Extracting Targeted Training Data from ASR Models, and How to Mitigate It
Conference of the International Speech Communication Association (INTERSPEECH)(2022)
摘要
Recent work has designed methods to demonstrate that model updates in ASR training can leak potentially sensitive attributes of the utterances used in computing the updates. In this work, we design the first method to demonstrate information leakage about training data from trained ASR models. We design Noise Masking, a fill-in-the-blank style method for extracting targeted parts of training data from trained ASR models. We demonstrate the success of Noise Masking by using it in four settings for extracting names from the LibriSpeech dataset used for training a state-of-the-art Conformer model. In particular, we show that we are able to extract the correct names from masked training utterances with 11.8% accuracy, while the model outputs some name from the train set 55.2% of the time. Further, we show that even in a setting that uses synthetic audio and partial transcripts from the test set, our method achieves 2.5% correct name accuracy (47.7% any name success rate). Lastly, we design Word Dropout, a data augmentation method that we show when used in training along with Multistyle TRaining (MTR), provides comparable utility as the baseline, along with significantly mitigating extraction via Noise Masking across the four evaluated settings.
更多查看译文
关键词
Information extraction, Data augmentation
AI 理解论文
溯源树
样例
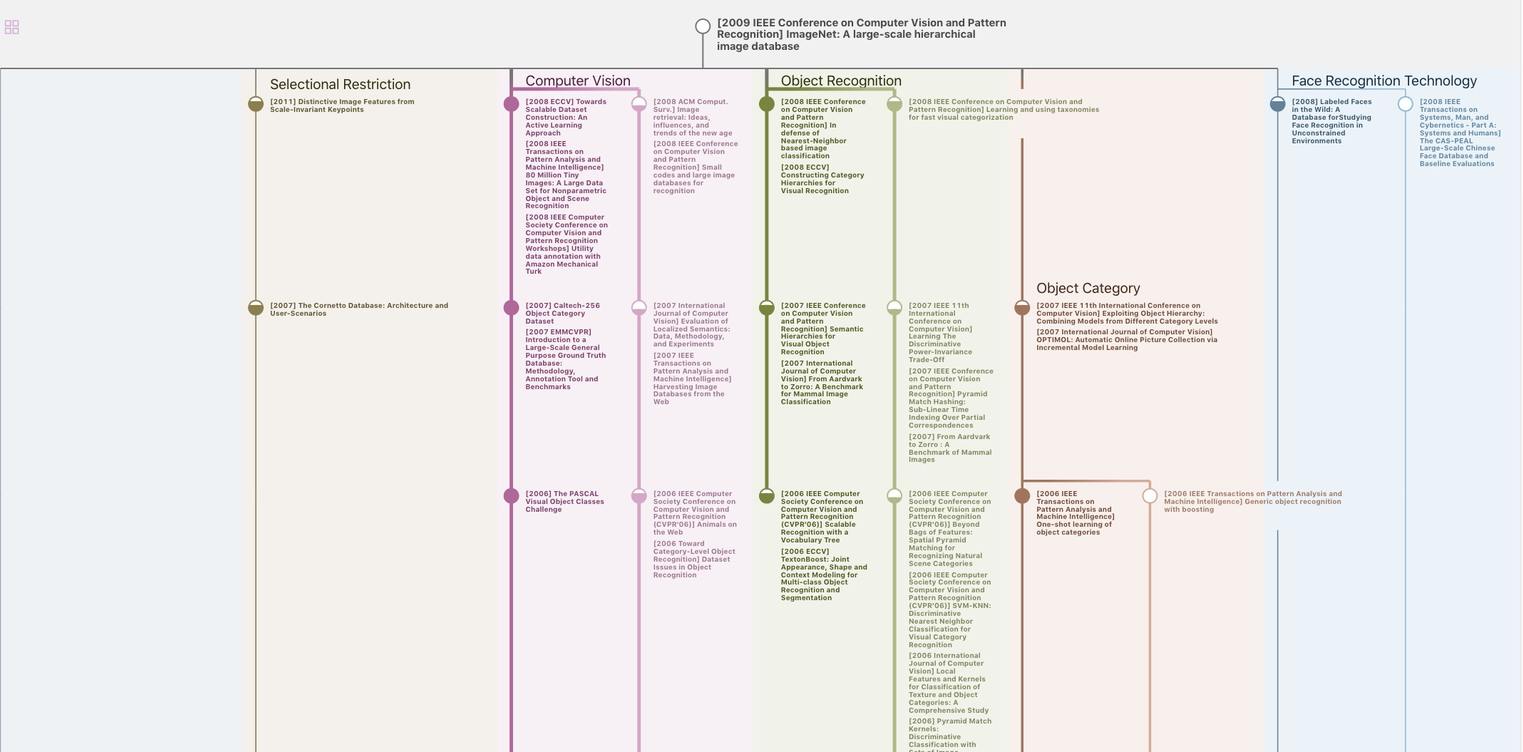
生成溯源树,研究论文发展脉络
Chat Paper
正在生成论文摘要