Impact of Artificial Intelligence on Miss Rate of Colorectal Neoplasia
Gastrointestinal Endoscopy(2022)
Mayo Clin | Univ Kansas | Queen Alexandra Hosp | John Radcliffe Hosp | Nuovo Regina Margherita Hosp | Klinikum Bayreuth GmbH | Cros NT | Mayo Clin Jacksonville | Mayo Clin LaCrosse | Mayo Clin Scottsdale | Mayo Clin Eau Claire | Geisinger Med Ctr | Ascens St John Hosp | Cosmo Artificial Intelligence AI Ltd | Humanitas Univ
Abstract
BACKGROUND & AIMS: Artificial intelligence (AI) may detect colorectal polyps that have been missed due to perceptual pitfalls. By reducing such miss rate, AI may increase the detection of colorectal neoplasia leading to a higher degree of colorectal cancer (CRC) prevention. METHODS: Patients undergoing CRC screening or surveillance were enrolled in 8 centers (Italy, UK, US), and randomized (1:1) to undergo 2 same-day, back-to-back colonoscopies with or without AI (deep learning computer aided diagnosis endoscopy) in 2 different arms, namely AI followed by colonoscopy without AI or vice-versa. Adenoma miss rate (AMR) was calculated as the number of histologically verified lesions detected at second colonoscopy divided by the total number of lesions detected at first and second colonoscopy. Mean number of lesions detected in the second colonoscopy and proportion of false negative subjects (no lesion at first colonoscopy and at least 1 at second) were calculated. Odds ratios (ORs) and 95% confidence intervals (CIs) were adjusted by endoscopist, age, sex, and indication for colonoscopy. Adverse events were also measured. RESULTS: A total of 230 subjects (116 AI first, 114 standard colonoscopy first) were included in the study analysis. AMR was 15.5% (38 of 246) and 32.4% (80 of 247) in the arm with AI and non-AI colonoscopy first, respectively (adjusted OR, 0.38; 95% CI, 0.23-0.62). In detail, AMR was lower for AI first for the <= 5 mm (15.9% vs 35.8%; OR, 0.34; 95% CI, 0.21-0.55) and nonpolypoid lesions (16.8% vs 45.8%; OR, 0.24; 95% CI, 0.13-0.43), and it was lower both in the proximal (18.3% vs 32.5%; OR, 0.46; 95% CI, 0.26-0.78) and distal colon (10.8% vs 32.1%; OR, 0.25; 95% CI, 0.11-0.57). Mean number of adenomas at second colonoscopy was lower in the AI-first group as compared with non-AI colonoscopy first (0.33 +/- 0.63 vs 0.70 +/- 0.97, P < .001). False negative rates were 6.8% (3 of 44 patients) and 29.6% (13 of 44) in the AI and non-AI first arms, respectively (OR, 0.17; 95% CI, 0.05-0.67). No difference in the rate of adverse events was found between the 2 groups. CONCLUSIONS: AI resulted in an approximately 2-fold reduction in miss rate of colorectal neoplasia, supporting AI-benefit in reducing perceptual errors for small and subtle lesions at standard colonoscopy.
MoreTranslated text
Key words
Colorectal Cancer,Artificial Intelligence,Miss Rate,Tandem Colonoscopy,Adenoma Miss Rate
求助PDF
上传PDF
View via Publisher
AI Read Science
AI Summary
AI Summary is the key point extracted automatically understanding the full text of the paper, including the background, methods, results, conclusions, icons and other key content, so that you can get the outline of the paper at a glance.
