Ex Vivo Thrombus Magnetic Resonance Imaging Features and Patient Clinical Data Enable Prediction of Acute Ischemic Stroke Cause
Stroke: Vascular and Interventional Neurology(2022)
摘要
HomeStroke: Vascular and Interventional NeurologyVol. 2, No. 2Ex Vivo Thrombus Magnetic Resonance Imaging Features and Patient Clinical Data Enable Prediction of Acute Ischemic Stroke Cause Open AccessResearch ArticlePDF/EPUBAboutView PDFView EPUBSections ToolsAdd to favoritesDownload citationsTrack citations ShareShare onFacebookTwitterLinked InMendeleyRedditDiggEmail Jump toSupplementary MaterialsOpen AccessResearch ArticlePDF/EPUBEx Vivo Thrombus Magnetic Resonance Imaging Features and Patient Clinical Data Enable Prediction of Acute Ischemic Stroke Cause Spencer D. Christiansen, PhD, Junmin Liu, PhD, Maria Bres Bullrich, MD, Manas Sharma, MD, Sachin K. Pandey, MD, Melfort Boulton, MDPhD, Sebastian Fridman, MDMPH, Luciano A. Sposato, MDMBA and Maria Drangova, PhD Spencer D. ChristiansenSpencer D. Christiansen https://orcid.org/0000-0002-9497-6789 , Imaging Research Laboratories, , Robarts Research Institute, , London, , Ontario, , Canada, , Department of Medical Biophysics, , Western University, , London, , Ontario, , Canada, Search for more papers by this author , Junmin LiuJunmin Liu , Imaging Research Laboratories, , Robarts Research Institute, , London, , Ontario, , Canada, Search for more papers by this author , Maria Bres BullrichMaria Bres Bullrich , Department of Clinical Neurological Sciences, , Western University, , London, , Ontario, , Canada, Search for more papers by this author , Manas SharmaManas Sharma , Department of Medical Imaging, , Western University, , London, , Ontario, , Canada, Search for more papers by this author , Sachin K. PandeySachin K. Pandey , Department of Medical Imaging, , Western University, , London, , Ontario, , Canada, Search for more papers by this author , Melfort BoultonMelfort Boulton , Department of Clinical Neurological Sciences, , Western University, , London, , Ontario, , Canada, Search for more papers by this author , Sebastian FridmanSebastian Fridman , Department of Clinical Neurological Sciences, , Western University, , London, , Ontario, , Canada, Search for more papers by this author , Luciano A. SposatoLuciano A. Sposato , Department of Clinical Neurological Sciences, , Western University, , London, , Ontario, , Canada, Search for more papers by this author and Maria DrangovaMaria Drangova *Correspondence to: Maria Drangova, PhD, Imaging Research Laboratories, Robarts Research Institute, Western University, 1151 Richmond Street, London, Ontario N6A 2B7, Canada.E‐mail: E-mail Address: [email protected] , Imaging Research Laboratories, , Robarts Research Institute, , London, , Ontario, , Canada, , Department of Medical Biophysics, , Western University, , London, , Ontario, , Canada, Search for more papers by this author Originally published31 Jan 2022https://doi.org/10.1161/SVIN.121.000157Stroke: Vascular and Interventional Neurology. 