Open-set Recognition via Augmentation-based Similarity Learning
ArXiv(2022)
摘要
The primary assumption of conventional supervised learning or classification is that the test samples are drawn from the same distribution as the training samples, which is called closed set learning or classification. In many practical scenarios, this is not the case because there are unknowns or unseen class samples in the test data, which is called the open set scenario, and the unknowns need to be detected. This problem is referred to as the open set recognition problem and is important in safety-critical applications. We propose to detect unknowns (or unseen class samples) through learning pairwise similarities. The proposed method works in two steps. It first learns a closed set classifier using the seen classes that have appeared in training and then learns how to compare seen classes with pseudo-unseen (automatically generated unseen class samples). The pseudo-unseen generation is carried out by performing distribution shifting augmentations on the seen or training samples. We call our method OPG (Open set recognition based on Pseudo unseen data Generation). The experimental evaluation shows that the learned similarity-based features can successfully distinguish seen from unseen in benchmark datasets for open set recognition.
更多查看译文
关键词
recognition,learning,open-set,augmentation-based
AI 理解论文
溯源树
样例
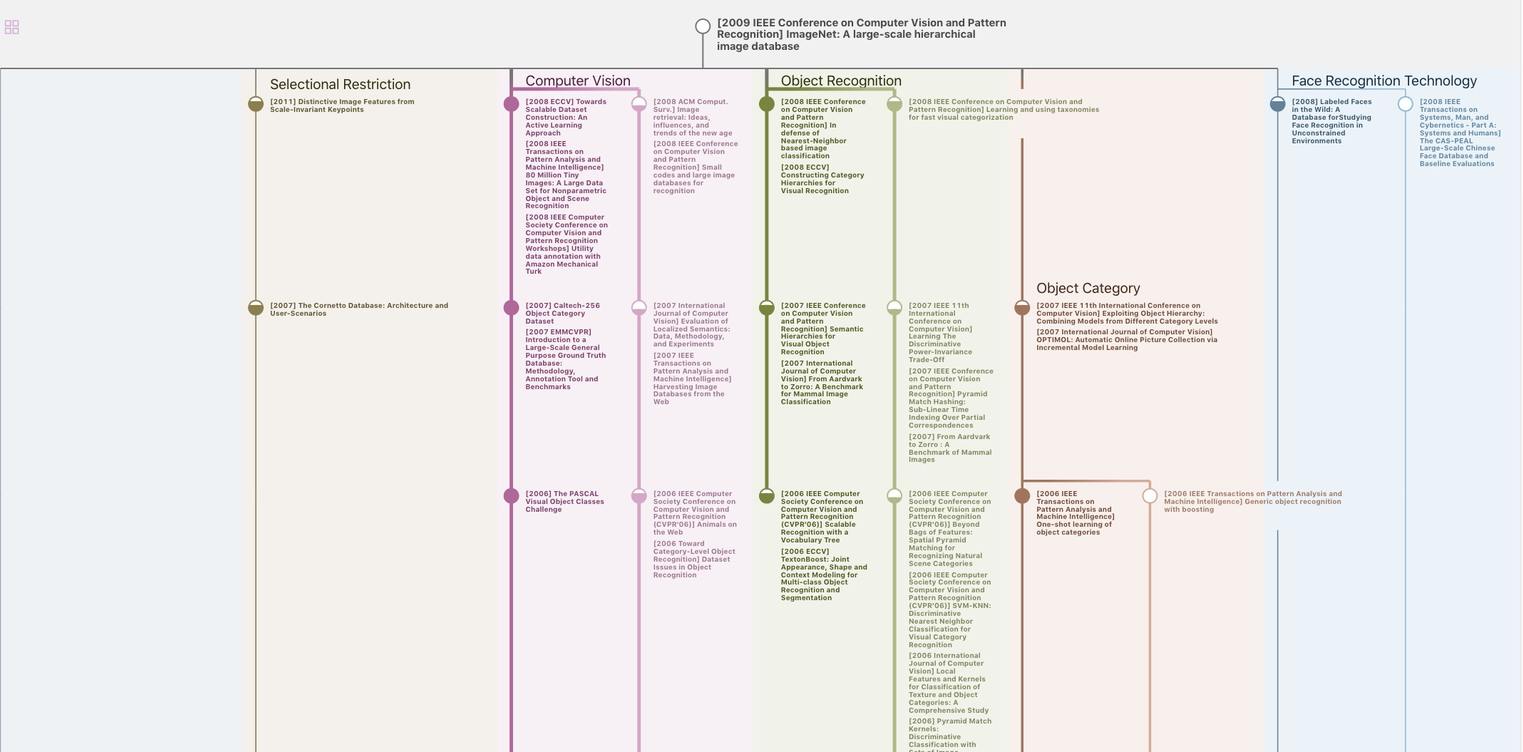
生成溯源树,研究论文发展脉络
Chat Paper
正在生成论文摘要