Diversity-accuracy Assessment of Multiple Classifier Systems for the Land Cover Classification of the Khumbu Region in the Himalayas
Journal of mountain science/Journal of Mountain Science(2022)
Abstract
Land cover classification of mountainous environments continues to be a challenging remote sensing problem, owing to landscape complexities exhibited by the region. This study explored a multiple classifier system(MCS) approach to the classification of mountain land cover for the Khumbu region in the Himalayas using Sentinel-2 images and a cloud-based model framework. The relationship between classification accuracy and MCS diversity was investigated, and the effects of different diversification and combination methods on MCS classification performance were comparatively assessed for this environment. We present ten MCS models that implement a homogeneous ensemble approach, using the high performing Random Forest(RF) algorithm as the selected classifier. The base classifiers of each MCS model were developed using different combinations of three diversity techniques:(1) distinct training sets,(2) Mean Decrease Accuracy feature selection, and(3) ‘One-vs-All’ problem reduction. The base classifier predictions of each RFMCS model were combined using:(1) majority vote,(2) weighted argmax, and(3) a meta RF classifier. All MCS models reported higher classification accuracies than the benchmark classifier(overall accuracy with 95% confidence interval: 87.33%±0.97%), with the highest performing model reporting an overall accuracy(±95%confidence interval)of 90.95%±0.84%. Our key findings include:(1) MCS is effective in mountainous environments prone to noise from landscape complexities,(2) problem reduction is indicated as a stronger method over feature selection in improving the diversity of the MCS,(3) although the MCS diversity and accuracy have a positive correlation, our results suggest this is a weak relationship for mountainous classifications, and(4)the selected diversity methods improve the discriminability of MCS against vegetation and forest classes in mountainous land cover classifications and exhibit a cumulative effect on MCS diversity for this context.
MoreTranslated text
Key words
Multiple classifier system,Ensemble diversity,Google Earth Engine,Land Cover Classification,Himalayas,Random Forest
AI Read Science
Must-Reading Tree
Example
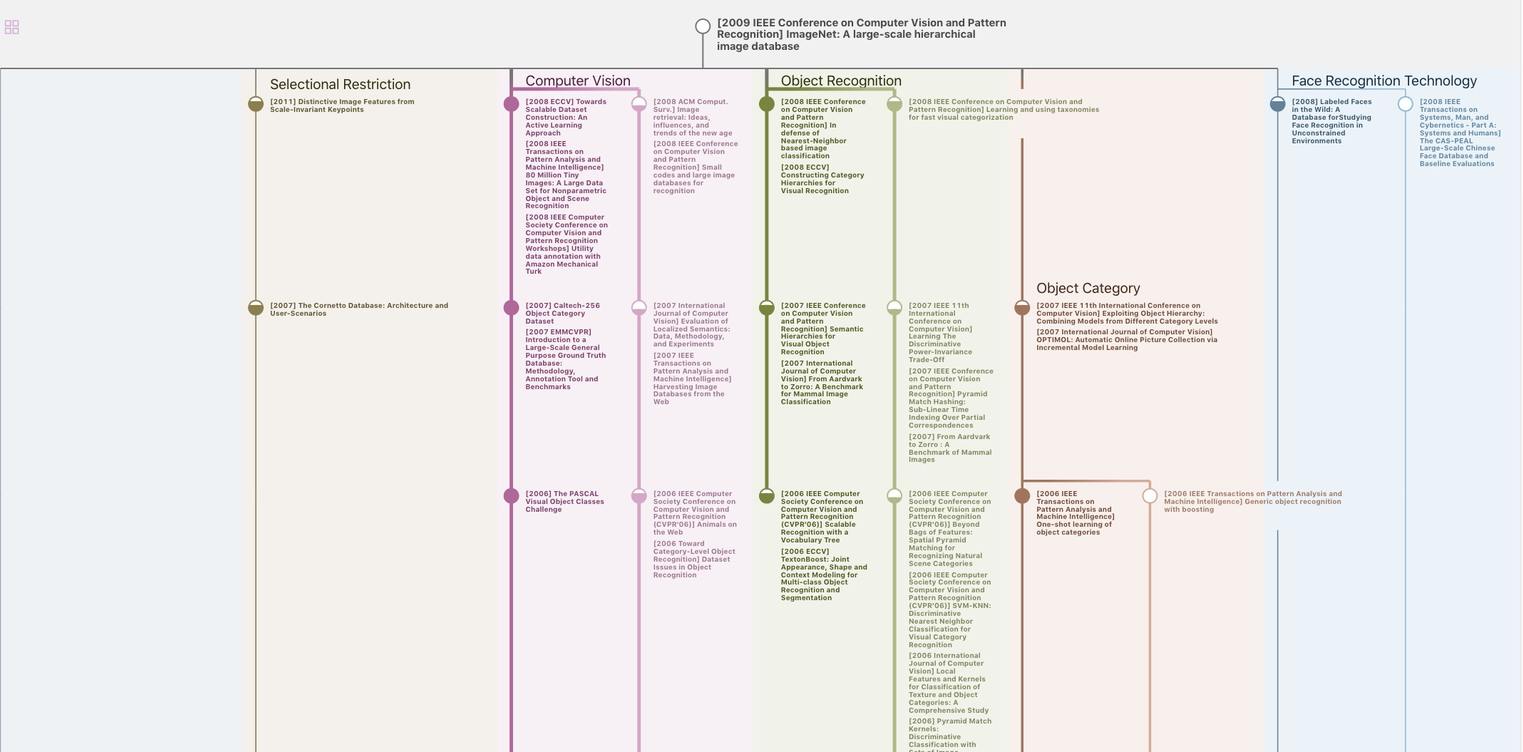
Generate MRT to find the research sequence of this paper
Chat Paper
Summary is being generated by the instructions you defined