BAW: Learning from Class Imbalance and Noisy Labels with Batch Adaptation Weighted Loss.
Multimedia tools and applications(2022)
摘要
Deep learning has made significant achievements in the field of medical image processing. To train a robust model with strong generalization, a large-scale, high-quality dataset with balanced categories and correct labels is required. However, most datasets follow a long-tail distribution that some classes occupy most of the data, and other classes have only a few samples. At the same time, incorrect labels exist in the datasets. The existing methods focus on solving only one of these two problems, such as Focal Loss for class imbalance and mean-absolute error loss function for noisy labels. However, methods that try to alleviate one of the problems will aggravate the other. In order to tackle the class imbalance while avoids fitting the noisy labels, we propose a novel Batch Adaptation Weighted (BAW) loss. It uses the loss weights of known samples to guide the direction of network optimization for next batch training. BAW is easy to implement and can be extended to various deep networks to improve accuracy without any extra cost. We evaluate BAW on a general natural image dataset, CIFAR-10, and verify it on a large-scale medical image dataset, ChestX-ray14. Compared with existing algorithms, BAW gets best results on both datasets. Experiments shows that our algorithm can solve the problem of class imbalance and noisy labels at the same time. The code of our project is available at https://github.com/pansiyuan123/chestnet .
更多查看译文
关键词
Class imbalance,Noisy labels,Batch adaptation weighted,ChestX-ray14
AI 理解论文
溯源树
样例
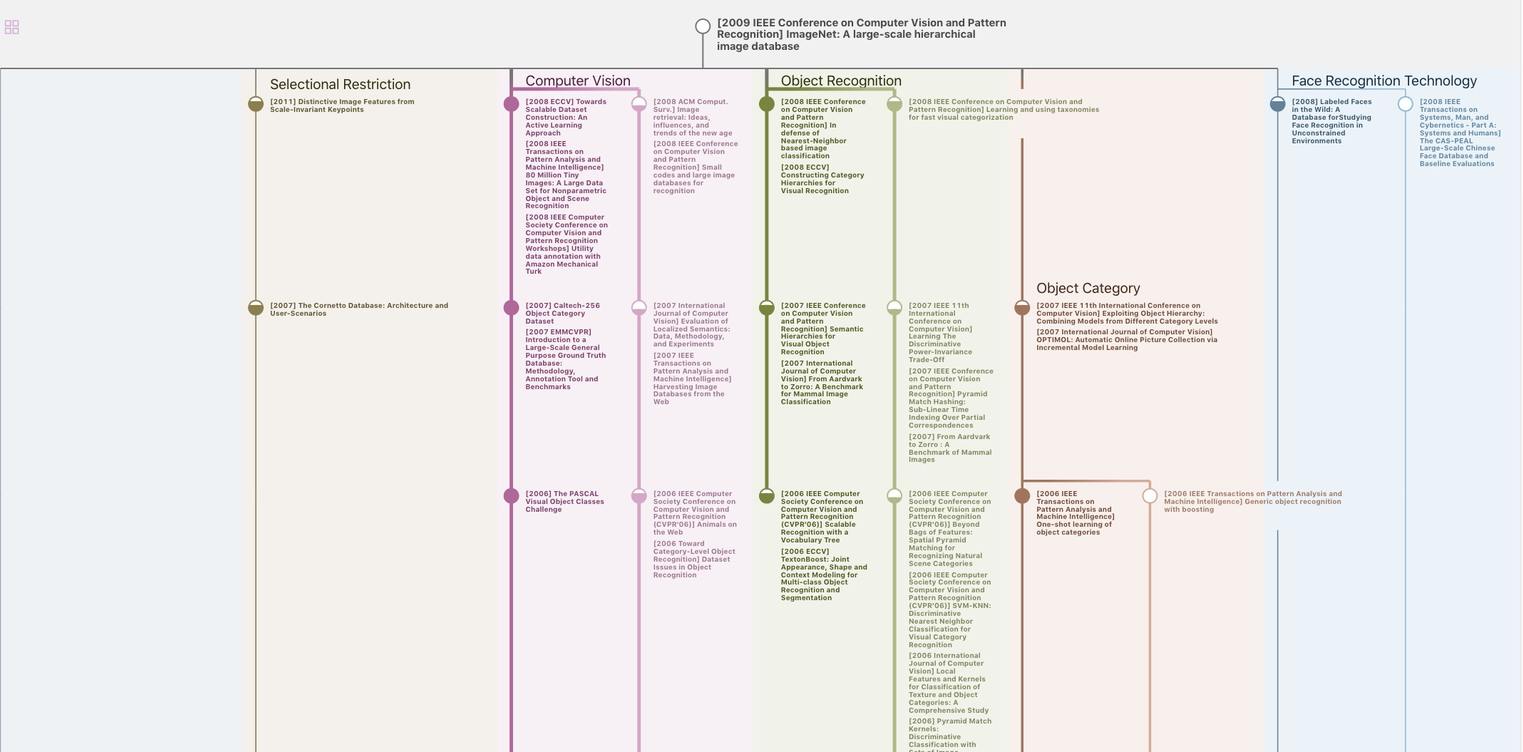
生成溯源树,研究论文发展脉络
Chat Paper
正在生成论文摘要