Hierarchical autoregressive neural networks for statistical systems
COMPUTER PHYSICS COMMUNICATIONS(2022)
摘要
It was recently proposed that neural networks could be used to approximate many-dimensional probability distributions that appear e.g. in lattice field theories or statistical mechanics. Subsequently they can be used as variational approximators to assess extensive properties of statistical systems, like free energy, and also as neural samplers used in Monte Carlo simulations. The practical application of this approach is unfortunately limited by its unfavourable scaling both of the numerical cost required for training, and the memory requirements with the system size. This is due to the fact that the original proposition involved a neural network of width which scaled with the total number of degrees of freedom, e.g. L-2 in case of a two dimensional L x L lattice. In this work we propose a hierarchical association of physical degrees of freedom, for instance spins, to neurons which replaces it with the scaling with the linear extent L of the system. We demonstrate our approach on the two-dimensional Ising model by simulating lattices of various sizes up to 128 x 128 spins, with time benchmarks reaching lattices of size 512 x 512. We observe that our proposal improves the quality of neural network training, i.e. the approximated probability distribution is closer to the target that could be previously achieved. As a consequence, the variational free energy reaches a value closer to its theoretical expectation and, if applied in a Markov Chain Monte Carlo algorithm, the resulting autocorrelation time is smaller. Finally, the replacement of a single neural network by a hierarchy of smaller networks considerably reduces the memory requirements. (C) 2022 The Author(s). Published by Elsevier B.V.
更多查看译文
关键词
Variational autoregressive neural networks, Hierarchical neural networks, Spin systems, Ising model, Markov Chain Monte Carlo
AI 理解论文
溯源树
样例
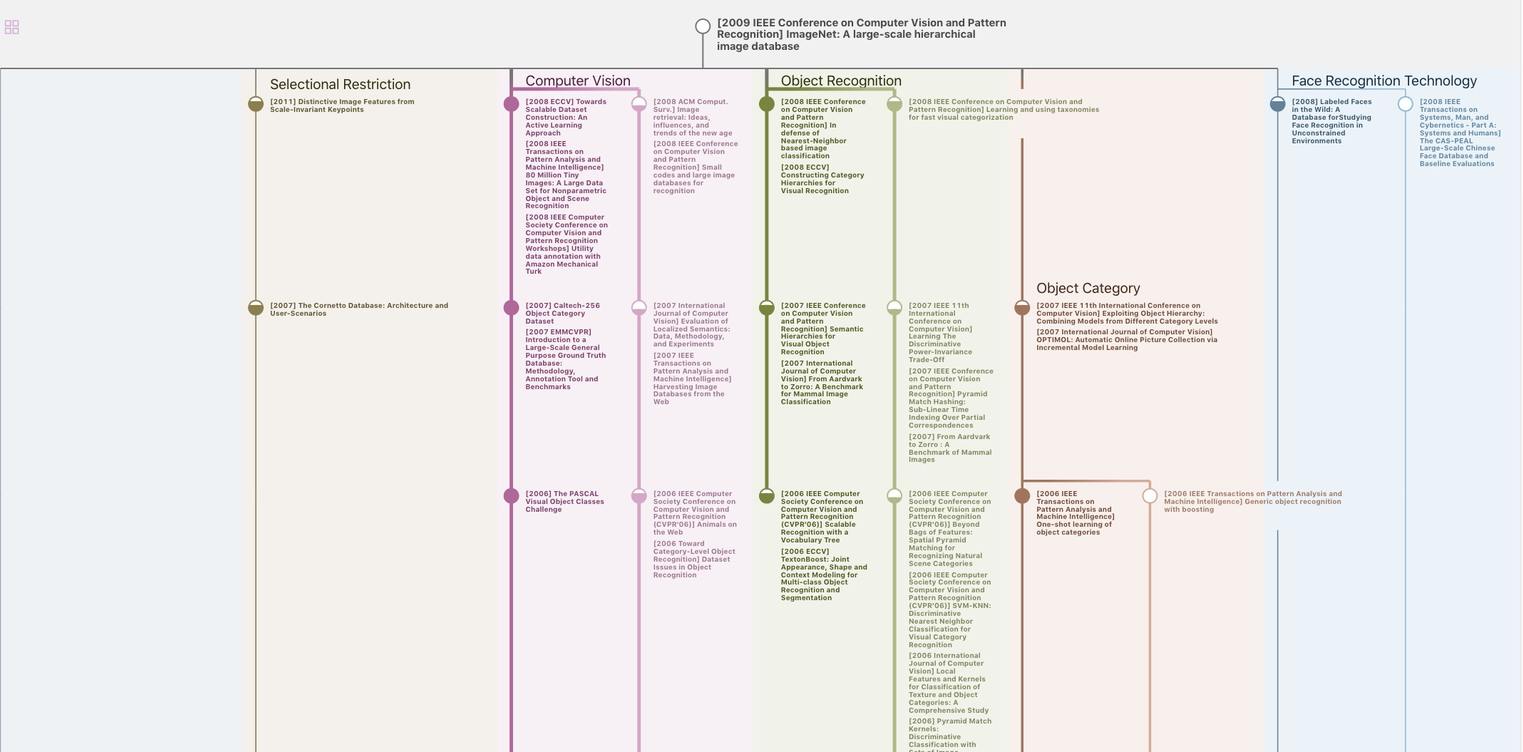
生成溯源树,研究论文发展脉络
Chat Paper
正在生成论文摘要