Efficient Hardware Trojan Detection Using Generic Feature Extraction and Weighted Ensemble Method
springer
摘要
Usage of smart devices has tremendously grown with the advent of Internet of Things (IoT). Like software, Integrated Circuit (IC) being the core component of hardware is also highly un-trusted. Outsourcing of ICs to off-shore foundries makes Hardware threats inevitable in IC design. Hardware threats are of five types, namely Intellectual Property (IP) piracy, IC over-building, counterfeiting, reverse engineering, and Hardware Trojan [2]. One of the most sought-after hardware security breaches is Hardware Trojan insertion. Many methods have been proposed in the last decade for Hardware Trojan detection. Recently, machine learning techniques are used to perform efficient Hardware Trojan detection. Prevailing machine learning approaches based on circuit learning achieve good performance but face some critical challenges. The most pertinent challenges being the complex feature extraction, time-consuming training process, and compromised performance metrics due to high data imbalance in the training dataset. The proposed work aims to overcome the aforementioned challenges. The work proposes a voting ensemble method, which detects Hardware Trojans from the gate-level netlist. Firstly, 15 generic features are extracted from the circuit netlist, which leverages the rich circuit knowledge present in synthesis reports. An ensemble of Random Forest Classifiers is trained and soft voting is performed to get the final prediction. The Random Forest Classifiers are class-weighted and are so configured to handle the effect of data imbalance. Finally, testing of the model is performed using Leave-One-Out Cross Validation. Experimental results on Trust-Hub circuits show that the proposed methodology achieves 99.73% accuracy, 98.25% recall, 96.81% precision, 97.44% F-measure, and 99.97% ROC-AUC score on an average of 16 circuits under test (CUT).
更多查看译文
关键词
Hardware trojans, Random forest classifier, Weighted ensemble method, Cross validation, Circuit under test
AI 理解论文
溯源树
样例
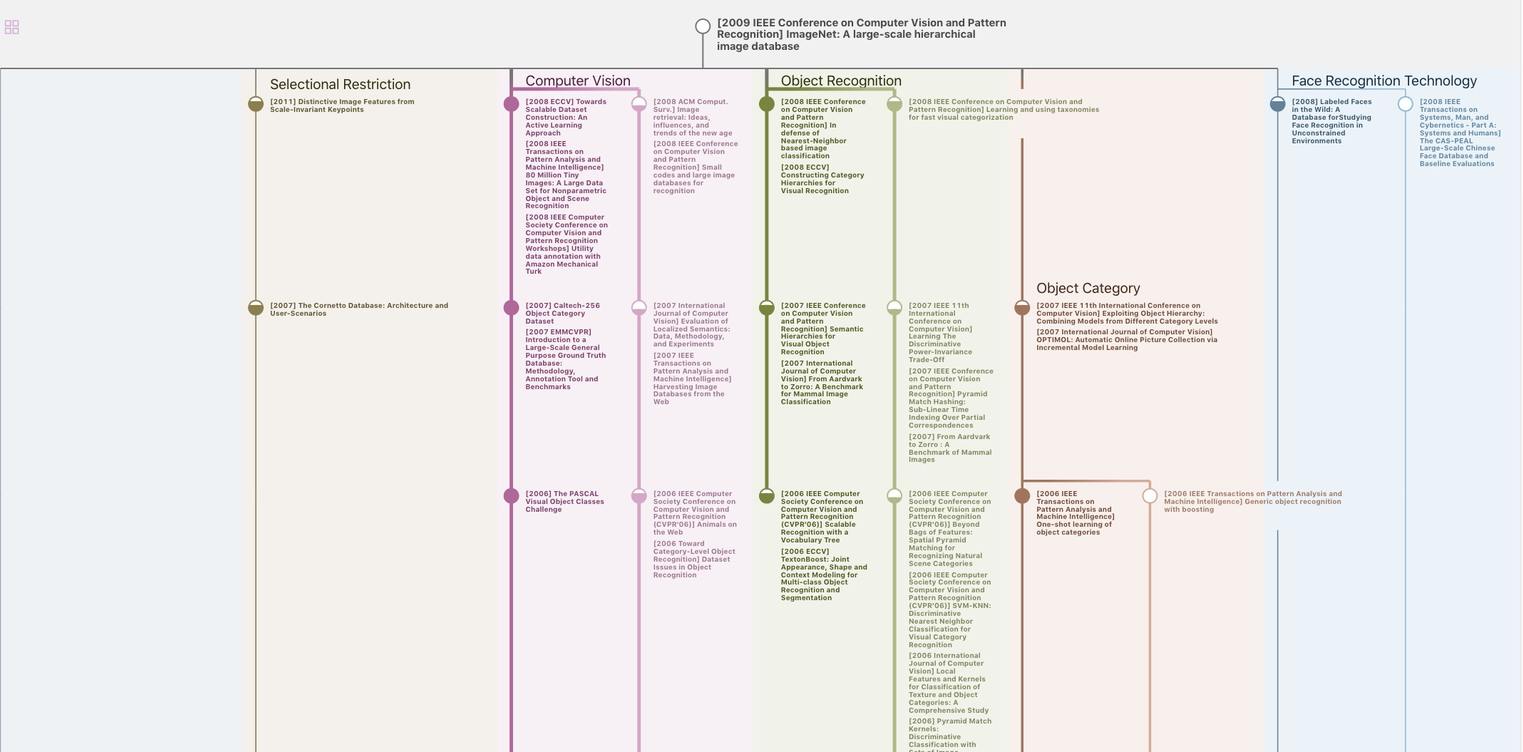
生成溯源树,研究论文发展脉络
Chat Paper
正在生成论文摘要