Dynamic boundary‐insensitive loss for MR medical image segmentation
Medical Physics(2021)
摘要
A deep learning method has achieved great success in MR medical image segmentation. One challenge in applying deep learning segmentation models to clinical practice is their poor generalization mainly due to limited labeled training samples, inter-site heterogeneity of different datasets, and ambiguous boundary definition, etc. The objective of this work is to develop a dynamic boundary-insensitive (DBI) loss to address this poor generalization caused by an uncertain boundary.The DBI loss is designed to assign higher penalties to misclassified voxels farther from the boundaries in each training iteration to reduce the sensitivity of the segmentation model to the uncertain boundary. The weighting factor of the DBI loss can be adjusted adaptively without any manual setting and adjustment. Extensive experiments were conducted to verify the performance of our DBI loss and its variant, DiceDBI, on four heterogeneous prostate MRI datasets for prostate zonal segmentation and whole prostate segmentation.Experimental results show that our DBI loss, when combined with Dice loss, outperforms all competing loss functions in dice similarity coefficient (DSC) and improves the segmentation performance across all datasets consistently, especially on unseen datasets and when segmenting small or narrow targets.The proposed DiceDBI loss will be valuable for enhancement of the generalization performance of the segmentation model.
更多查看译文
关键词
image segmentation,magnetic resonance,boundary‐insensitive
AI 理解论文
溯源树
样例
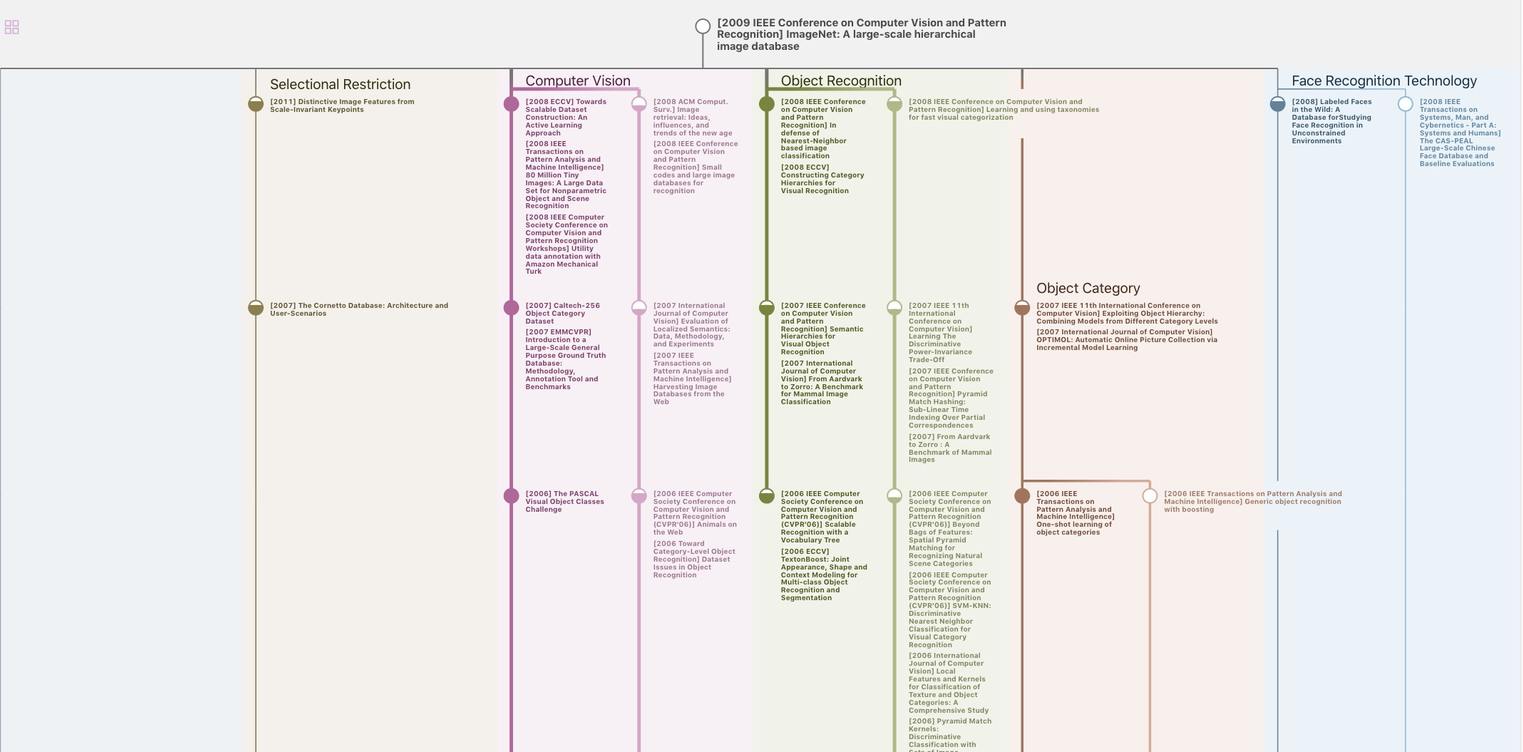
生成溯源树,研究论文发展脉络
Chat Paper
正在生成论文摘要