Incorporating information from LIGO data quality streams into the PyCBC search for gravitational waves
Physical Review D(2022)
摘要
We present a new method which accounts for changes in the properties of gravitational-wave detector noise over time in the PyCBC search for gravitational waves from compact binary coalescences. We use information from LIGO data quality streams that monitor the status of each detector and its environment to model changes in the rate of noise in each detector. These data quality streams allow candidates identified in the data during periods of detector malfunctions to be more efficiently rejected as noise. This method allows data from machine learning predictions of the detector state to be included as part of the PyCBC search, increasing the the total number of detectable gravitational-wave signals by up to 5%. When both machine learning classifications and manually-generated flags are used to search data from LIGO-Virgo's third observing run, the total number of detectable gravitational-wave signals is increased by up to 20% compared to not using any data quality streams. We also show how this method is flexible enough to include information from large numbers of additional arbitrary data streams that may be able to further increase the sensitivity of the search.
更多查看译文
关键词
gravitational waves,ligo data quality streams,pycbc
AI 理解论文
溯源树
样例
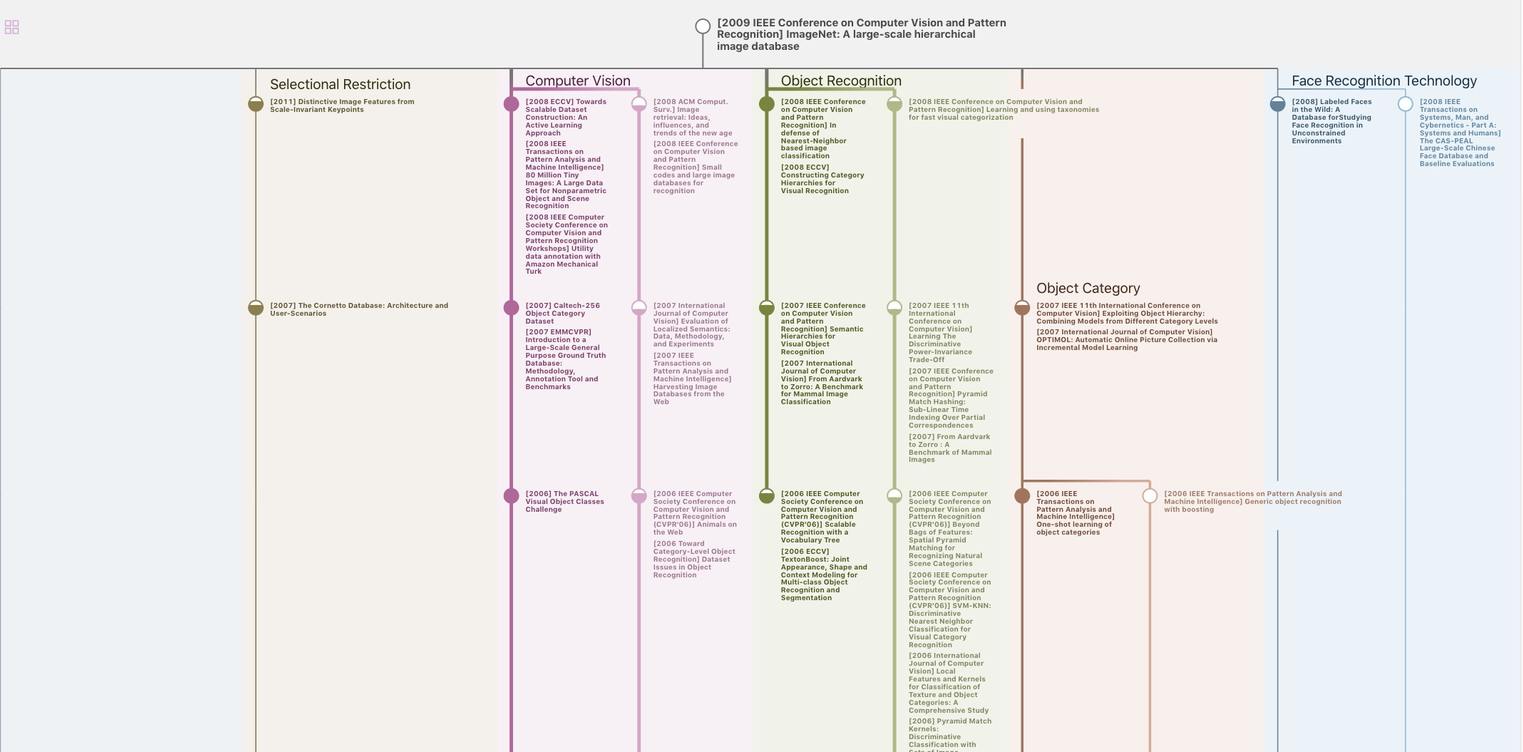
生成溯源树,研究论文发展脉络
Chat Paper
正在生成论文摘要