Learning-based Fuzzy Bitrate Matching at the Edge for Adaptive Video Streaming
International World Wide Web Conference(2022)
摘要
ABSTRACT The rapid growth of video traffic imposes significant challenges on content delivery over the Internet. Meanwhile, edge computing is developed to accelerate video transmission as well as release the traffic load of origin servers. Although some related techniques (e.g., transcoding and prefetching) are proposed to improve edge services, they cannot fully utilize cached videos. Therefore, we propose a Learning-based Fuzzy Bitrate Matching scheme (LFBM) at the edge for adaptive video streaming, which utilizes the capacity of network and edge servers. In accordance with user requests, cache states and network conditions, LFBM utilizes reinforcement learning to make a decision, either fetching the video of the exact bitrate from the origin server or responding with a different representation from the edge server. In the simulation, compared with the baseline, LFBM improves cache hit ratio by 128%. Besides, compared with the scheme without fuzzy bitrate matching, it improves Quality of Experience (QoE) by 45%. Moreover, the real-network experiments further demonstrate the effectiveness of LFBM. It increases the hit ratio by 84% compared with the baseline and improves the QoE by 51% compared with the scheme without fuzzy bitrate matching.
更多查看译文
关键词
DASH, Edge Computing, Reinforcement Learning
AI 理解论文
溯源树
样例
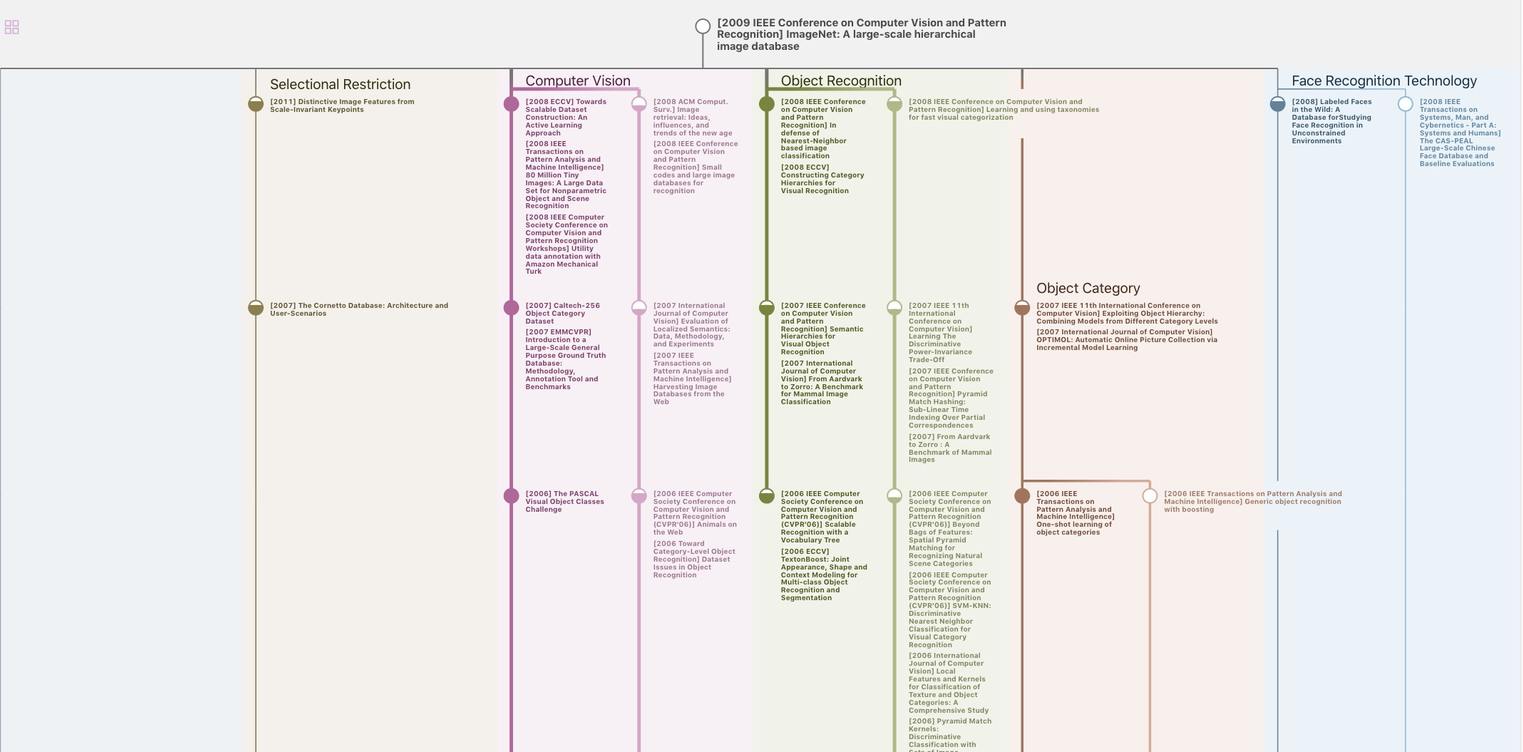
生成溯源树,研究论文发展脉络
Chat Paper
正在生成论文摘要