Safe Evaluation For Offline Learning: Are We Ready To Deploy?
semanticscholar(2021)
摘要
The world currently offers an abundance of data in multiple domains, from which we can learn reinforcement learning (RL) policies without further interaction with the environment. RL agents learning offline from such data is possible but deploying them while learning might be dangerous in domains where safety is critical. Therefore, it is essential to find a way to estimate how a newly-learned agent will perform if deployed in the target environment before actually deploying it and without the risk of overestimating its true performance. To achieve this, we introduce a framework for safe evaluation of offline learning using approximate high-confidence off-policy evaluation (HCOPE) to estimate the performance of offline policies during learning. In our setting, we assume a source of data, which we split into a train-set, to learn an offline policy, and a test-set, to estimate a lower-bound on the offline policy using off-policy evaluation with bootstrapping. A lower-bound estimate tells us how good a newly-learned target policy would perform before it is deployed in the real environment, and therefore allows us to decide when to deploy our learned policy.
更多查看译文
关键词
offline learning,evaluation
AI 理解论文
溯源树
样例
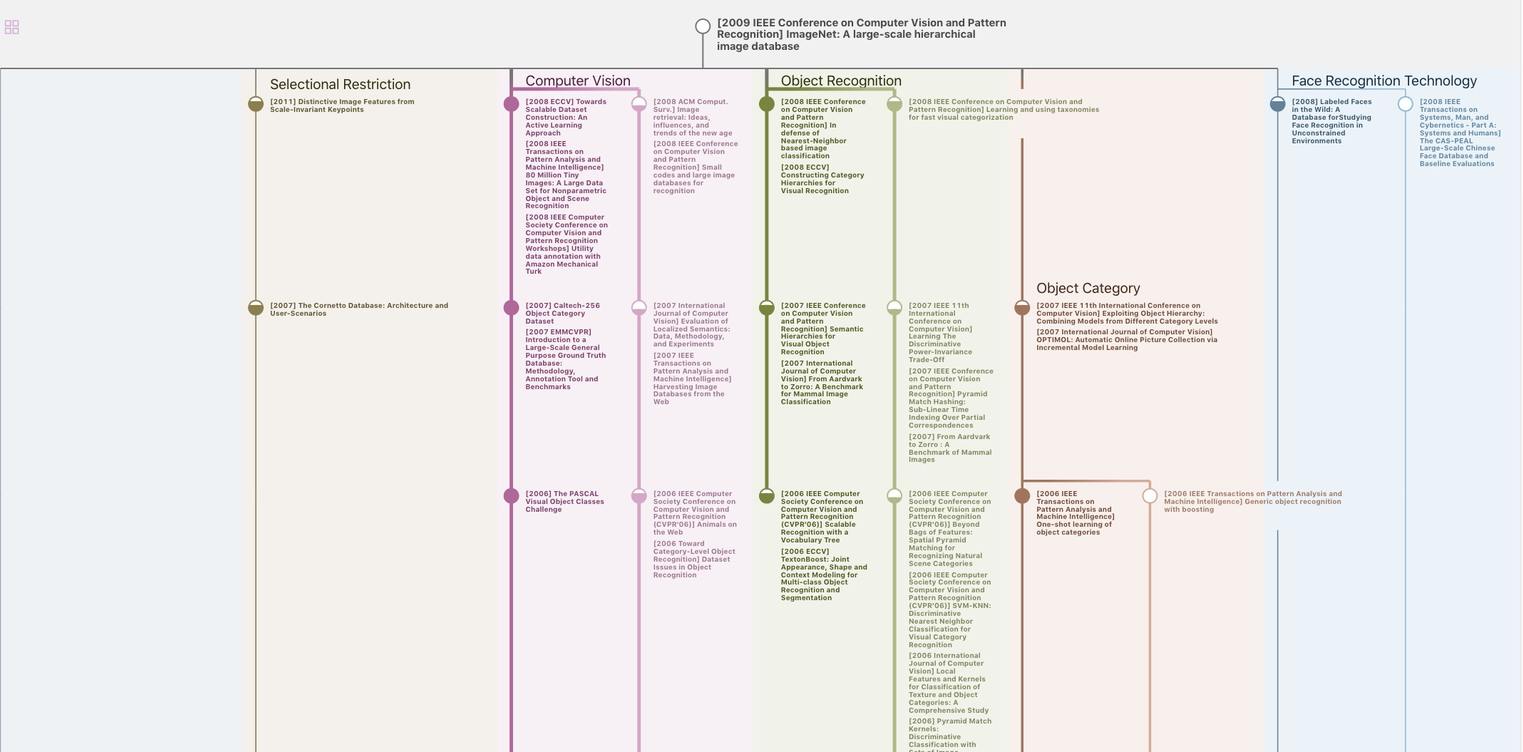
生成溯源树,研究论文发展脉络
Chat Paper
正在生成论文摘要