Panoptic NeRF: 3D-to-2D Label Transfer for Panoptic Urban Scene Segmentation
2022 International Conference on 3D Vision (3DV)(2022)
摘要
Large-scale training data with high-quality annotations is critical for training semantic and instance segmentation models. Unfortunately, pixel-wise annotation is labor-intensive and costly, raising the demand for more efficient labeling strategies. In this work, we present a novel 3D-to-2D label transfer method, Panoptic NeRF1, which aims for obtaining per-pixel 2D semantic and instance labels from easy-to-obtain coarse 3D bounding primitives. Our method utilizes NeRF as a differentiable tool to unify coarse 3D annotations and 2D semantic cues transferred from existing datasets. We demonstrate that this combination allows for improved geometry guided by semantic information, enabling rendering of accurate semantic maps across multiple views. Furthermore, this fusion process resolves label ambiguity of the coarse 3D annotations and filters noise in the 2D predictions. By inferring in 3D space and rendering to 2D labels, our 2D semantic and instance labels are multiview consistent by design. Experimental results show that Panoptic NeRF outperforms existing label transfer methods in terms of accuracy and multi-view consistency on challenging urban scenes of the KITTI-360 dataset.
更多查看译文
关键词
panoptic urban scene
AI 理解论文
溯源树
样例
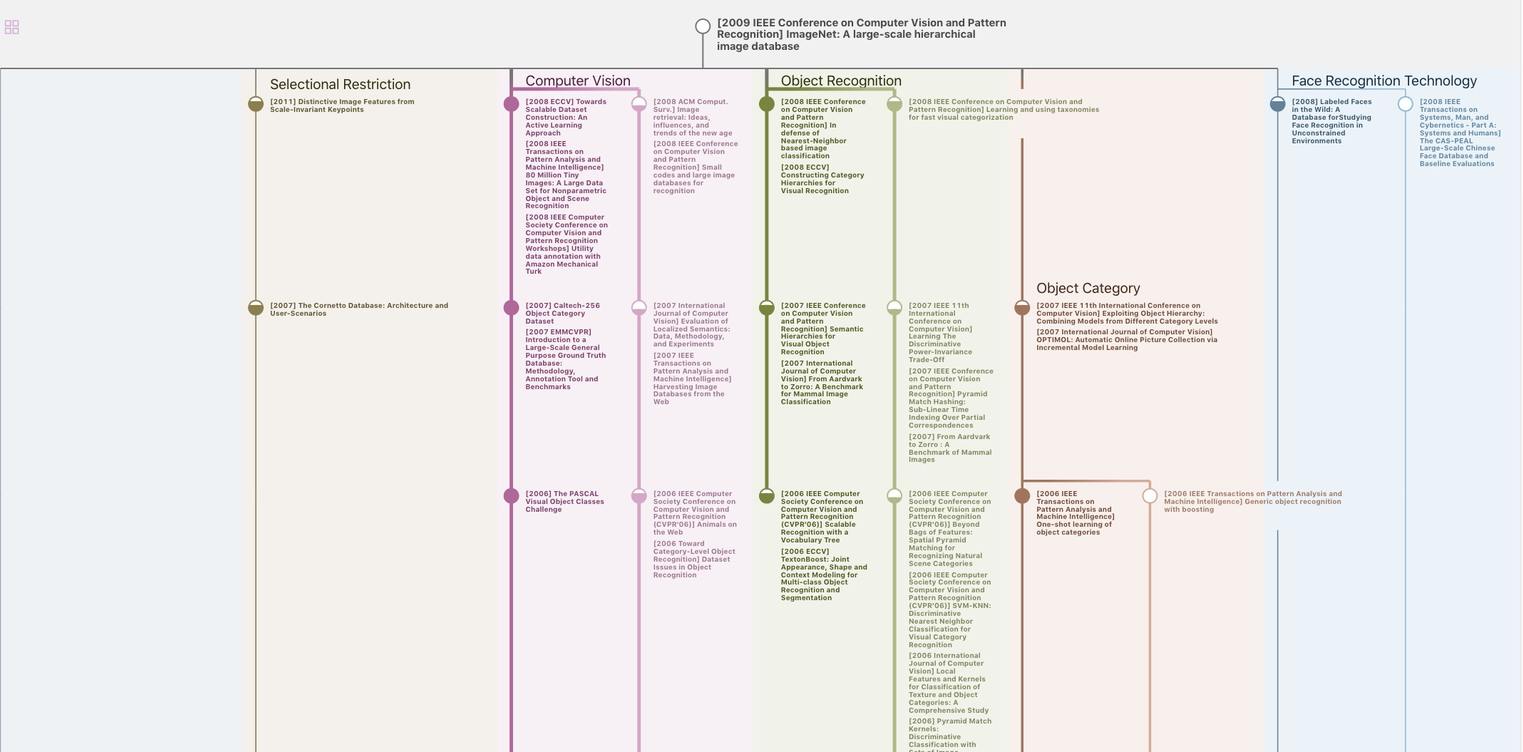
生成溯源树,研究论文发展脉络
Chat Paper
正在生成论文摘要