Learning to Compose Soft Prompts for Compositional Zero-Shot Learning
ICLR 2023(2022)
摘要
We introduce compositional soft prompting (CSP), a parameter-efficient learning technique to improve the zero-shot compositionality of large-scale pretrained vision-language models (VLMs). VLMs can represent arbitrary classes as natural language prompts in their flexible text encoders, but they underperform state-of-the-art methods on compositional zero-shot benchmark tasks. To improve VLMs, we propose a novel form of soft prompting. We treat the attributes and objects that are composed to define classes as learnable tokens of vocabulary and tune them on multiple prompt compositions. During inference, we recompose the learned attribute-object vocabulary in new combinations. We show that CSP outperforms the original VLM on benchmark datasets by an average of 10.9 percentage points on AUC. CSP also outperforms CoOp, a soft prompting method that tunes the prefix context, by an average of 5.8 percentage points on AUC. We perform additional experiments to show that CSP improves generalization to attribute-only classification, higher-order attribute-attribute-object compositions, and combinations of pretrained attributes and fine-tuned objects.
更多查看译文
关键词
compositional zero-shot learning,prompts,foundation models
AI 理解论文
溯源树
样例
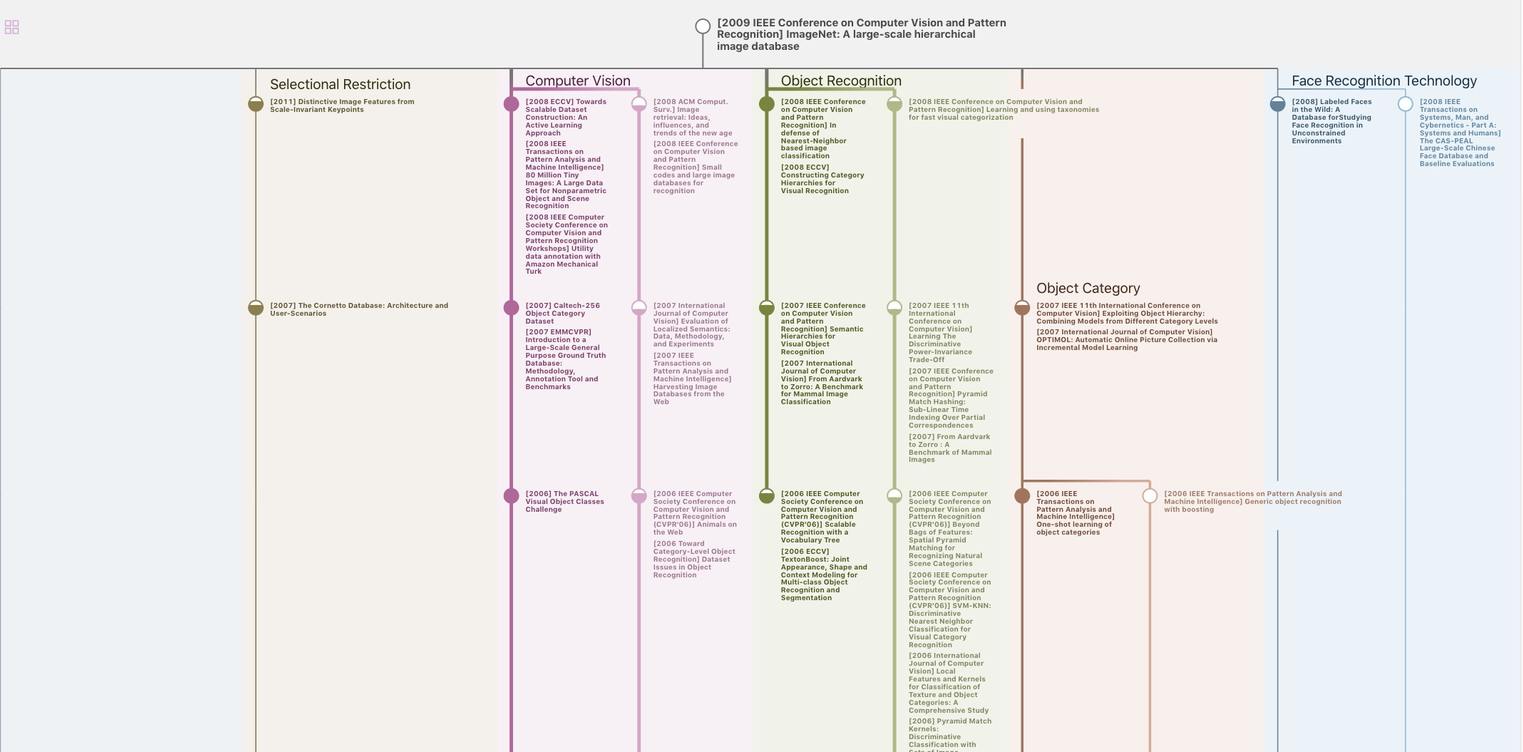
生成溯源树,研究论文发展脉络
Chat Paper
正在生成论文摘要