PATR: A Novel Poisoning Attack Based on Triangle Relations Against Deep Learning-Based Recommender Systems.
International Conference on Collaborative Computing (CollaborateCom)(2021)
Abstract
Recommender systems (RSs) have emerged as an effective way to deal with information overload and are very popular in e-commerce. However, because of the open nature of collaborative characteristics of the systems, RSs are susceptible to poisoning attacks, which inject fake user profiles into RSs to increase or decrease the recommended frequency of the target item. The traditional poisoning attack methods (such as random attack and average attack) are easy to be detected and lack of generality since they usually use global statistics, e.g., the number of each user’s ratings and the average rating for filler items. Moreover, as deep learning (DL) becomes more widely used in RSs, attackers are likely to use related techniques to attack RSs. To explore the robustness of DL-based RSs under the possible attacks, we propose a novel poisoning attack with triangle relations (PATR). The triangle relations refer to the balance among a fake user and two real users, aiming to improve attack performance. We also present a novel fake & real sampling strategy, i.e., sampling a set of fake users from the real users, to decrease the possibility of being detected. Comprehensive experiments on three public datasets show that PATR outperforms traditional poisoning attacks on attack effectiveness and anti-detection capability.
MoreTranslated text
Key words
Deep learning, Poisoning attack, Recommender system, Triangle relation
AI Read Science
Must-Reading Tree
Example
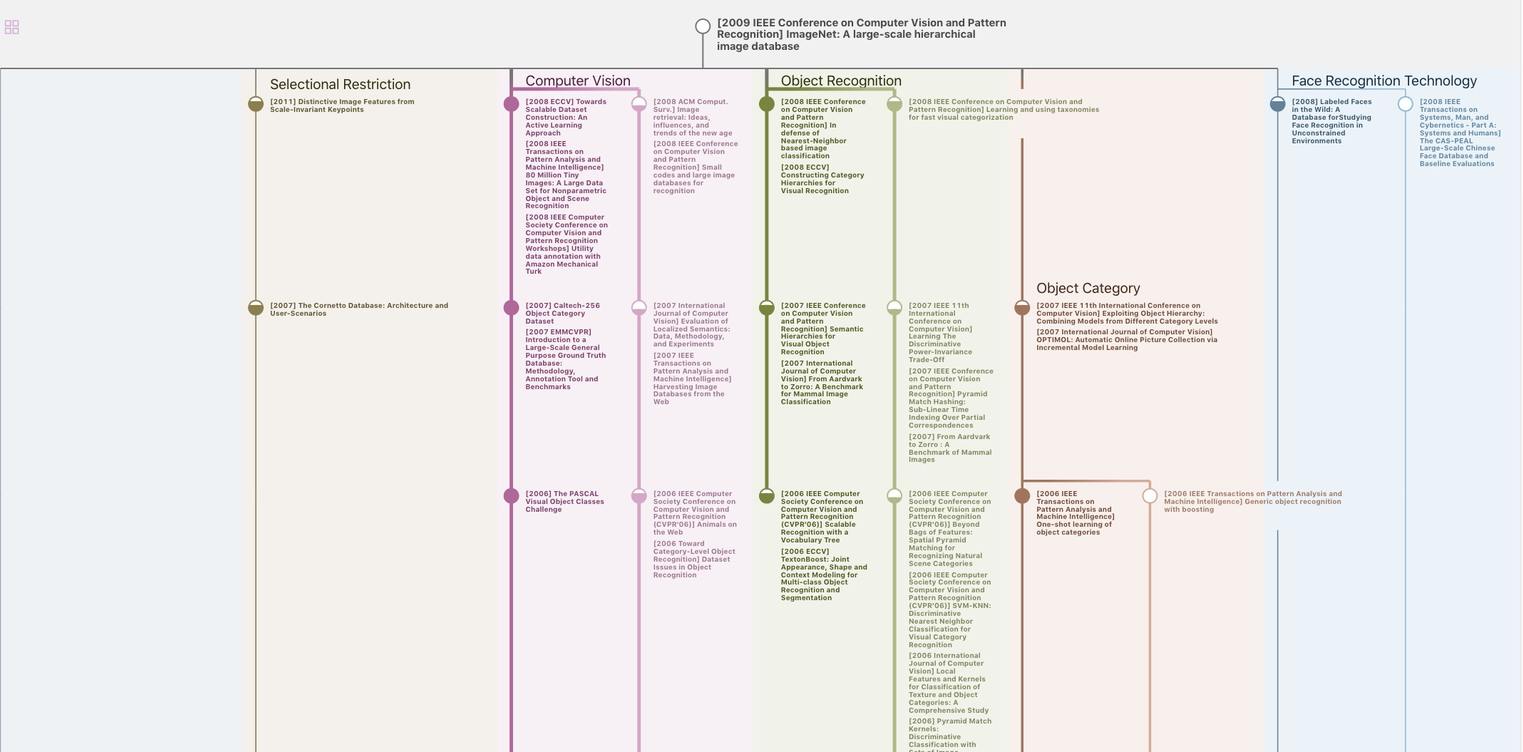
Generate MRT to find the research sequence of this paper
Chat Paper
Summary is being generated by the instructions you defined