A Morphometric Analysis of the Osteocyte Canaliculus Using Applied Automatic Semantic Segmentation by Machine Learning
Journal of bone and mineral metabolism(2022)
摘要
Osteocytes play a role as mechanosensory cells by sensing flow-induced mechanical stimuli applied on their cell processes. High-resolution imaging of osteocyte processes and the canalicular wall are necessary for the analysis of this mechanosensing mechanism. Focused ion beam-scanning electron microscopy (FIB-SEM) enabled the visualization of the structure at the nanometer scale with thousands of serial-section SEM images. We applied machine learning for the automatic semantic segmentation of osteocyte processes and canalicular wall and performed a morphometric analysis using three-dimensionally reconstructed images. Six-week-old-mice femur were used. Osteocyte processes and canaliculi were observed at a resolution of 2 nm/voxel in a 4 × 4 μm region with 2000 serial-section SEM images. Machine learning was used for automatic semantic segmentation of the osteocyte processes and canaliculi from serial-section SEM images. The results of semantic segmentation were evaluated using the dice similarity coefficient (DSC). The segmented data were reconstructed to create three-dimensional images and a morphological analysis was performed. The DSC was > 83%. Using the segmented data, a three-dimensional image of approximately 3.5 μm in length was reconstructed. The morphometric analysis revealed that the median osteocyte process diameter was 73.8 ± 18.0 nm, and the median pericellular fluid space around the osteocyte process was 40.0 ± 17.5 nm. We used machine learning for the semantic segmentation of osteocyte processes and canalicular wall for the first time, and performed a morphological analysis using three-dimensionally reconstructed images.
更多查看译文
关键词
Osteocyte process,Osteocyte canaliculus,Machine learning,Fluid shear stress,FIB-SEM
AI 理解论文
溯源树
样例
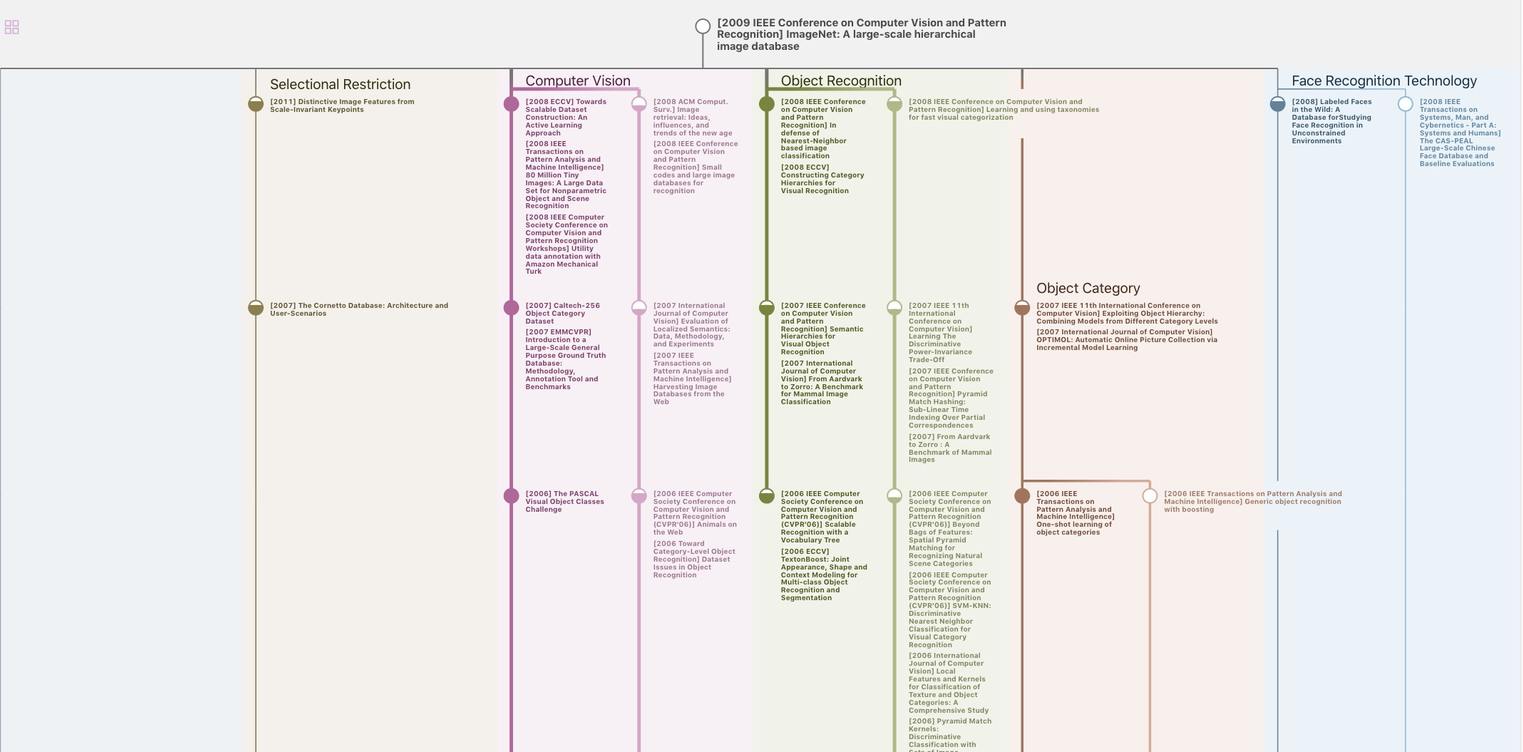
生成溯源树,研究论文发展脉络
Chat Paper
正在生成论文摘要