Deep Reinforcement Learning Acceleration for Real-Time Edge Computing Mixed Integer Programming Problems
IEEE access(2022)
摘要
In this work, we present the design and implementation of an ultra-low latency Deep Reinforcement Learning (DRL) FPGA based accelerator for addressing hard real-time Mixed Integer Programming problems. The accelerator exhibits ultra-low latency performance for both training and inference operations, enabled by training-inference parallelism, pipelined training, on-chip weights and replay memory, multi-level replication-based parallelism and DRL algorithmic modifications such as distribution of training over time. The design principles can be extended to support hardware acceleration for other relevant DRL algorithms (embedding the experience replay technique) with hard real time constraints. We evaluate the accuracy of the accelerator in a task offloading and resource allocation problem stemming from a Mobile Edge Computing (MEC/5G) scenario. The design has been implemented on a Xilinx Zynq Ultrascale+ MPSoC ZCU104 evaluation kit using High Level Synthesis. The accelerator achieves near optimal performance and exhibits a 10-fold decrease in training-inference execution latency when compared to a high-end CPU-based implementation.
更多查看译文
关键词
Training,Inference algorithms,Real-time systems,Artificial neural networks,Task analysis,Resource management,Field programmable gate arrays,Accelerator,deep reinforcement learning,edge computing,FPGA,high level synthesis,mixed integer programming,5G
AI 理解论文
溯源树
样例
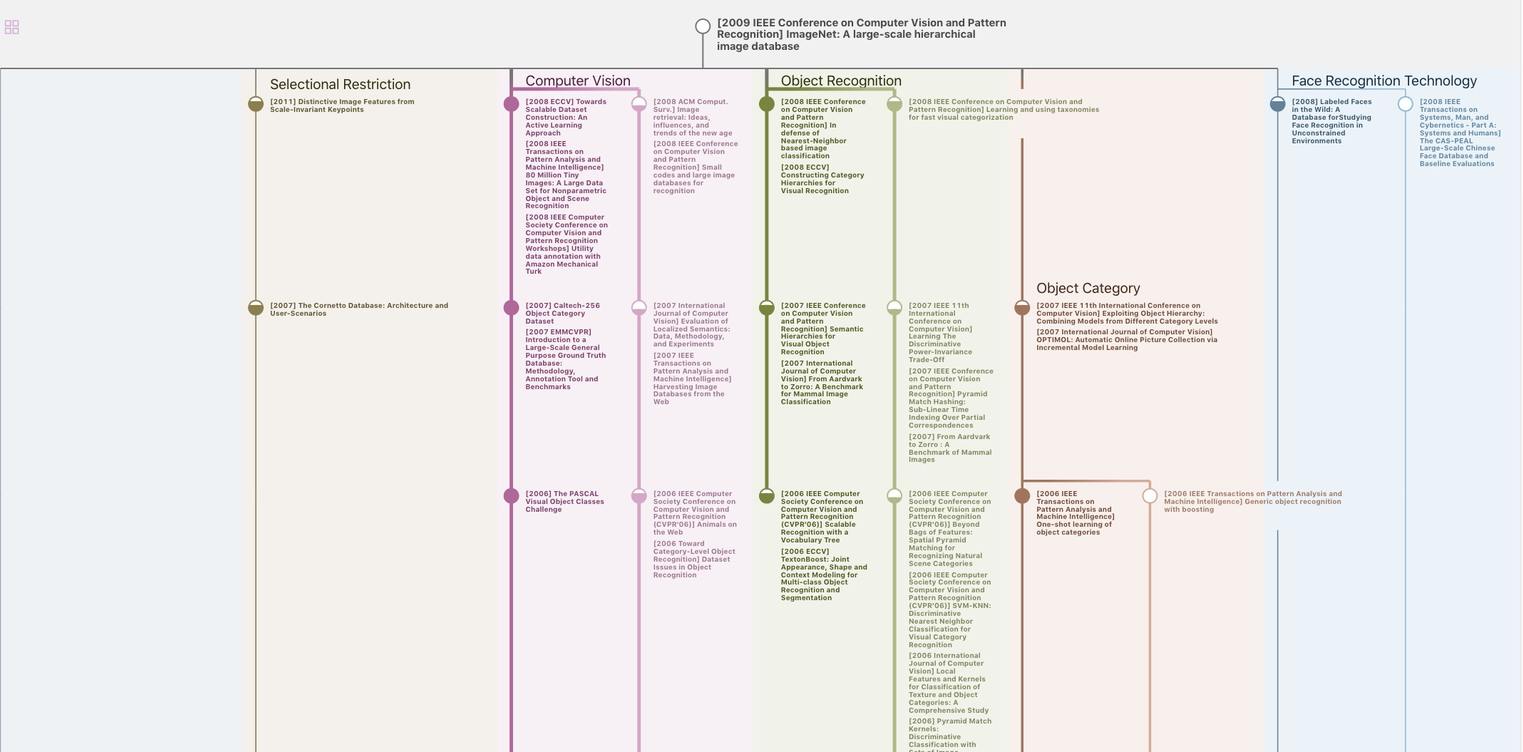
生成溯源树,研究论文发展脉络
Chat Paper
正在生成论文摘要