Deep network for retinal disease classification based on limited clinical OCT angiography datasets (Conference Presentation)
https://doi.org/10.1117/12.2291431(2018)
摘要
Optical coherence tomography angiography (OCT-A) is a novel non-invasive imaging technique that provide the visualization of retinal microvasculature. However, the quantification and evaluation of OCT-A are still a challenge for the diagnosis for ophthalmology. Deep convolutional neural network (CNN) architectures were initially designed for the task of natural image classification, delivering promising precision in computer vision tasks and recent research has applied deep CNN to biomedical image processing tasks and produces impressive outcomes. However, so far, there is no report relating to the application of large deep neural networks on a small annotated OCT-A dataset. We collected and annotated OCT-A datasets that contain diabetic retinopathy (DR), uveitis, dry age-related macular degeneration (AMD) patients, and normal cases. We propose a transfer learning CNN model for automated disease classification using clinical OCT-A images. The CNN model is pre-trained on the ImageNet dataset and fine-tuned the top feedforward layers of the model to fit the classification task during the training process. The proposed approach can offer a real-time evaluation and discrimination of retinal pathologies with a depth-encoded OCT-A projected image. Our results show great accuracy of transfer learning CNN model on the classification task with the limited dataset. The CNN model with the pre-trained weights has better performance in comparison with an SVM using HOG feature approach.
更多查看译文
关键词
retinal disease classification,deep network,angiography
AI 理解论文
溯源树
样例
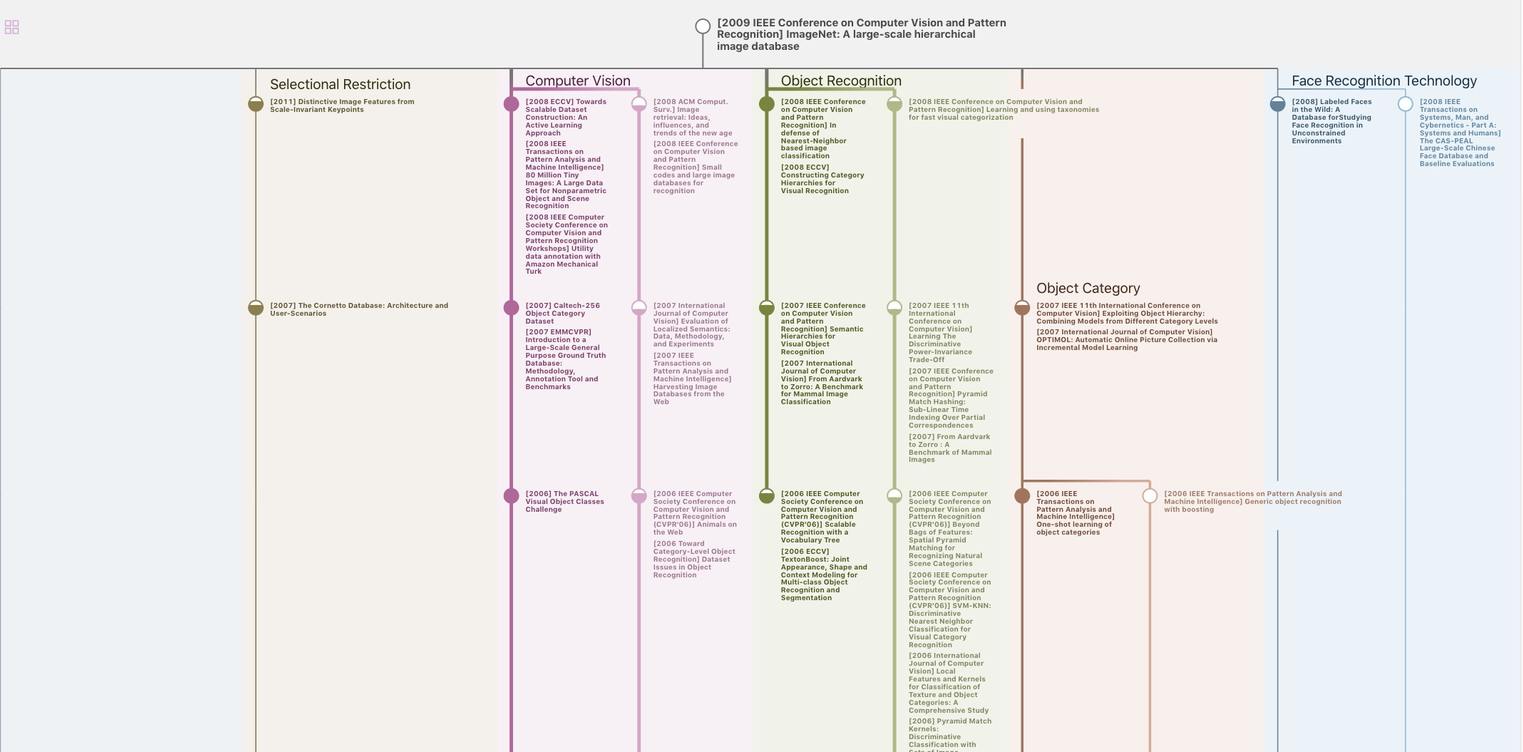
生成溯源树,研究论文发展脉络
Chat Paper
正在生成论文摘要