A matter of trust: Learning lessons about causality will make qAOPs credible.
Computational toxicology (Amsterdam, Netherlands)(2022)
摘要
Toxicology in the 21st Century has seen a shift from chemical risk assessment based on traditional animal tests, identifying apical endpoints and doses that are "safe", to the prospect of Next Generation Risk Assessment based on non-animal methods. Increasingly, large and high throughput datasets are being generated and exploited to develop computational models. This is accompanied by an increased use of machine learning approaches in the model building process. A potential problem, however, is that such models, while robust and predictive, may still lack credibility from the perspective of the end-user. In this commentary, we argue that the science of causal inference and reasoning, as proposed by Judea Pearl, will facilitate the development, use and acceptance of quantitative AOP models. Our hope is that by importing established concepts of causality from outside the field of toxicology, we can be "constructively disruptive" to the current toxicological paradigm, using the "Causal Revolution" to bring about a "Toxicological Revolution" more rapidly.
更多查看译文
关键词
Adverse Outcome Pathway,Causality,Model credibility,Next Generation Risk Assessment,qAOP
AI 理解论文
溯源树
样例
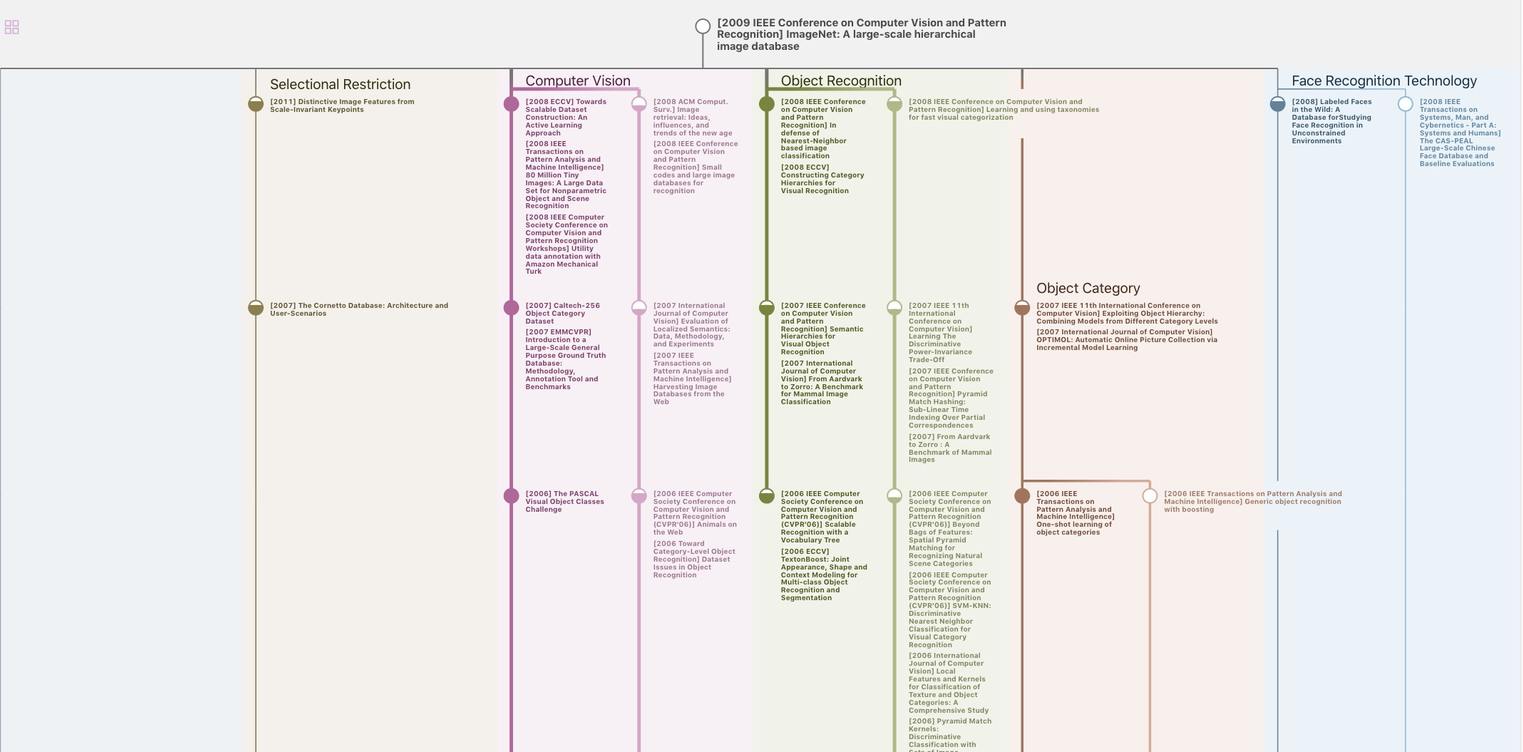
生成溯源树,研究论文发展脉络
Chat Paper
正在生成论文摘要