Stochastic Home Energy Management System Via Approximate Dynamic Programming
IET energy systems integration(2020)
摘要
This study proposes an approximate dynamic programming (ADP) method for a stochastic home energy management system (HEMS) that aims to minimise the electricity cost and discomfort of a household under uncertainties. The study focuses on a HEMS that optimally schedules heating, ventilation, and air conditioning, water heater, and electric vehicle, while accounting for uncertainties in outside temperature, hot water usage, and non-controllable net load. The authors approach the ADP-based HEMS via an effective combination of Sobol sampling backward induction and a K-D tree nearest neighbour techniques for the value function approximation. A subset of possible states is sampled and used to create an approximation of the value of being in aggregated states. They compare the ADP approach with other prevailing HEMS methods, including dynamic programming (DP) and mixed-integer linear programming (MILP), in a model predictive control framework. Simulation results show that the proposed ADP approach can yield near-optimal appliance schedules under uncertainties when finely discretised. Merits and drawbacks of the proposed ADP method in comparison with DP and MILP are also revealed.
更多查看译文
关键词
linear programming,demand side management,integer programming,predictive control,function approximation,dynamic programming,energy management systems,domestic appliances,nearest neighbour methods,trees (mathematics),HVAC,electric vehicles,mixed-integer linear programming,ADP approach,near-optimal appliance schedules,ADP method,stochastic home energy management system,approximate dynamic programming method,electricity cost,optimal scheduling,water heater,electric vehicle,hot water usage,noncontrollable net load,ADP-based HEMS,K-D tree nearest neighbour techniques,value function approximation,HEMS methods,heating-ventilation-air conditioning,outside temperature,Sobol sampling backward induction,model predictive control framework
AI 理解论文
溯源树
样例
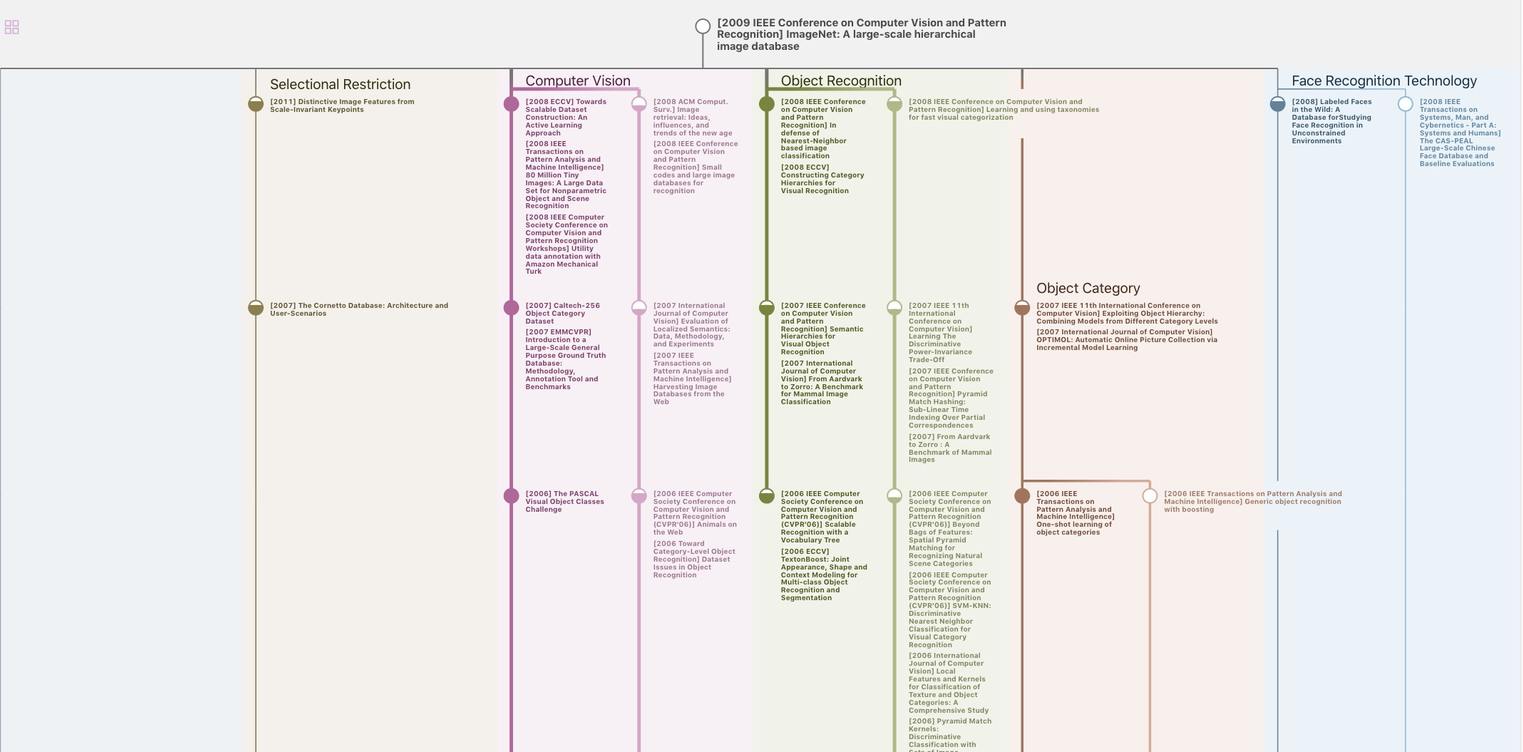
生成溯源树,研究论文发展脉络
Chat Paper
正在生成论文摘要