DBCal: Density Based Calibration of classifier predictions for uncertainty quantification
arXiv (Cornell University)(2022)
摘要
Measurement of uncertainty of predictions from machine learning methods is important across scientific domains and applications. We present, to our knowledge, the first such technique that quantifies the uncertainty of predictions from a classifier and accounts for both the classifier's belief and performance. We prove that our method provides an accurate estimate of the probability that the outputs of two neural networks are correct by showing an expected calibration error of less than 0.2% on a binary classifier, and less than 3% on a semantic segmentation network with extreme class imbalance. We empirically show that the uncertainty returned by our method is an accurate measurement of the probability that the classifier's prediction is correct and, therefore has broad utility in uncertainty propagation.
更多查看译文
关键词
uncertainty,classifier predictions,density based calibration,quantification
AI 理解论文
溯源树
样例
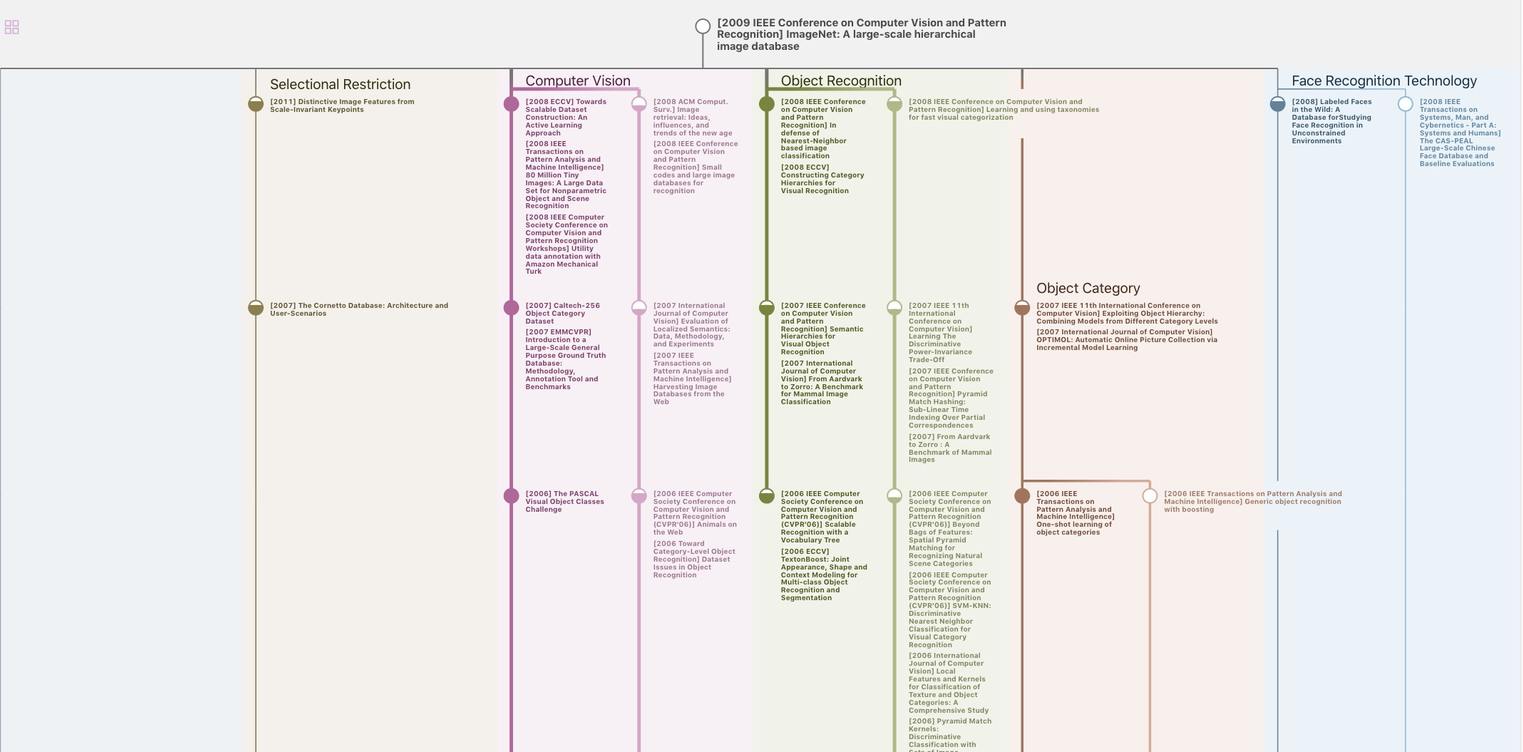
生成溯源树,研究论文发展脉络
Chat Paper
正在生成论文摘要