AI4SeaIce: Toward Solving Ambiguous SAR Textures in Convolutional Neural Networks for Automatic Sea Ice Concentration Charting
IEEE transactions on geoscience and remote sensing(2022)
摘要
Automatically producing Arctic sea ice charts from Sentinel-1 synthetic aperture radar (SAR) images is challenging for convolutional neural networks (CNNs) due to ambiguous backscattering signatures. The number of pixels viewed by the CNN model in the input image used to generate an output pixel, or the receptive field, is important to detect large features or physical objects such as sea ice and correctly classify them. In addition, a noise phenomenon is present in the Sentinel-1 ESA Instrument Processing Facility (IPF) v2.9 SAR data, particularly in subswath transitions, visible as long vertical lines and grained particles resembling small sea ice floes. To overcome these two challenges, we suggest adjusting the receptive field of the popular U-Net CNN architecture used for semantic segmentation. It is achieved by symmetrically adding additional blocks of convolutional, pooling and upsampling layers in the encoder and decoder of the U-Net, constituting an increase in the number of levels. This shows great improvements in the performance and in the homogeneity of predictions. Second, training models on SAR data noise-corrected with an enhanced technique has demonstrated a significant increase in model performance and enabled better predictions in uncertain regions. An eight-level U-Net trained on the alternative noise-corrected SAR data is presented to be capable of correctly predicting many ambiguous SAR signatures and increased the performance by 8.44% points compared with the regular U-Net trained on the ordinary ESA IPF v2.9 noise-corrected SAR data. This is the first installment of this multi-series installment of articles related to AI applied to sea ice (in short AI4SeaIce).
更多查看译文
关键词
Sea ice,Synthetic aperture radar,Radar polarimetry,Convolutional neural networks,Backscatter,Image resolution,Arctic,AI4Arctic,AI4EO,cryosphere,deep learning,receptive field,SAR noise correction,sea ice charting,synthetic aperture radar (SAR) data,U-Net
AI 理解论文
溯源树
样例
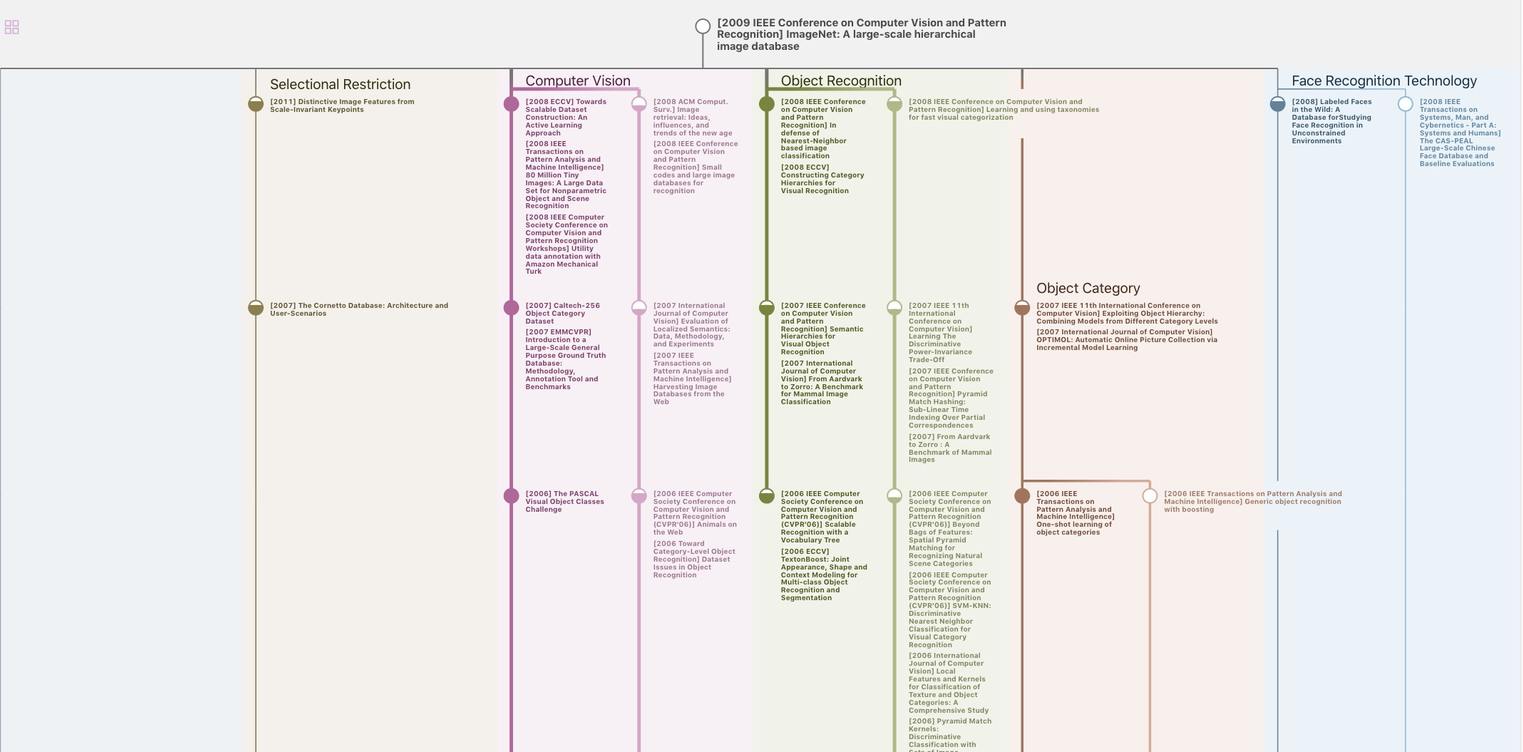
生成溯源树,研究论文发展脉络
Chat Paper
正在生成论文摘要