Optimal quantum dataset for learning a unitary transformation
PHYSICAL REVIEW APPLIED(2023)
摘要
Unitary transformations formulate the time evolution of quantum states. How to learn a unitary transformation efficiently is a fundamental problem in quantum machine learning. The most natural and leading strategy is to train a quantum machine learning model based on a quantum dataset. Although the presence of more training data results in better models, using too much data reduces the efficiency of training. In this work, we solve the problem on the minimum size of sufficient quantum datasets for learning a unitary transformation exactly, which reveals the power and limitation of quantum data. First, we prove that the minimum size of a dataset with pure states is $2^n$ for learning an $n$-qubit unitary transformation. To fully explore the capability of quantum data, we introduce a practical quantum dataset consisting of $n+1$ elementary tensor product states that are sufficient for exact training. The main idea is to simplify the structure utilizing decoupling, which leads to an exponential improvement in the size of the datasets with pure states. Furthermore, we show that the size of the quantum dataset with mixed states can be reduced to a constant, which yields an optimal quantum dataset for learning a unitary. We showcase the applications of our results in oracle compiling and Hamiltonian simulation. Notably, to accurately simulate a 3-qubit one-dimensional nearest-neighbor Heisenberg model, our circuit only uses $96$ elementary quantum gates, which is significantly less than $4080$ gates in the circuit constructed by the Trotter-Suzuki product formula.
更多查看译文
关键词
optimal quantum dataset,learning
AI 理解论文
溯源树
样例
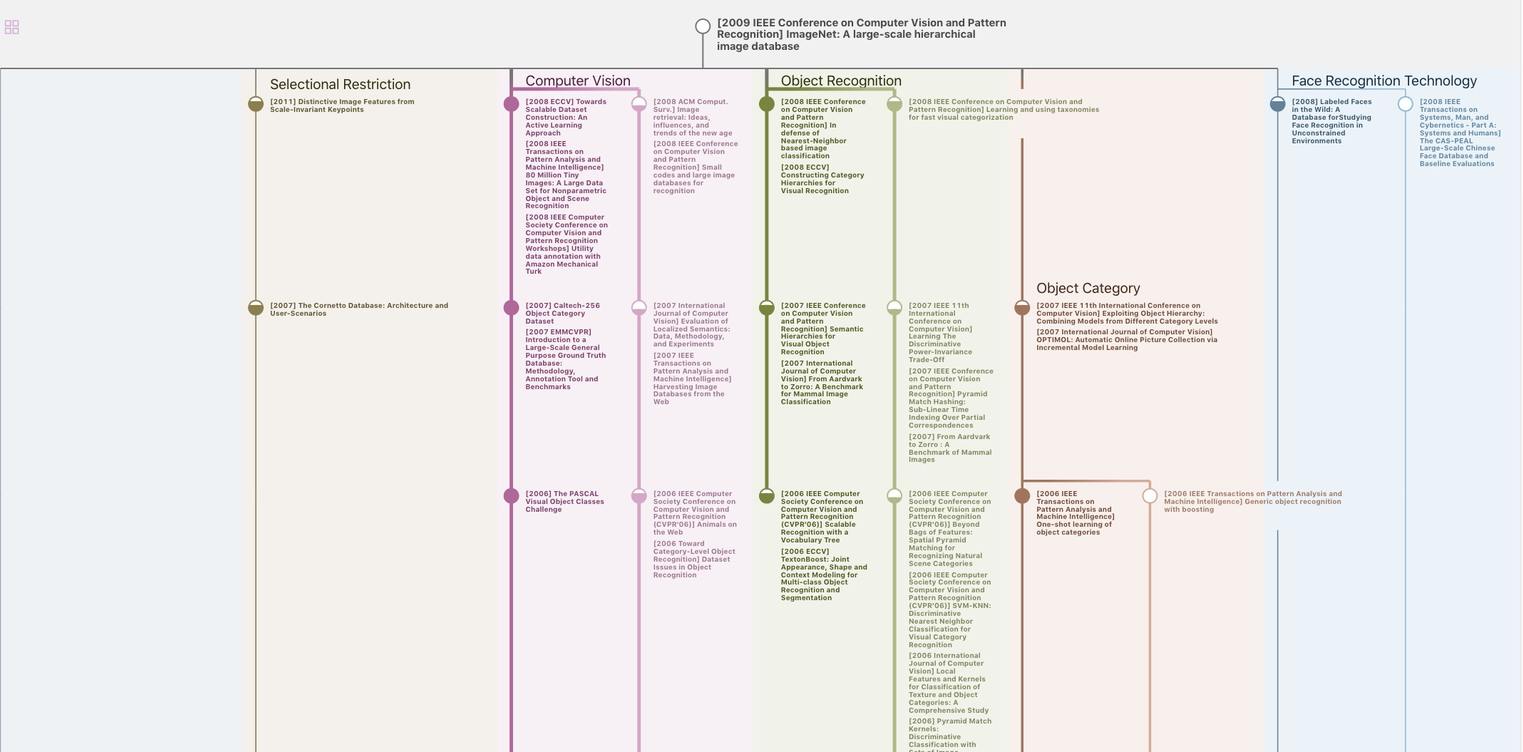
生成溯源树,研究论文发展脉络
Chat Paper
正在生成论文摘要