An Edge Computing-oriented Net Power Forecasting for PV-assisted Charging Station: Model Complexity and Forecasting Accuracy Trade-off
APPLIED ENERGY(2022)
摘要
The PV-assisted charging station (PVCS) aggregates the two key resources of electric vehicle (EV) charging load and photovoltaic (PV) system to maximize operation profit. In order to quantify the PVCS impact to grid and the renewable energy utilization by EV charging load, it is crucial to predict the PVCS net power and capture the most correlated factors for power variation. Given that the PVCS is located near the user side, the forecasting model complexity must be restricted to meet the online training demands on edge computing, apart from ensuring the prediction accuracy. A PVCS net power forecasting approach is proposed by simplifying model complexity from the entire cycle of training process, including the input data pruning, lightweight model training and hyperparameter optimization. Finally, a comprehensive analysis of the real data in PVCS shows that the most sensitivity factors affecting the net power of PVCS is time of use price, and the deep auto-encoded extreme learning machine (DA-ELM) can make a satisfactory compromise between prediction accuracy and model complexity for edge computing utilization. The forecasting model has outstanding prediction performance on Raspberry pi-based edge platform, which has enough significance for PVCS promotion.
更多查看译文
关键词
PV-assisted charging station (PVCS),Net power forecasting,Edge computing,Online training,Model complexity
AI 理解论文
溯源树
样例
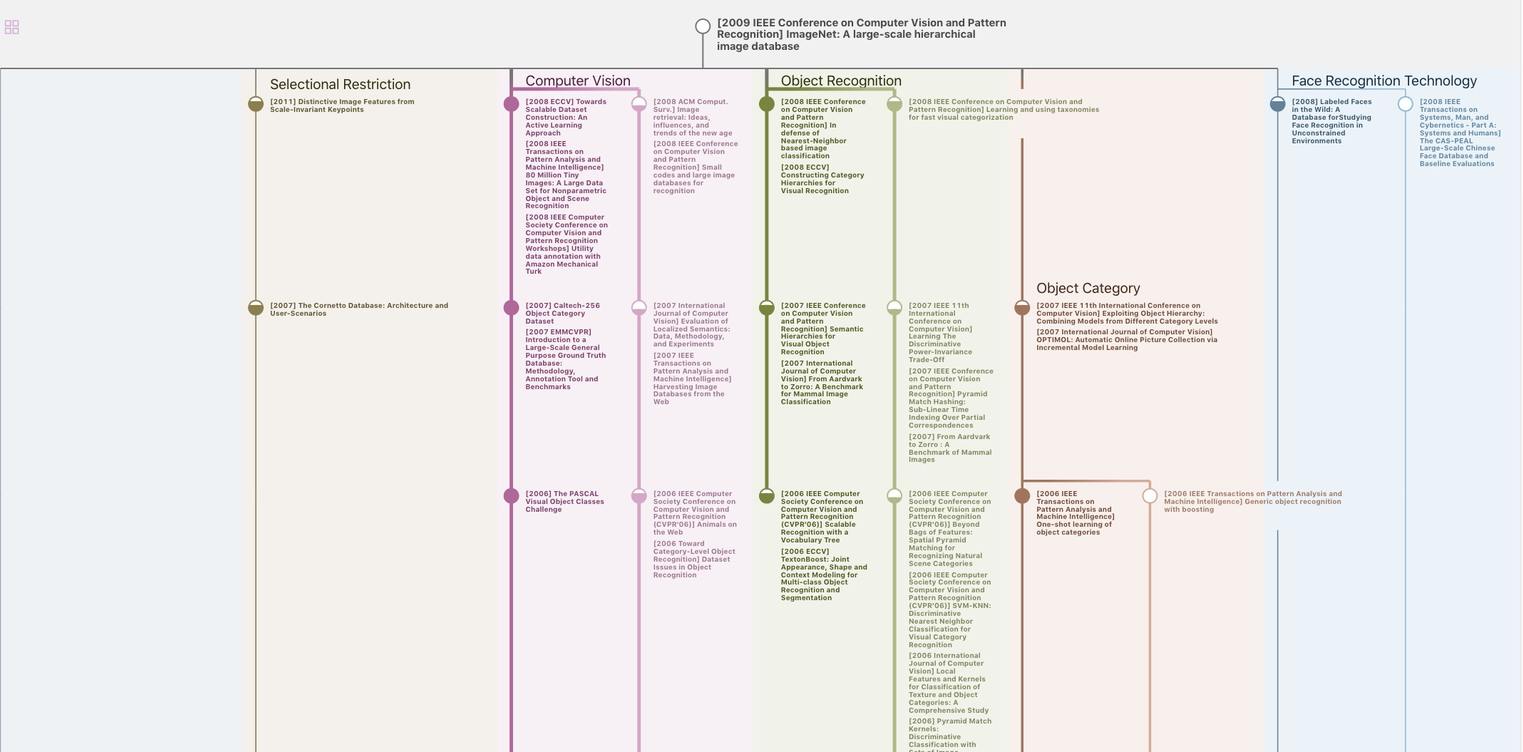
生成溯源树,研究论文发展脉络
Chat Paper
正在生成论文摘要