Improving Accuracy in Predicting City-Level Construction Cost Indices by Combining Linear ARIMA and Nonlinear ANNs
JOURNAL OF MANAGEMENT IN ENGINEERING(2022)
摘要
Accurate cost forecasting in budget planning and contract bidding is crucial for the success of construction projects. Linear models such as the autoregressive integrated moving average (ARIMA) and nonlinear models such as the artificial neural network (ANN) have been adopted in the literature for forecasting construction costs. However, both linear and nonlinear models are subject to some limitations derived from their modeling structure and assumptions. This study proposes a hybrid ARIMA-ANN model for forecasting construction costs and explores whether the hybrid ARIMA-ANN model can provide more accurate forecasts than an individual ARIMA or ANN. The national and city-level construction cost indices (CCIs) are forecasted for three forecasting horizons (short-term, mid-term, and long-term) using three forecasting models: (1) linear ARIMA, (2) nonlinear ANNs, and (3) the hybrid ARIMA-ANN model. Out-of-sample forecasting exercise reveals that the hybrid model combining the distinctive features of both ARIMA and ANNs performs better than individual models in most forecasting cases, especially for longer-term forecasting horizons. The findings can help project planners, cost engineers, and decision makers prepare for more accurate budgets and bids for diverse construction projects in different locations.
更多查看译文
关键词
Hybrid model,Out-of-sample forecasting,City-level Engineering News-Record (ENR) construction cost index (CCI),US cities,Autoregressive integrated moving average (ARIMA) model,Artificial neural network (ANN)
AI 理解论文
溯源树
样例
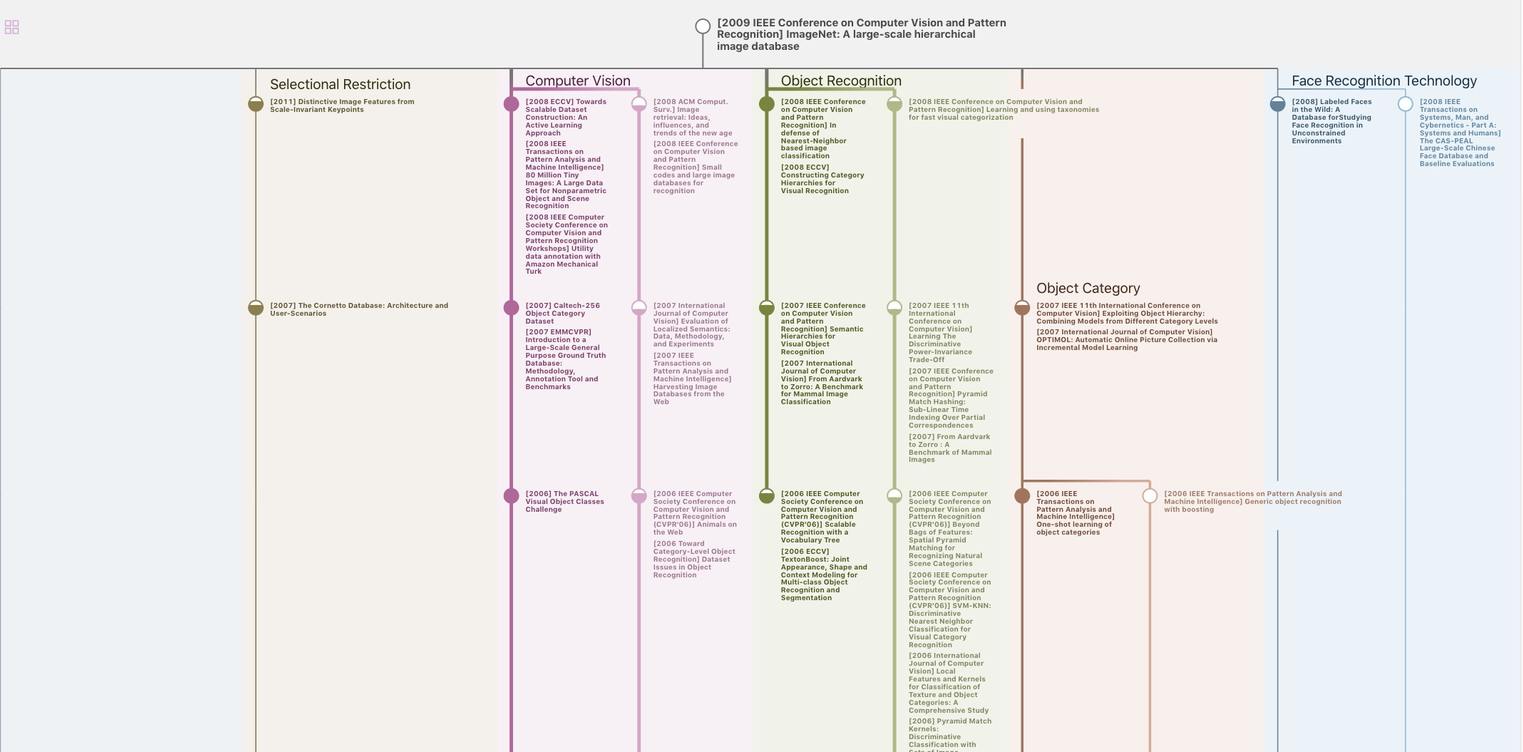
生成溯源树,研究论文发展脉络
Chat Paper
正在生成论文摘要