Impact of material data in assembly delay prediction—a machine learning-based case study in machinery industry
The International Journal of Advanced Manufacturing Technology(2022)
摘要
Designing customized products for customer needs is a key characteristic of machine and plant manufacturers. Their manufacturing process typically consists of a design phase followed by planning and executing a production process of components required in the subsequent assembly. Production delays can lead to a delayed start of the assembly. Predicting potentially delayed components—we call those components assembly start delayers—in early phases of the manufacturing process can support an on-time assembly. In recent research, prediction models typically include information about the orders, workstations, and the status of the manufacturing system, but information about the design of the component is not used. Since the components of machine and plant manufacturers are designed specifically for the customer needs, we assumed that material data influence the quality of a model predicting assembly start delayers. To analyze our hypothesis, we followed the established CRISP-DM method to set up 12 prediction models at an exemplary chosen machine and plant manufacturer utilizing a binary classification approach. These 12 models differentiated in the utilization of material data—including or excluding material data—and in the utilized machine learning algorithm—six algorithms per data case. Evaluating the different models revealed a positive impact of the material data on the model quality. With the achieved results, our study validates the benefit of using material data in models predicting assembly start delayers. Thus, we identified that considering data sources, which are commonly not used in prediction models, such as material data, increases the model quality.
更多查看译文
关键词
Production control,Assembly,Prediction methods,Lead time reduction,Machine learning,Supervised Learning,Classification algorithms,Data analysis
AI 理解论文
溯源树
样例
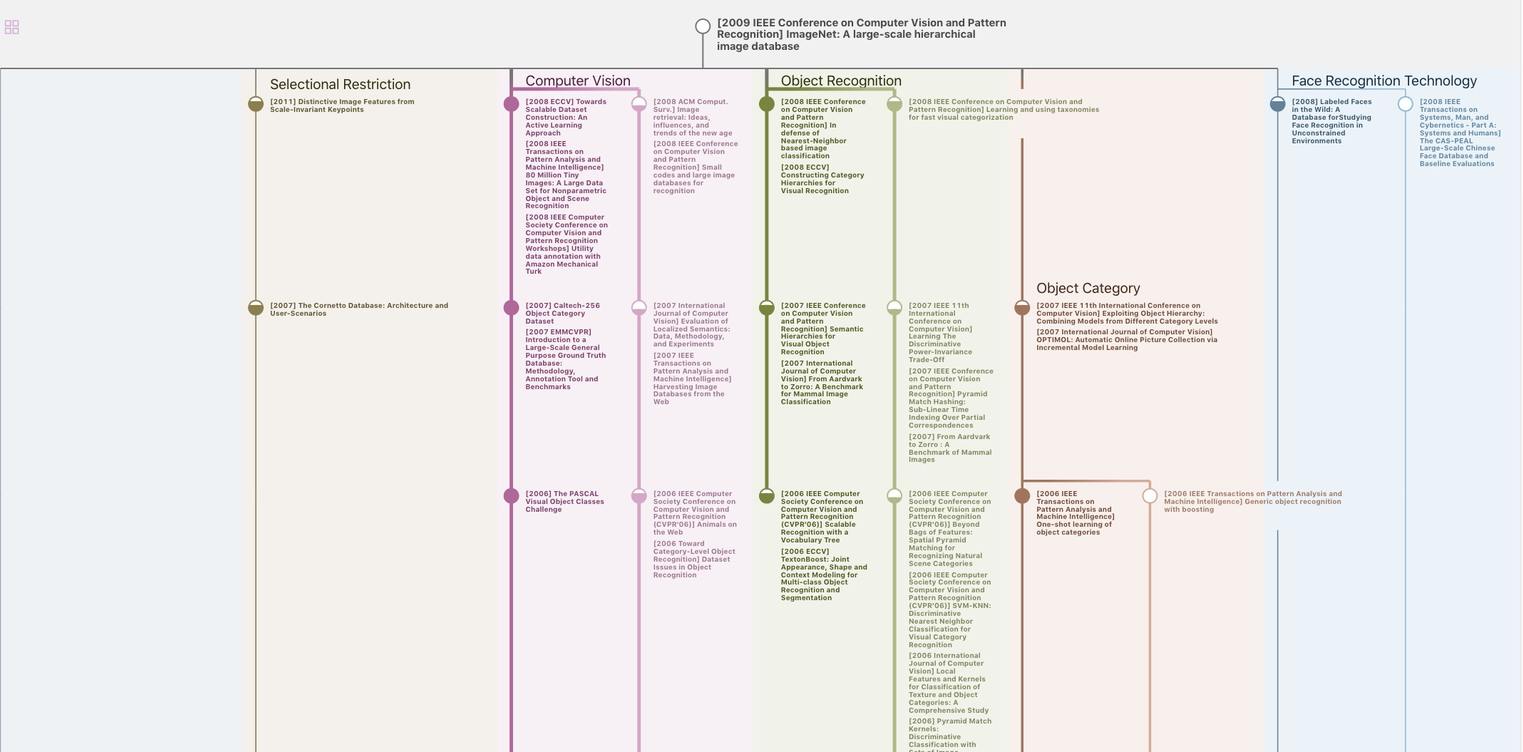
生成溯源树,研究论文发展脉络
Chat Paper
正在生成论文摘要