Recent Improvements of ASR Models in the Face of Adversarial Attacks
Interspeech 2022(2022)
摘要
Like many other tasks involving neural networks, Speech Recognition models are vulnerable to adversarial attacks. However recent research has pointed out differences between attacks and defenses on ASR models compared to image models. Improving the robustness of ASR models requires a paradigm shift from evaluating attacks on one or a few models to a systemic approach in evaluation. We lay the ground for such research by evaluating on various architectures a representative set of adversarial attacks: targeted and untargeted, optimization and speech processing-based, white-box, black-box and targeted attacks. Our results show that the relative strengths of different attack algorithms vary considerably when changing the model architecture, and that the results of some attacks are not to be blindly trusted. They also indicate that training choices such as self-supervised pretraining can significantly impact robustness by enabling transferable perturbations. We release our source code as a package that should help future research in evaluating their attacks and defenses.
更多查看译文
关键词
speech recognition,security,robustness,adversarial attacks
AI 理解论文
溯源树
样例
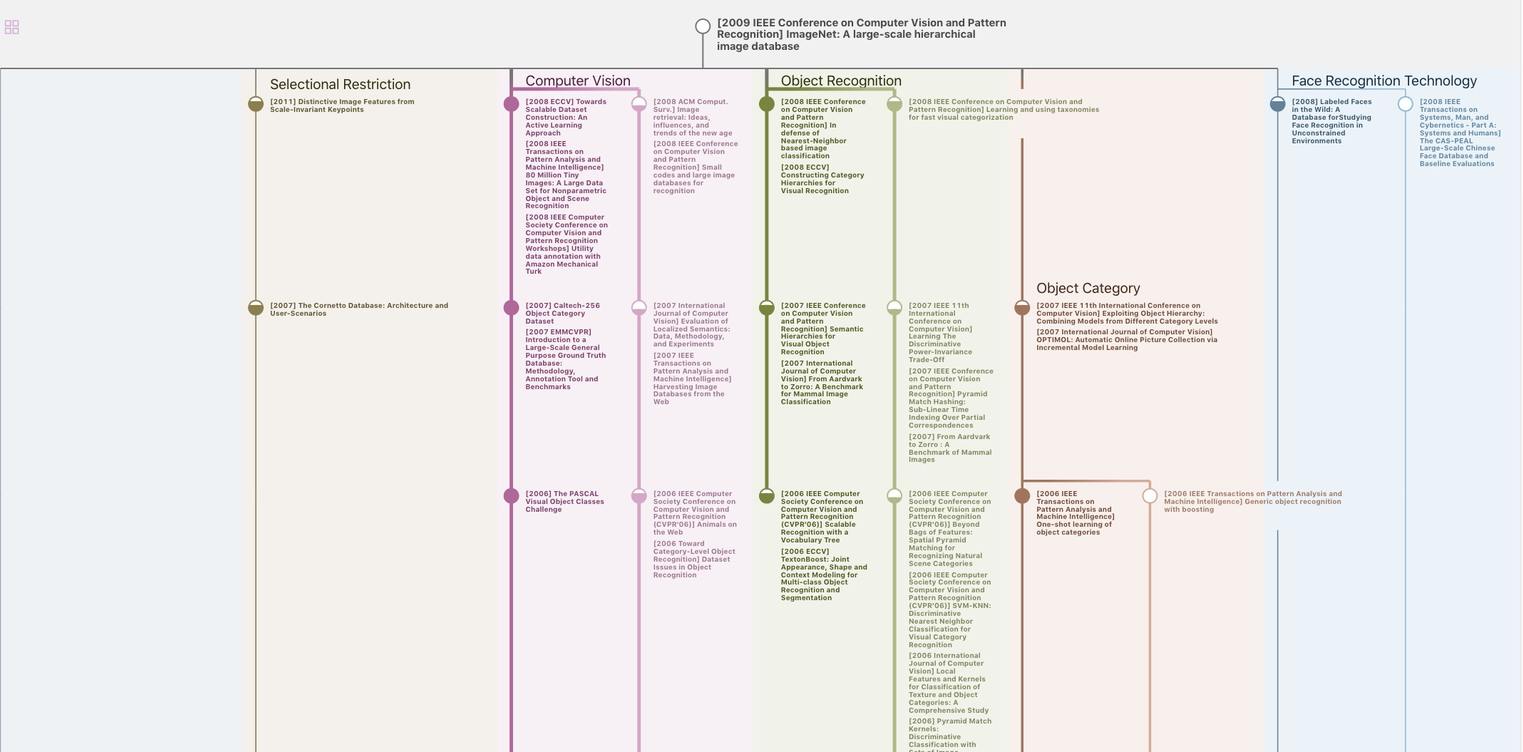
生成溯源树,研究论文发展脉络
Chat Paper
正在生成论文摘要