Generalized Quantifiers as a Source of Error in Multilingual NLU Benchmarks
North American Chapter of the Association for Computational Linguistics (NAACL)(2022)
摘要
Logical approaches to representing language have developed and evaluated computational models of quantifier words since the 19th century, but today's NLU models still struggle to capture their semantics. We rely on Generalized Quantifier Theory for language-independent representations of the semantics of quantifier words, to quantify their contribution to the errors of NLU models. We find that quantifiers are pervasive in NLU benchmarks, and their occurrence at test time is associated with performance drops. Multilingual models also exhibit unsatisfying quantifier reasoning abilities, but not necessarily worse for non-English languages. To facilitate directly-targeted probing, we present an adversarial generalized quantifier NLI task (GQNLI) and show that pre-trained language models have a clear lack of robustness in generalized quantifier reasoning.
更多查看译文
关键词
multilingual nlu
AI 理解论文
溯源树
样例
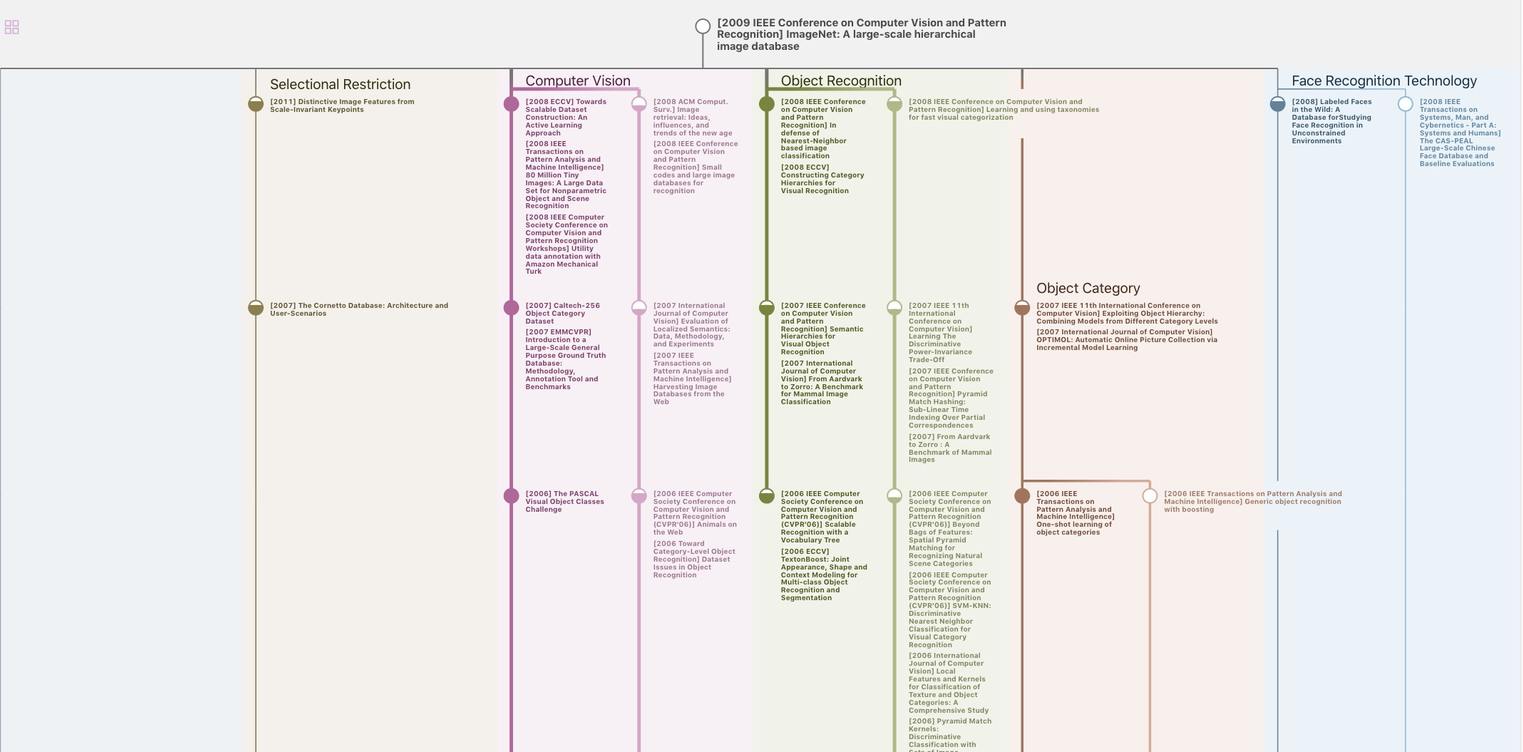
生成溯源树,研究论文发展脉络
Chat Paper
正在生成论文摘要