Leveraging Real Conversational Data for Multi-Channel Continuous Speech Separation
Conference of the International Speech Communication Association (INTERSPEECH)(2022)
摘要
Existing multi-channel continuous speech separation (CSS) models are heavily dependent on supervised data - either simulated data which causes data mismatch between the training and real-data testing, or the real transcribed overlapping data, which is difficult to be acquired, hindering further improvements in the conversational/meeting transcription tasks. In this paper, we propose a three-stage training scheme for the CSS model that can leverage both supervised data and extra large-scale unsupervised real-world conversational data. The scheme consists of two conventional training approaches -- pre-training using simulated data and ASR-loss-based training using transcribed data -- and a novel continuous semi-supervised training between the two, in which the CSS model is further trained by using real data based on the teacher-student learning framework. We apply this scheme to an array-geometry-agnostic CSS model, which can use the multi-channel data collected from any microphone array. Large-scale meeting transcription experiments are carried out on both Microsoft internal meeting data and the AMI meeting corpus. The steady improvement by each training stage has been observed, showing the effect of the proposed method that enables leveraging real conversational data for CSS model training.
更多查看译文
关键词
Continuous speech separation, array-geometry-agnostic, semi-supervised training, real unsupervised data
AI 理解论文
溯源树
样例
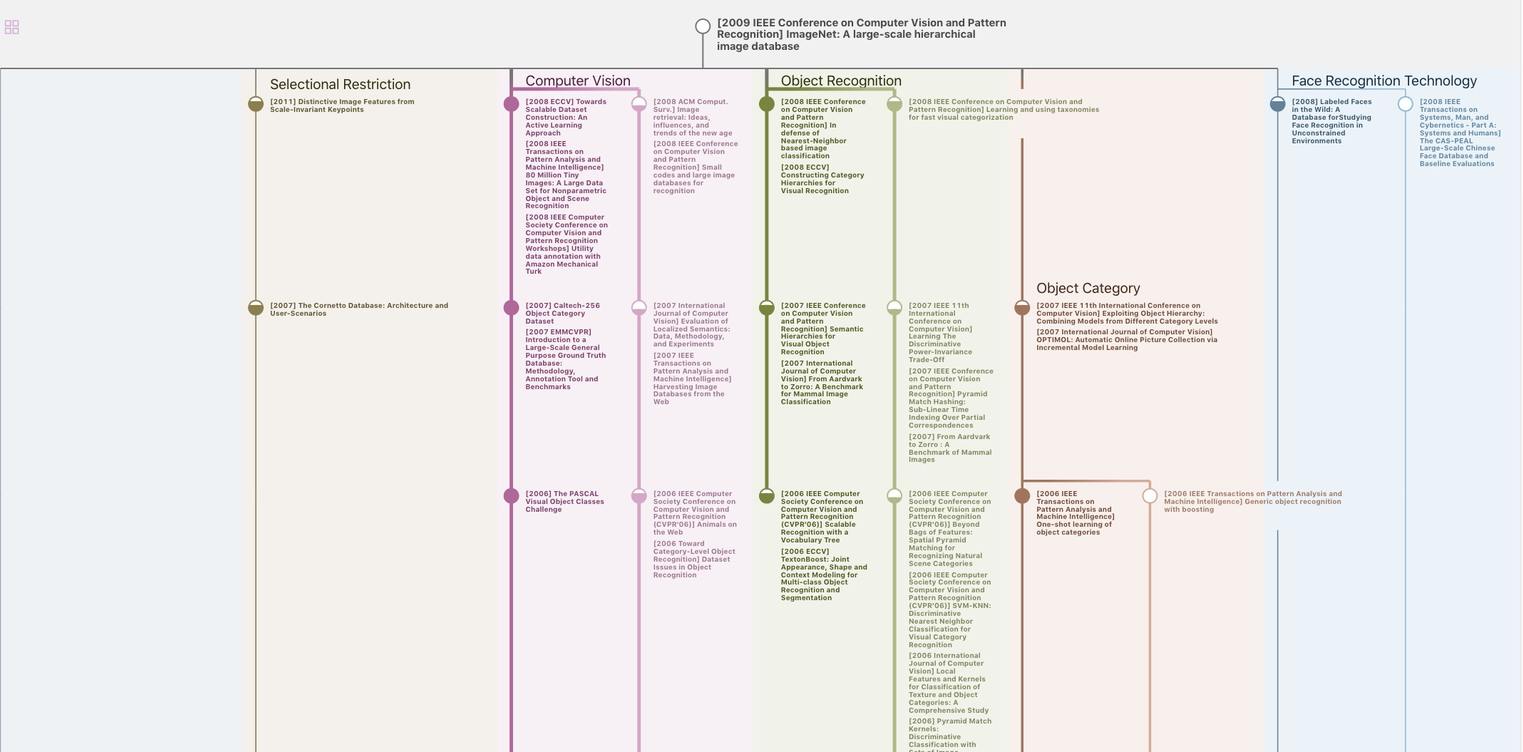
生成溯源树,研究论文发展脉络
Chat Paper
正在生成论文摘要