2022;2:e000157Other version(s) of this articleYou are viewing the most recent version of this article. Previous versions: March 8, 2022: Previous Version of Record February 1, 2022: Ahead of Print AbstractDownload figureThe cause of ischemic stroke often remains elusive even after full stroke workup is completed. Cardioembolic mechanisms in particular are frequently presumed but challenging to definitively diagnose. Quantitative thrombus texture analysis is emerging as a powerful tool for stroke characterization, having shown the ability to predict response to stroke treatment,1 but its ability to predict stroke cause and complement machine learning models built from standard clinical features has not been studied.2, 3 The purpose of this study is to evaluate the ability of radiomics features extracted from quantitative magnetic resonance images of retrieved ischemic stroke thrombi (R2*(=1/T2*), quantitative susceptibility mapping, and fat fraction) to improve the accuracy of machine learning models built from clinical data for the prediction of cardioembolic stroke.MethodsInstitutional research ethics board approval was obtained for this study; data are available from the corresponding author on reasonable request.Patients with acute ischemic stroke with cardioembolic or large artery atherosclerosis causes determined using TOAST criteria and thrombi retrieved through endovascular therapy were consecutively enrolled into training (February 2016–November 2017; N=49) and validation (November 2019–March 2020; N=11) cohorts. Summary clinical details of each cohort are included in Supplemental Table SI (available from: ir.lib.uwo.ca/vascularpub/59). Patients or their substitute decision‐maker gave informed consent after the procedure was completed and ≥1 thrombi were retrieved. A dual‐echo‐train gradient echo sequence4 was acquired on the thrombi ex vivo with 0.94×0.94×1.0 mm3 resolution and a scan time of 5 minutes 33 seconds on a 3T clinical scanner. A balanced steady‐state gradient echo sequence (FIESTA‐C) with identical resolution was also acquired and used for thrombus segmentation (scan time: 2 minutes 47 seconds). R2*, quantitative susceptibility mapping, and fat fraction maps were generated from the multiecho gradient echo data using previously described methods.4 Random forest classifier models were built to differentiate between cardioembolic and large artery atherosclerosis stroke on a per‐thrombus basis using patient clinical data features available from the basic stroke workup and quantitative ex vivo thrombus magnetic resonance texture features extracted from R2*, quantitative susceptibility mapping, and fat fraction maps. Models were built in MATLAB (The MathWorks, Inc) using code modified from Vallières et al.5 Tested texture features are listed in Supplemental Table SII (available from: ir.lib.uwo.ca/vascularpub/59). Feature selection was performed on the training cohort using multivariate logistic regression for texture and univariate statistics for clinical features, respectively. Models were evaluated on the entire validation cohort, and area under the receiver operating characteristic curve (AUC), sensitivity, specificity, and accuracy for all thrombi within the cohort (n=18) were determined.ResultsWithin the training cohort, age, smoking, left atrial enlargement, left ventricular ejection fraction, cardiac wall motion abnormalities, and triglyceride levels differed between patients with cardioembolic and large artery atherosclerosis (all P<0.05; Supplemental Table SIII, ir.lib.uwo.ca/vascularpub/59) and were thus included in the clinical model. This model predicted cardioembolic mechanism in the validation cohort with an AUC of 0.80 (95% CI, 0.45–1), sensitivity of 80% (95% CI, 52%–100%), specificity of 100%, and accuracy of 91% (95% CI, 78%–100%). Selected thrombus imaging texture features were quantitative susceptibility mapping global skewness, gray‐level run‐length matrix run‐length variance, gray‐level