Diagnostic of Operation Conditions and Sensor Faults Using Machine Learning in Sucker-Rod Pumping Wells
SENSORS(2021)
摘要
In sucker-rod pumping wells, due to the lack of an early diagnosis of operating condition or sensor faults, several problems can go unnoticed. These problems can increase downtime and production loss. In these wells, the diagnosis of operation conditions is carried out through downhole dynamometer cards, via pre-established patterns, with human visual effort in the operation centers. Starting with machine learning algorithms, several papers have been published on the subject, but it is still common to have doubts concerning the difficulty level of the dynamometer card classification task and best practices for solving the problem. In the search for answers to these questions, this work carried out sixty tests with more than 50,000 dynamometer cards from 38 wells in the Mossoro, RN, Brazil. In addition, it presented test results for three algorithms (decision tree, random forest and XGBoost), three descriptors (Fourier, wavelet and card load values), as well as pipelines provided by automated machine learning. Tests with and without the tuning of hypermeters, different levels of dataset balancing and various evaluation metrics were evaluated. The research shows that it is possible to detect sensor failures from dynamometer cards. Of the results that will be presented, 75% of the tests had an accuracy above 92% and the maximum accuracy was 99.84%.
更多查看译文
关键词
sucker-rod pumping,machine learning algorithms,dynamometer card,petroleum industry
AI 理解论文
溯源树
样例
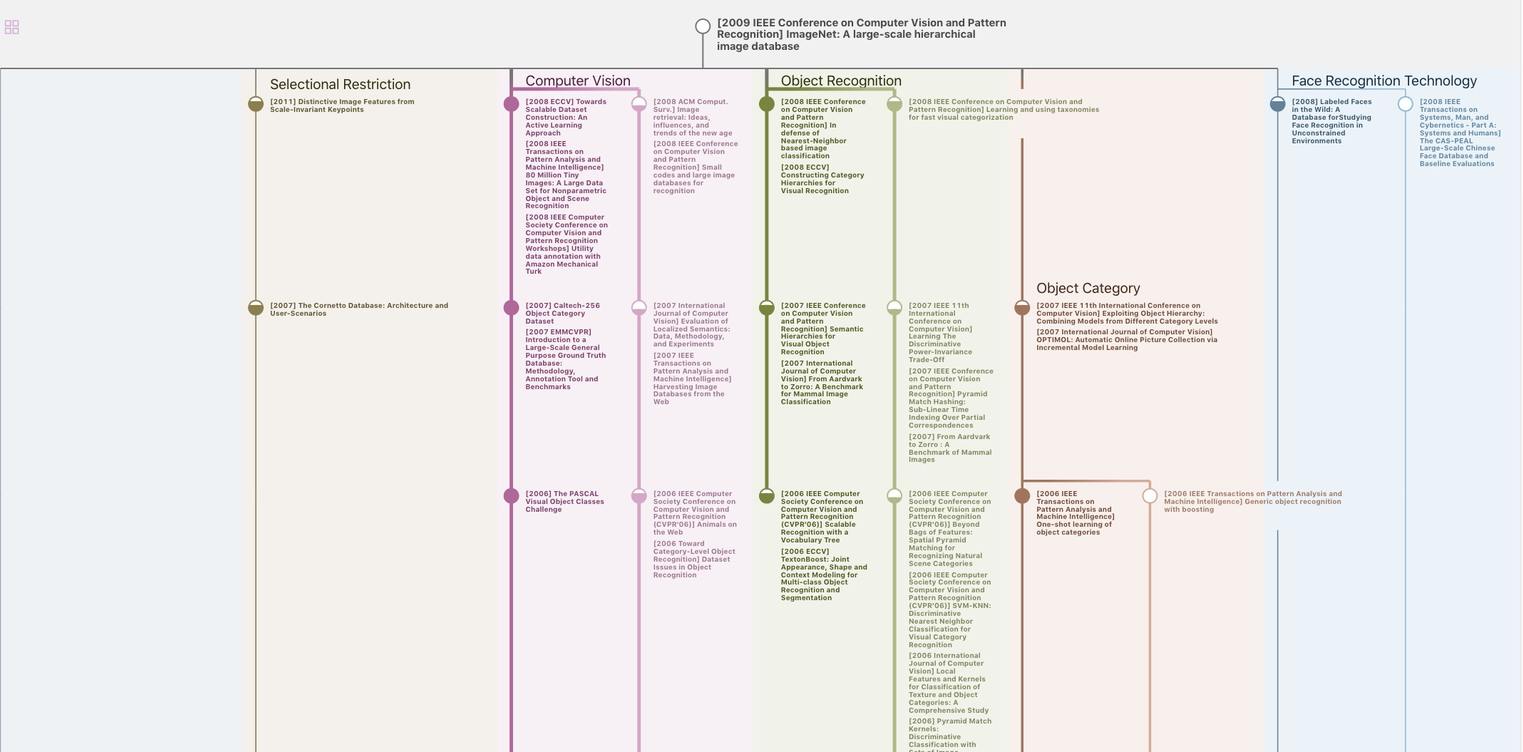
生成溯源树,研究论文发展脉络
Chat Paper
正在生成论文摘要