Foreground-guided textural-focused person re-identification
Neurocomputing(2022)
摘要
Person re-identification (Re-ID) is challenging in computer vision. It is crucial to strengthen discriminative features and suppress irrelevant ones for high-performance. Existing Re-ID approaches have won significant progress by attention mechanisms or introducing key-point/part priors. However, these methods suffer the high cost of building models. Besides, potential feature false alarm problems may happen due to background interference and inaccurate priors. Moreover, they usually ignore the degradation of texture clues which are identity sensitive. In this paper, we propose the Foreground-Guided Textural-Focused Network (FTN) to address these problems. Specifically, our FTN is an end-to-end Re-ID framework, which consists of a Semantic Encoder (S-Enc), a Compact Foreground Attention (CFA) module, and a Texture-Focused Decoder (TF-Dec). First, based on CFA and 2D Gaussian kernel, a coarse foreground-guided learning strategy is developed to suppress the feature false alarms at the source. Its core idea lies in constructing foreground guidance, which forces S-Enc to pay more attention to person instance-level features. Second, the TF-Dec is designed as a lightweight reconstruction task. It is trained via a novel gradient loss, and further forces S-Enc to maintain texture-wise details. Our method is computationally efficient as TF-Dec is abandoned in the inference phase. Extensive experiments are conducted on three large-scale Re-ID datasets Market1501, CUHK03, MSMT17, and two other occluded datasets. The results indicate that FTN achieves superior performance against the state-of-the-art methods, e.g., Rank-1 of 96.2% and 59.0% on Market1501 and Occluded-Duke respectively.
更多查看译文
关键词
Person re-identification,Foreground guidance,Texture reconstruction
AI 理解论文
溯源树
样例
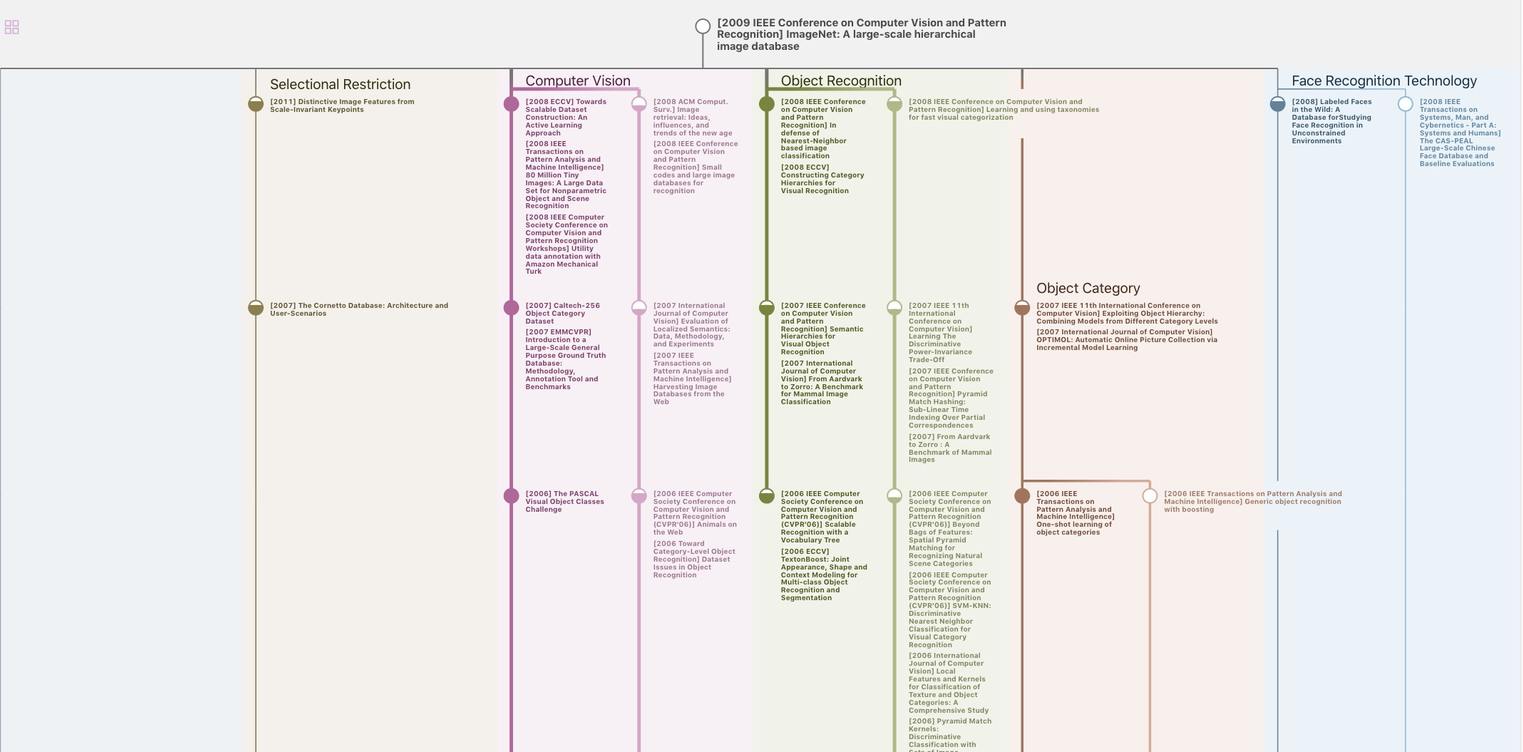
生成溯源树,研究论文发展脉络
Chat Paper
正在生成论文摘要