size zone matrix gray‐level nonuniformity, and fat fraction global variance, and their inclusion increased model AUC, sensitivity, and accuracy to 0.89 (95% CI, 0.68–1), 88% (95% CI, 65%–100%), and 94% (95% CI, 84%–100%), respectively, while maintaining 100% specificity. A separate model built using only thrombus texture features produced an AUC, sensitivity, specificity, and accuracy of 0.63 (95% CI, 0.35–0.9), 75 (95% CI, 45%–100%), 60 (95% CI, 30%–90%), and 67% (95% CI, 45%–89%), respectively. A diagram displaying the texture feature extraction process for 2 representative thrombi and demonstrating the ability for imaging information to improve clinical model predictions is shown in Figure 1.Download figureDownload PowerPointFigure 1. Overview of model processing steps for representative cardioembolic (A) and large artery atherosclerosis (B) thrombi. Segmentations derived from FIESTA‐C images are applied to naturally coregistered quantitative susceptibility mapping (QSM) and fat fraction (FF) maps, which are binned before extraction of the histograms and matrices used to derive the 4 predictive texture features for model input. In (A), a patient with known atrial fibrillation did not undergo echocardiography and was missing multiple variables included in the clinical model, uncommon in this cohort but typical of clinical data in general, which resulted in a weak cardioembolic cause prediction that was greatly improved through the addition of thrombus texture information. In (B), all patient clinical features were available and the addition of thrombus texture information improved the model's confidence in the identification of a noncardioembolic cause. GLN indicates gray‐level nonuniformity; GLRLM, gray‐level run‐length matrix; GLSZM, gray‐level size zone matrix; LA, left atrial; LV, left ventricular; RLV, run‐length variance; and WM, wall motion.DiscussionThis study suggests that thrombus magnetic resonance imaging can improve the accuracy of clinical data for the prediction of cardioembolic stroke mechanisms. The combination of thrombus imaging texture and baseline clinical data features discriminated between stroke sources with exceptional accuracy.Recently, a large study by Kamel et al2 developed a machine learning model for stroke cause prediction using only clinical variables. Similar to our study, the model identified age, left atrial enlargement, ejection fraction, and smoking history as important predictors of cardioembolic cause, and yielded an AUC of 0.85 akin to our clinical‐only model. Here, the addition of imaging features improved the performance of the clinical model, suggesting that thrombus imaging information can complement clinical data for this task. This study is limited by its small sample size and ex vivo design; only patients who underwent successful endovascular therapy could be included and alterations to thrombi during thrombolysis, retrieval, or storage could have affected the imaging values. The study is also limited by the lack of a histological validation of imaging features and the inclusion of multiple cardioembolic stroke subtypes in the cardioembolic group. The generalizability of the model to thrombi imaged in vivo remains to be evaluated. Nonetheless, this proof‐of‐concept study suggests that thrombus magnetic resonance texture features can improve the accuracy of clinical features alone for predicting cardioembolic cause among patients with acute ischemic stroke.FundingThis work was supported by