Panoptic Neural Fields: A Semantic Object-Aware Neural Scene Representation.
IEEE Conference on Computer Vision and Pattern Recognition(2022)
摘要
We present Panoptic Neural Fields (PNF), an object-aware neural scene representation that decomposes a scene into a set of objects (things) and background (stuff). Each object is represented by an oriented 3D bounding box and a multi-layer perceptron (MLP) that takes position, direction, and time and outputs density and radiance. The background stuff is represented by a similar MLP that additionally outputs semantic labels. Each object MLPs are instance-specific and thus can be smaller and faster than previous object-aware approaches, while still leveraging category-specific priors incorporated via meta-learned initialization. Our model builds a panoptic radiance field representation of any scene from just color images. We use off-the-shelf algorithms to predict camera poses, object tracks, and 2D image semantic segmentations. Then we jointly optimize the MLP weights and bounding box parameters using analysis-by-synthesis with self-supervision from color images and pseudo-supervision from predicted semantic segmentations. During experiments with real-world dynamic scenes, we find that our model can be used effectively for several tasks like novel view synthesis, 2D panoptic segmentation, 3D scene editing, and multiview depth prediction.
更多查看译文
关键词
scene,fields,semantic,object-aware
AI 理解论文
溯源树
样例
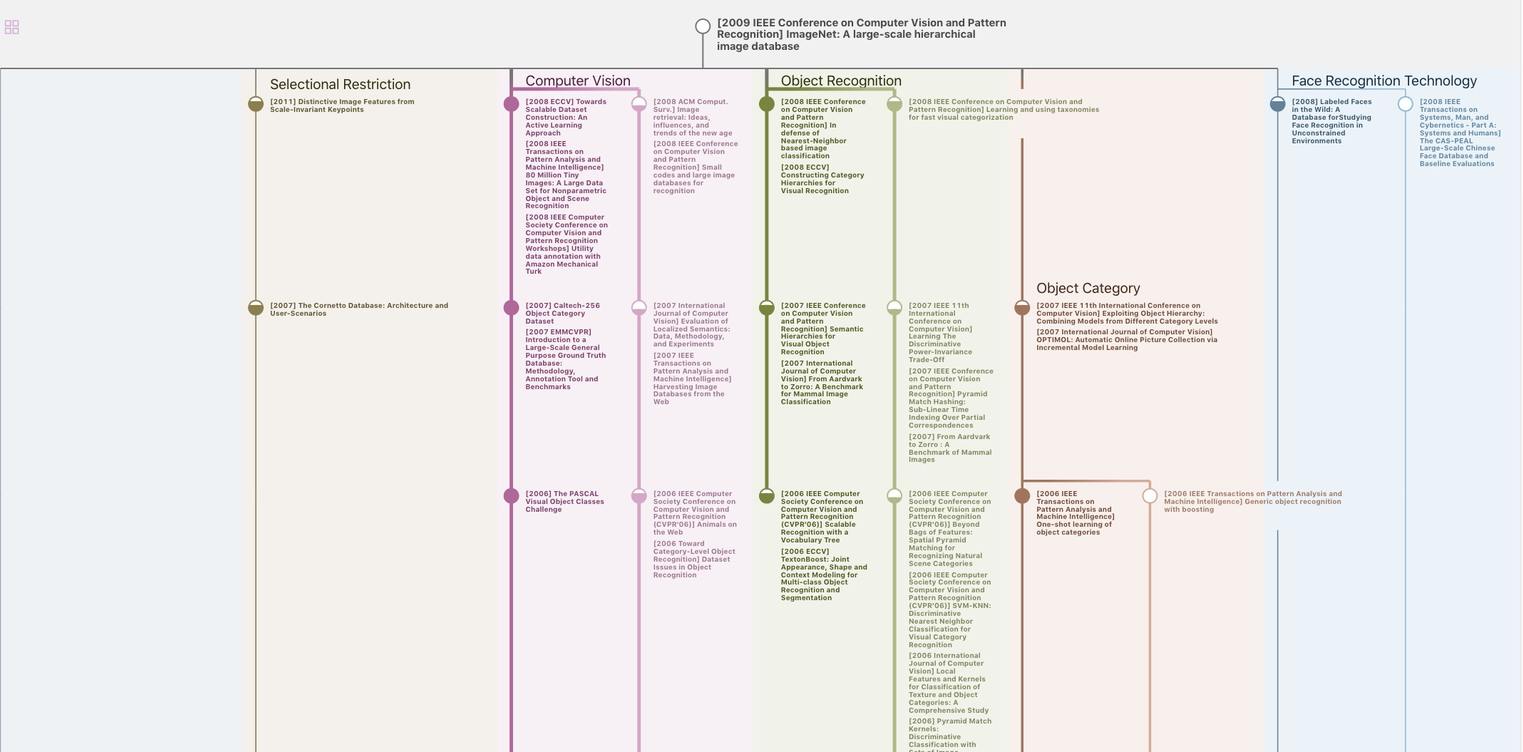
生成溯源树,研究论文发展脉络
Chat Paper
正在生成论文摘要