational forecasting are discussed. The optimal lead time for the Pshish river was found to be 15–18 h (with S/σΔ varying within 0.38–0.39 for XGBoost model); the simulation quality for the Mzymta river is evaluated as good; however, the necessary forecast efficiency is not attained (at the lead time of 5 h, we have S/σΔ = 0.87 for MLP model). The obtained results allow the machine learning models to be regarded as acceptable for short-term hydrological forecasting based on high-frequency water level observation data.
更多查看译文
关键词
hydrological forecasts,machine learning,artificial neural networks,flash floods,short-term water level forecasts
AI 理解论文
溯源树
样例
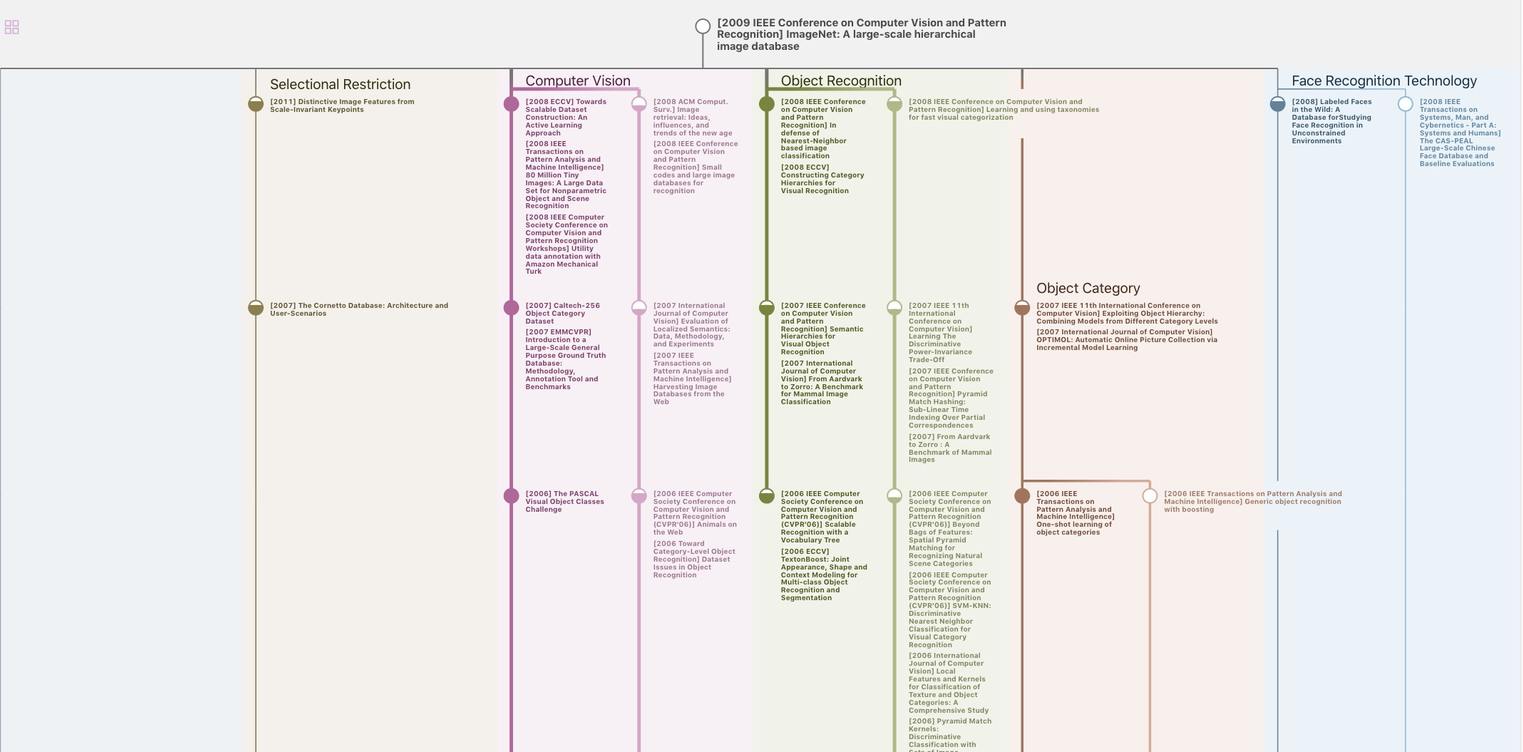
生成溯源树,研究论文发展脉络
Chat Paper
正在生成论文摘要