Canadian Institutes of Health Research grant number CIHR #PJT 153411.DisclosuresS.D.C., J.L., M.B.B., M.S., S.K.P., M.B., S.F., and M.D. report no disclosures relevant to the article. L.A.S. reports speaker honoraria from Boehringer Ingelheim, Pfizer, Gore, and Bayer, and research/quality improvement grants from Boehringer Ingelheim and Bayer. L.A.S. is a member of the editorial board of Neurology, Stroke, and World Stroke Academy.AcknowledgmentsThe authors wish to thank Justina Diaz Legaspe for recruiting and consenting participants; Ada Manini, Leanne Sperlich, Jon Collier, Sarah Sitts, Barb Lehrbass, Andrew Kernohan, Elizabeth Cesarin, and Jill Uitvlugt for storage of thrombi and facilitating sample transfer; all interventional neuroradiologists at London Health Sciences Centre for thrombus retrieval; and Trevor Wade for implementation of the pulse sequence.Footnotes*Correspondence to: Maria Drangova, PhD, Imaging Research Laboratories, Robarts Research Institute, Western University, 1151 Richmond Street, London, Ontario N6A 2B7, Canada.E‐mail: [email protected]ca[Correction added on 13th May 2022, after online publication: The copyright line is changed].References1 Hofmeister J, Bernava G, Rosi A, Vargas MI, Carrera E, Montet X, Burgermeister S, Poletti PA, Platon A, Lovblad KO. Clot‐based radiomics predict a mechanical thrombectomy strategy for successful recanalization in acute ischemic stroke. Stroke. 2020; 51:2488–2494.LinkGoogle Scholar2 Kamel H, Navi BB, Parikh NS, Merkler AE, Okin PM, Devereux RB, Weinsaft JW, Kim J, Cheung JW, Kim LK. Machine learning prediction of stroke mechanism in embolic strokes of undetermined source. Stroke. 2020; 51:e203–e210.LinkGoogle Scholar3 Guan W, Ko D, Khurshid S, Trisini Lipsanopoulos AT, Ashburner JM, Harrington LX, Rost NS, Atlas SJ, Singer DE, McManus DD. Automated electronic phenotyping of cardioembolic stroke. Stroke. 2021; 52:181–189.LinkGoogle Scholar4 Liu J, Christiansen SD, Drangova M. Single multi‐echo GRE acquisition with short and long echo spacing for simultaneous quantitative mapping of fat fraction, B0 inhomogeneity, and susceptibility. NeuroImage. 2018; 172:703–717.Google Scholar5 Vallières M, Freeman CR, Skamene SR, El Naqa I. A radiomics model from joint FDG‐PET and MRI texture features for the prediction of lung metastases in soft‐tissue sarcomas of the extremities. Phys Med Biol. 2015; 60:5471–5496.Google Scholar Previous Back to top Next FiguresReferencesRelatedDetails March 2022Vol 2, Issue 2Article InformationMetrics © 2022 The Authors. Published on behalf of the American Heart Association, Inc., and the Society of Vascular and Interventional Neurology by Wiley Periodicals LLC.This is an open access article under the terms of the Creative Commons Attribution‐NonCommercial License, which permits use, distribution and reproduction in any medium, provided the original work is properly cited and is not used for commercial purposes.https://doi.org/10.1161/SVIN.121.000157 Manuscript receivedAugust 11, 2021Manuscript acceptedDecember 29, 2021Originally publishedJanuary 31, 2022 Keywordsmagnetic resonance imagingstrokethrombosisischemic strokemachine learningthromboembolismischemiahumansPDF download SubjectsEtiologyIschemic StrokeMachine Learning and Artificial IntelligenceMagnetic Resonance Imaging (MRI)
更多查看译文
关键词
humans,ischemia,ischemic stroke,machine learning,magnetic resonance imaging,stroke
AI 理解论文
溯源树
样例
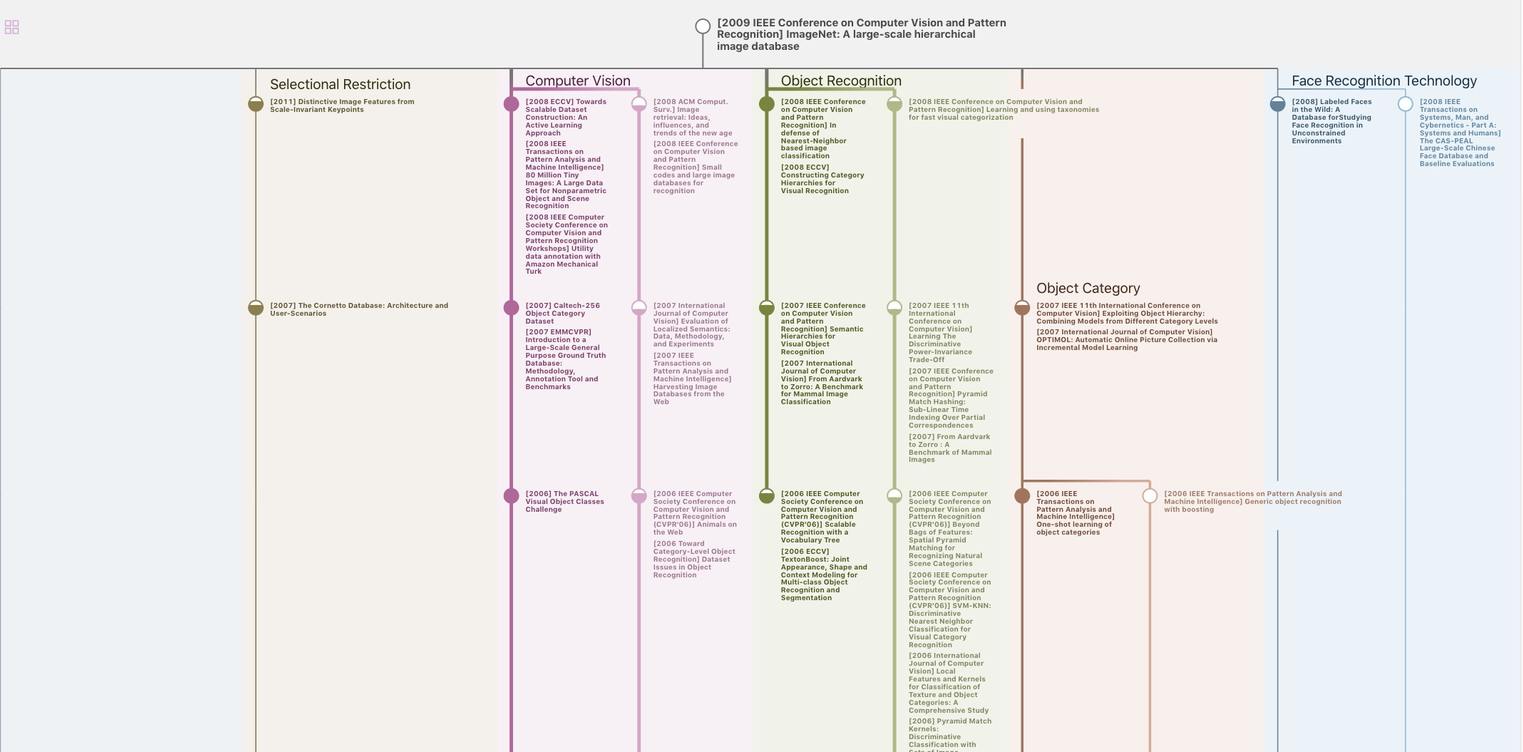
生成溯源树,研究论文发展脉络
Chat Paper
正在生成论文摘要