Combining YOLO and Deep Reinforcement Learning for Autonomous Driving in Public Roadworks Scenarios
Proceedings of the 14th International Conference on Agents and Artificial Intelligence(2022)
摘要
Autonomous driving is emerging as a useful practical application of Artificial Intelligence (AI) algorithms regarding both supervised learning and reinforcement learning methods. AI is a well-known solution for some autonomous driving problems but it is not yet established and fully researched for facing real world problems regarding specific situations human drivers face every day, such as temporary roadworks and temporary signs. This is the core motivation for the proposed framework in this project. YOLOv3-tiny is used for detecting roadworks signs in the path traveled by the vehicle. Deep Deterministic Policy Gradient (DDPG) is used for controlling the behavior of the vehicle when overtaking the working zones. Security and safety of the passengers and the surrounding environment are the main concern taken into account. YOLOv3-tiny achieved an 94.8% mAP and proved to be reliable in real-world applications. DDPG made the vehicle behave with success more than 50% of the episodes when testing, although still needs some improvements to be transported to the real-world for secure and safe driving.
更多查看译文
关键词
Deep Learning,YOLO,Reinforcement Learning,Deep Deterministic Policy Gradient,Autonomous Driving,Public Roadworks
AI 理解论文
溯源树
样例
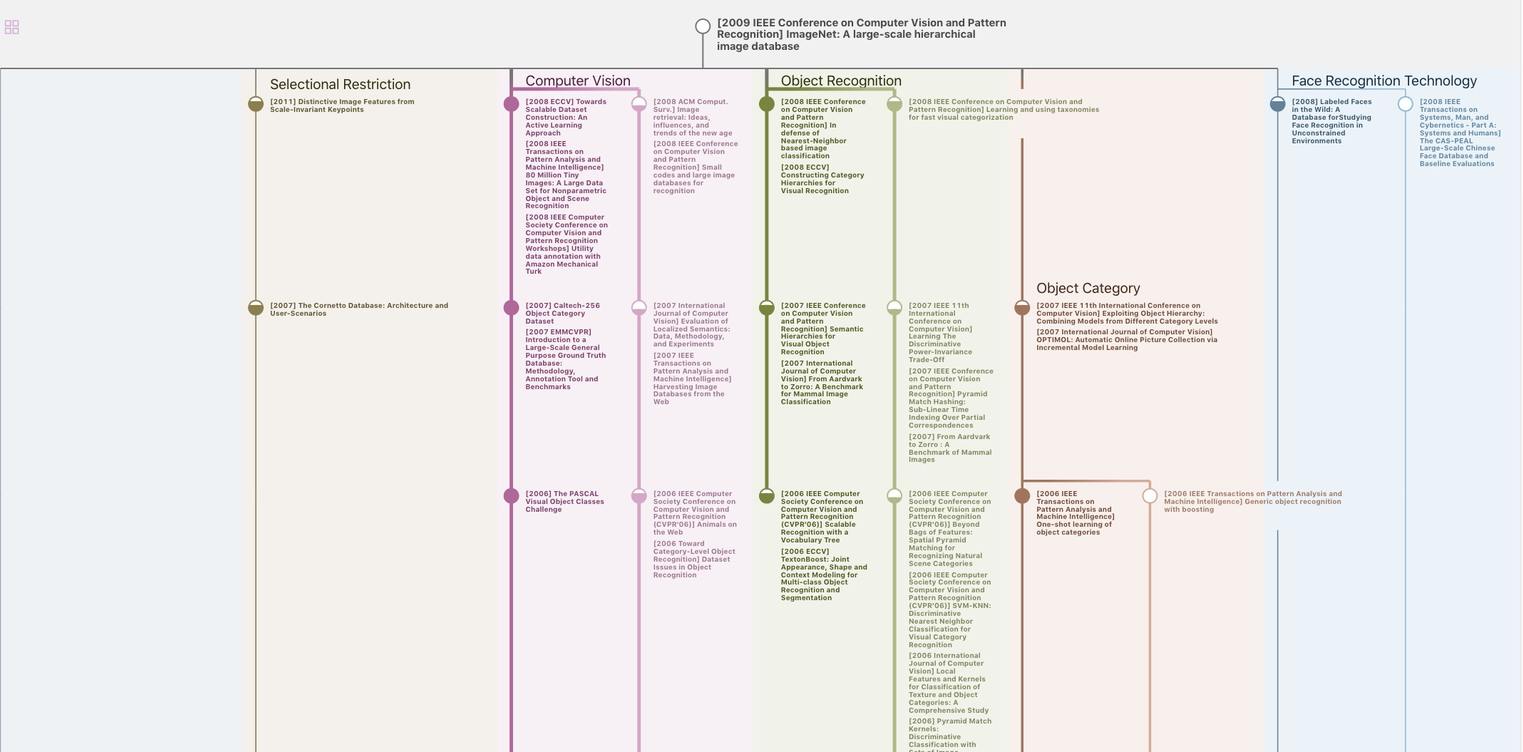
生成溯源树,研究论文发展脉络
Chat Paper
正在生成论文摘要