Research on the Rice Fertiliser Decision-Making Method Based on UAV Remote Sensing Data Assimilation
COMPUTERS AND ELECTRONICS IN AGRICULTURE(2024)
Abstract
Rice fertilisation is a crucial factor affecting the final yield of rice. Improving the fertiliser use rate and reducing fertiliser inputs to ensure stable rice yields are key issues to be solved in the process of large-scale rice production at present. Currently, most rice nutritional diagnoses rely on hyperspectral and multispectral data, but the spectral information of paddy fields has certain drawbacks compared with red, green, and blue (RGB) data in terms of data acquisition cost and reflectance calibration. Therefore, in this study, based on an unmanned aerial vehicle (UAV), remote sensing images were used to extract rice texture features, with rice fertiliser decision-making in northeast China as the research object. Through the design of different nitrogen fertiliser rates of rice field experiments and the use of data assimilation method to couple with the UAV RGB image data, RiceGrows, and ground-based Internet of Things (IoT) meteorological data, the Runge–Kutta optimiser-Extreme Learning Machine (RUN-ELM) method and UAV RGB images were used to construct a rice canopy leaf area index (LAI) inversion model. The model training set had an R2 of 0.822 and an RMSE of 0.647, and the validation set had an R2 of 0.791 and an RMSE of 0.592. Based on the Kalman Filter (KF) method, the Ensemble Kalman filter (EnKF) method, and the Particle Filter (PF) method, data assimilation of the collected LAI and the inverted LAI of the UAV visible remote sensing imagery was carried out to reduce the error and coupled with the RiceGrow. Remote sensing information was introduced into the RiceGrow to generate fertiliser decisions and to produce a prescription map for plant protection UAV operations to carry out accurate rice fertiliser applications. Through the fertilisation decision-making method adopted in this study, the rice yield in the four experimental plots was basically the same, with an average increase of 963.8 kg/ha. This study provides an effective technical way to predict the amount of fertiliser to be applied to rice with mechanisation and precision. Traditional fertiliser decision-making is guided by data on fertiliser application but lacks research on the agronomic mechanism in rice cultivation. However, this problem is solved in this study, which has important theoretical value and practical significance for the large-scale production of rice and the “reduction and efficiency” of nitrogen fertiliser application.
MoreTranslated text
Key words
Unmanned aerial vehicle (UAV),Machine learning,RiceGrow,RUN-ELM,Rice fertilisation,Leaf area index (LAI)
求助PDF
上传PDF
View via Publisher
AI Read Science
AI Summary
AI Summary is the key point extracted automatically understanding the full text of the paper, including the background, methods, results, conclusions, icons and other key content, so that you can get the outline of the paper at a glance.
Example
Background
Key content
Introduction
Methods
Results
Related work
Fund
Key content
- Pretraining has recently greatly promoted the development of natural language processing (NLP)
- We show that M6 outperforms the baselines in multimodal downstream tasks, and the large M6 with 10 parameters can reach a better performance
- We propose a method called M6 that is able to process information of multiple modalities and perform both single-modal and cross-modal understanding and generation
- The model is scaled to large model with 10 billion parameters with sophisticated deployment, and the 10 -parameter M6-large is the largest pretrained model in Chinese
- Experimental results show that our proposed M6 outperforms the baseline in a number of downstream tasks concerning both single modality and multiple modalities We will continue the pretraining of extremely large models by increasing data to explore the limit of its performance
Upload PDF to Generate Summary
Must-Reading Tree
Example
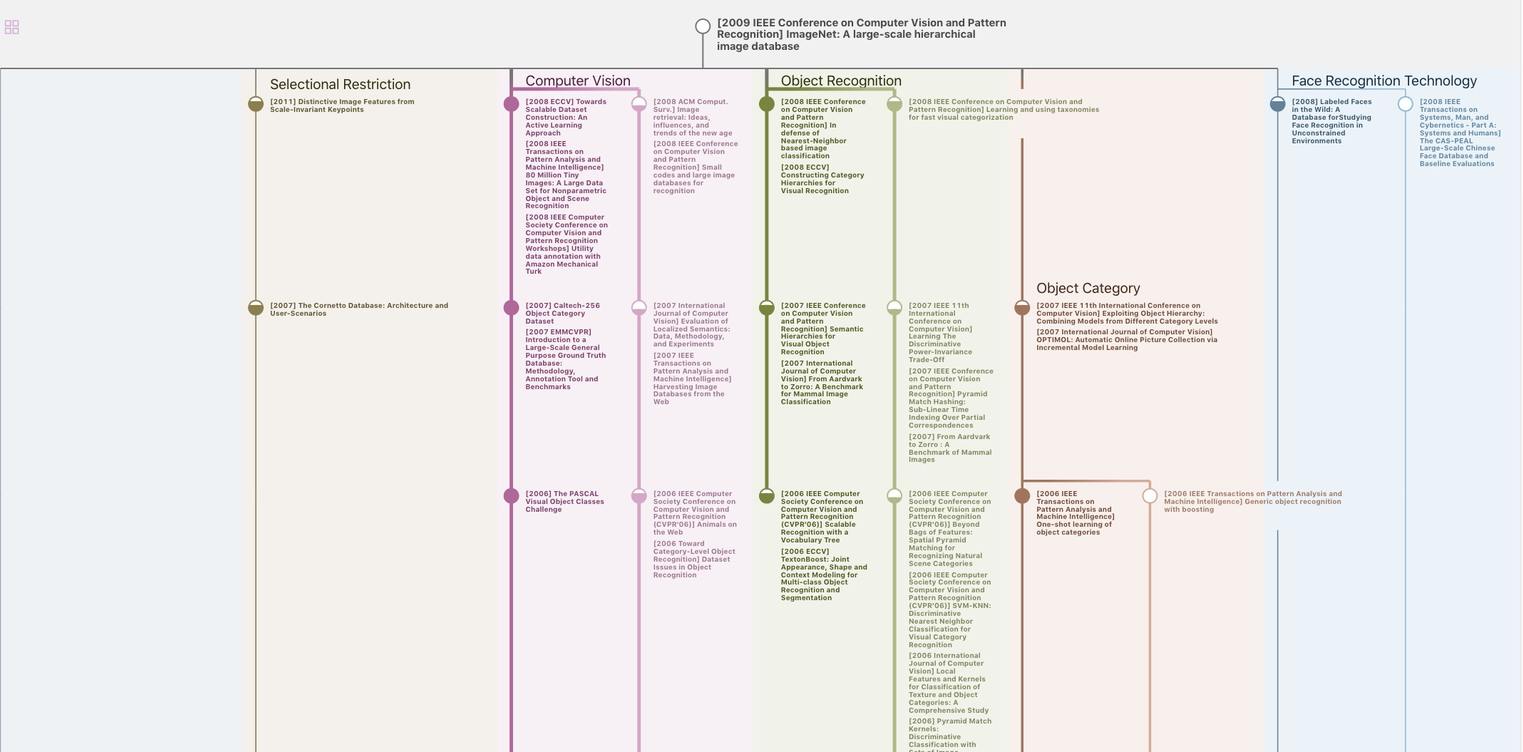
Generate MRT to find the research sequence of this paper
Data Disclaimer
The page data are from open Internet sources, cooperative publishers and automatic analysis results through AI technology. We do not make any commitments and guarantees for the validity, accuracy, correctness, reliability, completeness and timeliness of the page data. If you have any questions, please contact us by email: report@aminer.cn
Chat Paper