Accelerated Derivative-Free Deep Reinforcement Learning for Large-Scale Grid Emergency Voltage Control
IEEE Transactions on Power Systems(2022)
摘要
Load shedding has been one of the most widely used and effective emergency control approaches against voltage instability. With increased uncertainties and rapidly changing operational conditions in power systems, existing methods have outstanding issues in terms of either speed, adaptiveness, or scalability. Deep reinforcement learning (DRL) was regarded and adopted as a promising approach for fast and adaptive grid stability control in recent years. However, existing DRL algorithms show two outstanding issues when being applied to power system control problems: 1) computational inefficiency that requires extensive training and tuning time; and 2) poor scalability making it difficult to scale to high dimensional control problems. To overcome these issues, an novel derivative-free DRL algorithm named PARS was developed and tailored for power system voltage stability control via load shedding. The method was tested on both the IEEE 39-bus and IEEE 300-bus systems, and the latter is by far the largest scale for such a study. Test results show that, compared to other methods including model-predictive control (MPC) and proximal policy optimization(PPO) methods, PARS shows better computational efficiency (faster convergence), more robustness in learning, excellent scalability and generalization capability.
更多查看译文
关键词
Deep reinforcement learning,voltage stability,load shedding,augmented random search
AI 理解论文
溯源树
样例
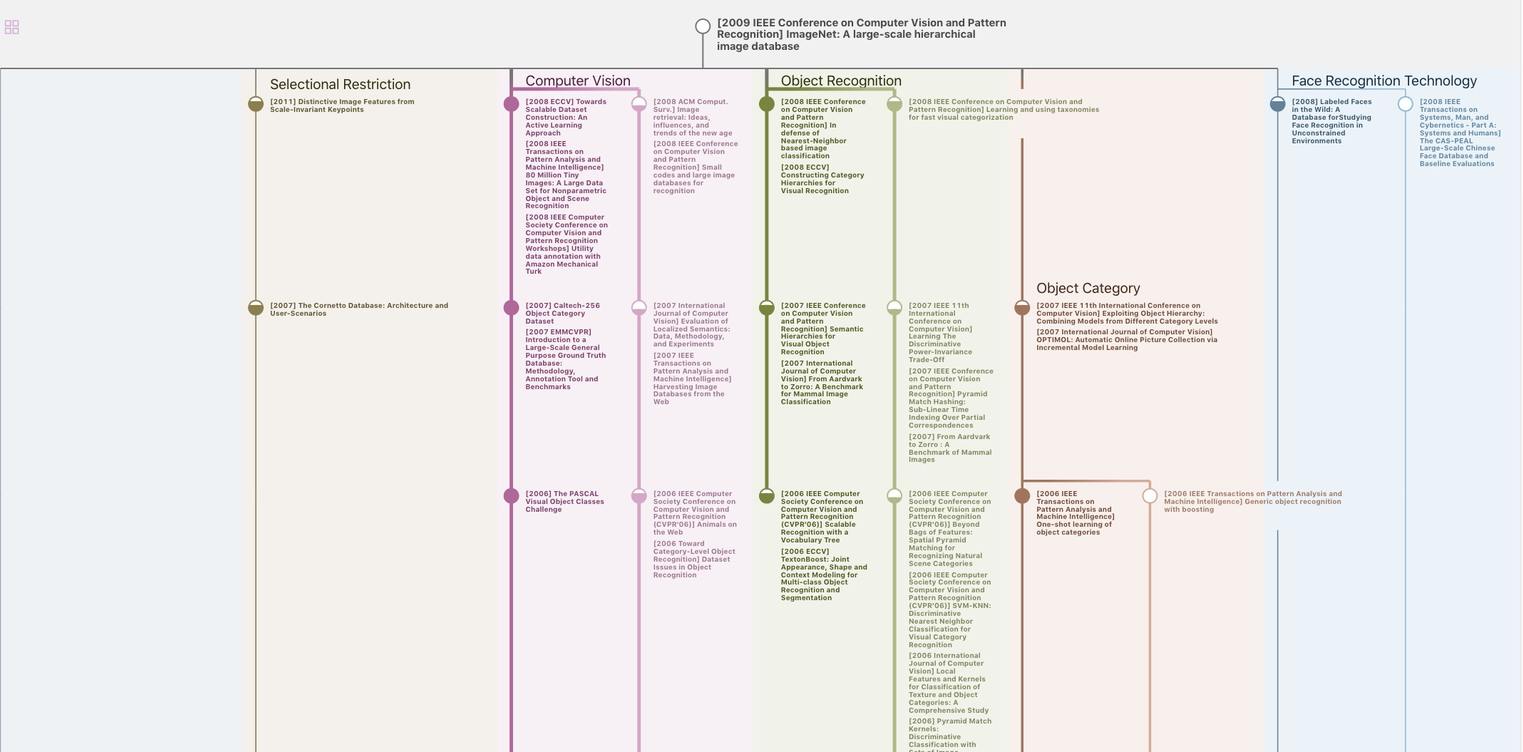
生成溯源树,研究论文发展脉络
Chat Paper
正在生成论文摘要