Example
Background
Key content
Introduction
Methods
Results
Related work
Fund
Key content
- Pretraining has recently greatly promoted the development of natural language processing (NLP)
- We show that M6 outperforms the baselines in multimodal downstream tasks, and the large M6 with 10 parameters can reach a better performance
- We propose a method called M6 that is able to process information of multiple modalities and perform both single-modal and cross-modal understanding and generation
- The model is scaled to large model with 10 billion parameters with sophisticated deployment, and the 10 -parameter M6-large is the largest pretrained model in Chinese
- Experimental results show that our proposed M6 outperforms the baseline in a number of downstream tasks concerning both single modality and multiple modalities We will continue the pretraining of extremely large models by increasing data to explore the limit of its performance
Upload PDF to Generate Summary
Must-Reading Tree
Example
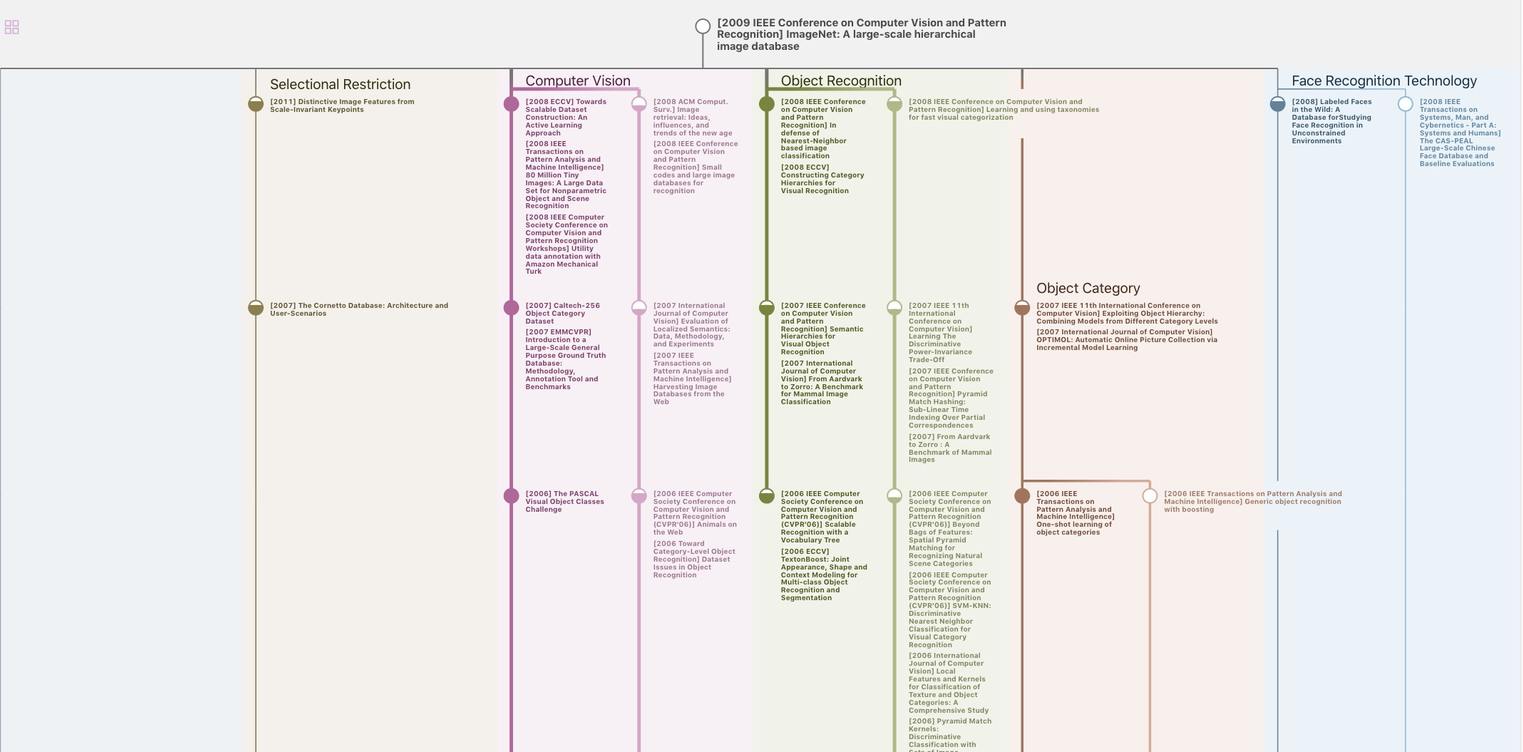
Generate MRT to find the research sequence of this paper
Related Papers
2009
被引用1127 | 浏览
2008
被引用1110 | 浏览
2018
被引用215 | 浏览
2019
被引用302 | 浏览
2019
被引用182 | 浏览
2020
被引用103 | 浏览
2020
被引用83 | 浏览
2020
被引用337 | 浏览
2020
被引用142 | 浏览
2020
被引用124 | 浏览
2003
被引用1502 | 浏览
2020
被引用52 | 浏览
2021
被引用100 | 浏览
2021
被引用55 | 浏览
2003
被引用1134 | 浏览
Data Disclaimer
The page data are from open Internet sources, cooperative publishers and automatic analysis results through AI technology. We do not make any commitments and guarantees for the validity, accuracy, correctness, reliability, completeness and timeliness of the page data. If you have any questions, please contact us by email: report@aminer.cn
Chat Paper