Deep Learning-based Phase Prediction of High-Entropy Alloys
IOP Conference Series Materials Science and Engineering(2020)
摘要
High-entropy alloys (HEAs) offer a new approach to the design of superior metallic materials, wherein alloys are based on multiple principal elements rather than just one. Deep Neural Networks (DNNs), machine learning tools that are efficiently used for prediction purposes, are transforming fields, from speech recognition to computational medicine. In this study, we extend DNN applications to the field phase prediction of high-entropy alloys. Using the built-in capabilities in TensorFlow and Keras, we train DNNs with different layers and numbers of neurons, achieving a 90% prediction accuracy. The DDN prediction model is examined in detail with different datasets to verify model robustness. Due to the high cost of HEAs and in order to save time, it is important to predict phases in order to design alloy composition. Through this study, we show trained DNNs to be a viable tool for predicting the phases of high-entropy alloys, where 90% phase prediction accuracy was achieved in this work.
更多查看译文
关键词
High-Entropy Alloys,Materials Discovery
AI 理解论文
溯源树
样例
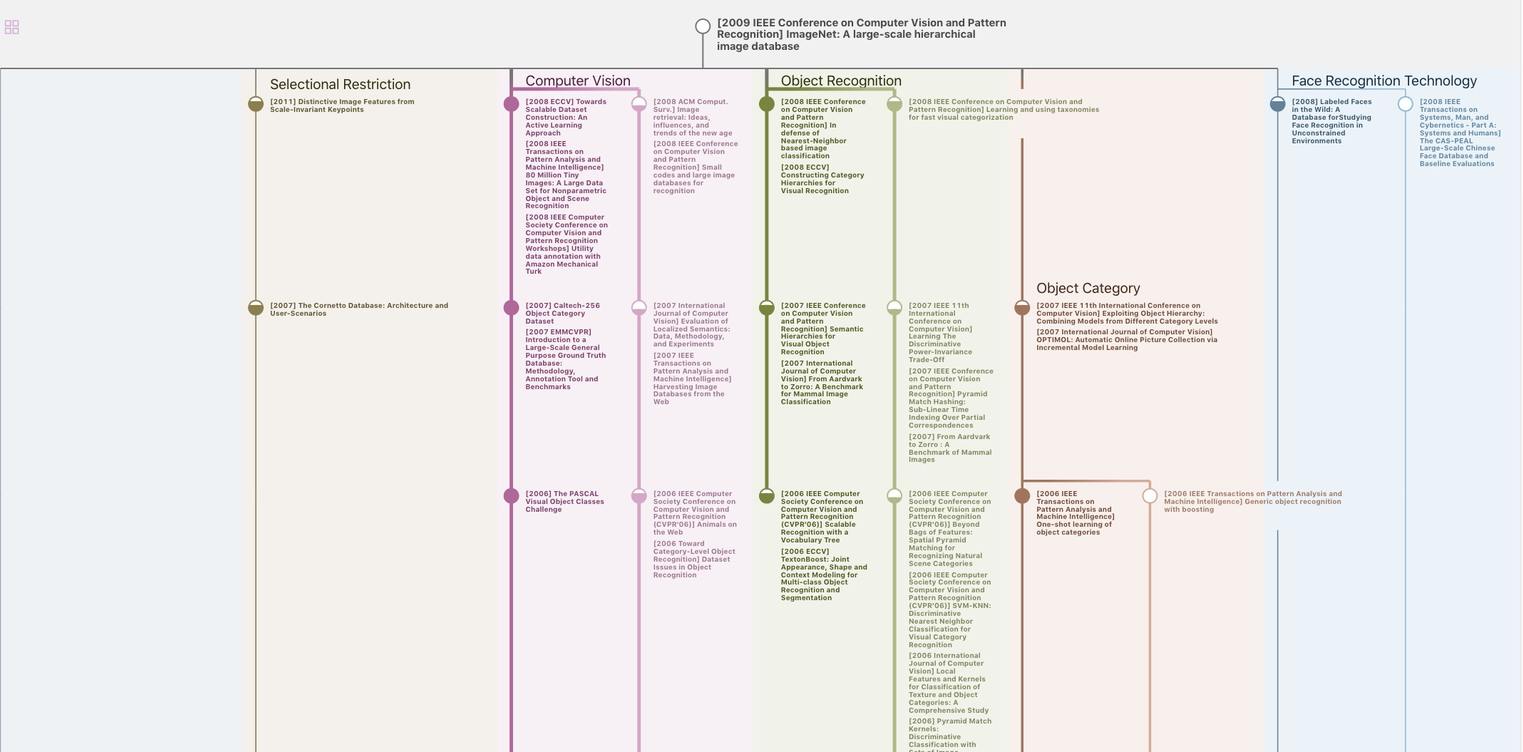
生成溯源树,研究论文发展脉络
Chat Paper
正在生成论文摘要