A machine learning method for estimating the probability of presence using presence-background data
biorxiv(2022)
摘要
Estimating the prevalence or the absolute probability of presence of a species from presence-background data has become a controversial topic in species distribution modelling. In this paper we propose a new method by combining both statistics and machine learning algorithms that helps overcome some of the known existing problems. We have also revisited the popular but highly controversial Lele and Keim (LK) method by evaluating its performance and assessing the RSPF condition it relies on. Simulations show that the LK method with unfounded model assumptions would render fragile estimation/prediction of the desired probabilities. Rather we propose the local knowledge condition, which relaxes the pre-determined population prevalence condition that has so often been used in much of the existing literature. Simulations demonstrate the performance of the CLK method utilising the local knowledge assumption to successfully estimate the probability of presence. The local knowledge extends the local certainty or the prototypical presence location assumption, and has significant implications for demonstrating the necessary condition for identifying absolute (rather than relative) probability of presence without absence data in species distribution modelling.
### Competing Interest Statement
The authors have declared no competing interest.
更多查看译文
关键词
constrained LK method,local certainty,local knowledge,presence‐background,prevalence,probability of presence
AI 理解论文
溯源树
样例
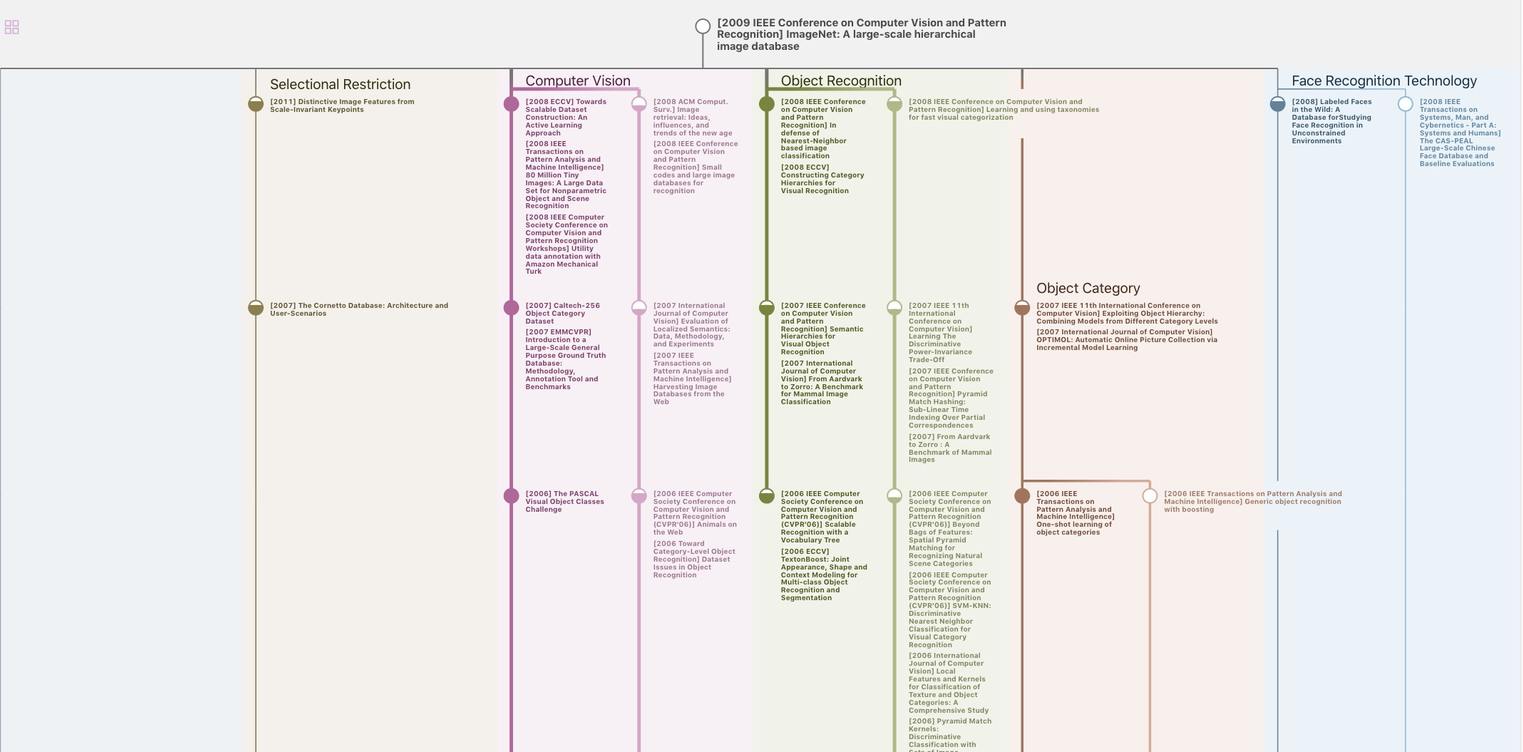
生成溯源树,研究论文发展脉络
Chat Paper
正在生成论文摘要